Multiple Output Sparse Gaussian Processes for Short-Term Electric Load Forecasting
2023 5th International Conference on Power and Energy Technology (ICPET)(2023)
摘要
Smart grid is introduced to address power grid challenges such as reliability, efficiency, greenhouse gas and carbon emissions, economics, safety, and energy security. Electric load forecasting is one of the techniques that support smart grid objectives. It forecasts the electric load at aggregated levels and is mandatory for the smart grid’s proper functioning. It keeps a balance between load generation and load consumption and can be classified as very short-term, short-term, medium-term, and long-term electric load forecasting based on the time interval of its prediction. In this paper, multiple output sparse Gaussian processes are proposed to predict 24 values of the electric load for the next day (short-term electric load forecasting) based on load and weather data (temperature and dew point) from previous days. The performance of the proposed multiple output sparse Gaussian processes was compared with the persistence method. By comparing the mean absolute percentage error values of the proposed method with the persistence method, it can be seen that the proposed method outperforms the persistence method very well.
更多查看译文
关键词
Electric load forecasting,Gaussian processes,machine learning,mean absolute percentage error,persistence method,smart grid,sparsity,statistical methods
AI 理解论文
溯源树
样例
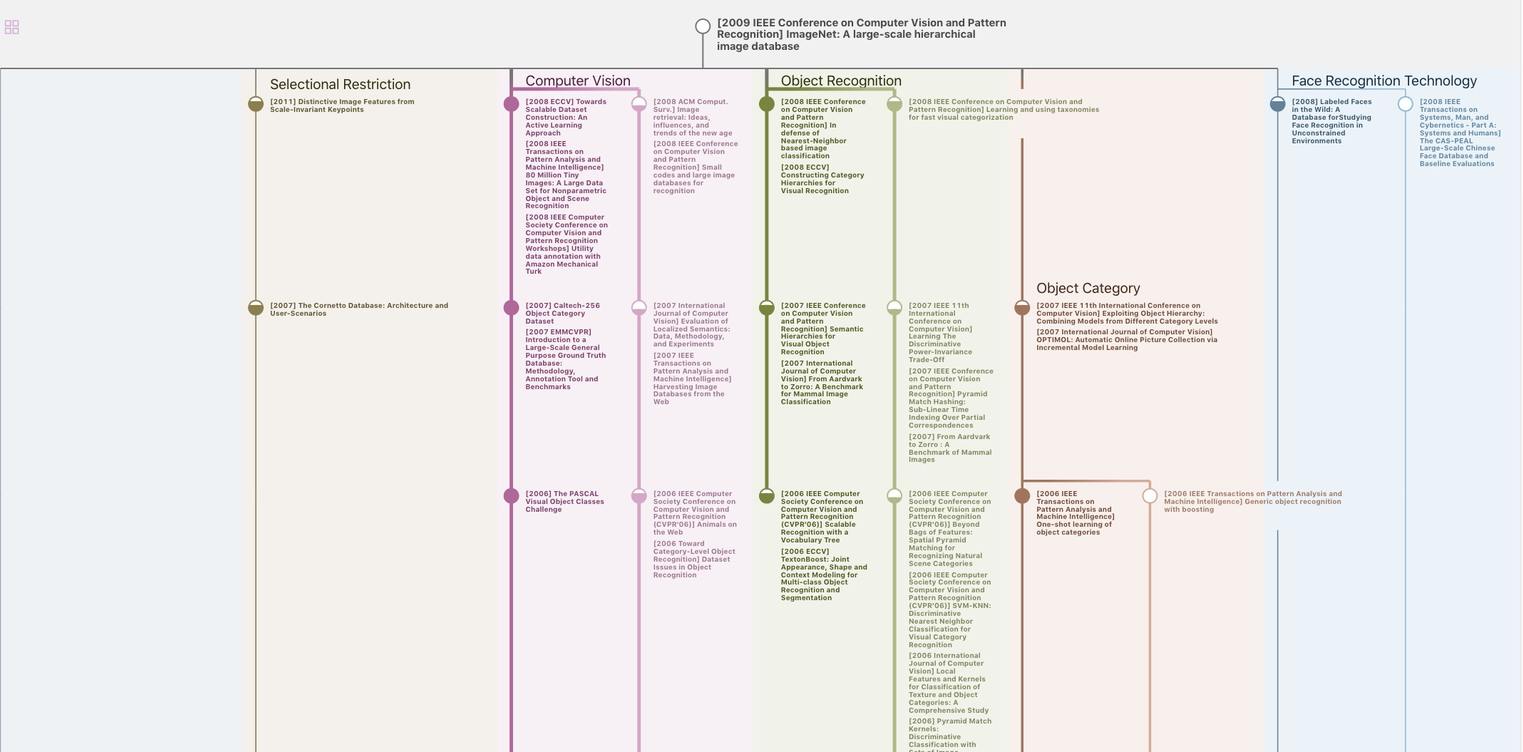
生成溯源树,研究论文发展脉络
Chat Paper
正在生成论文摘要