FPGA-based Degradation Evaluation for Traction Power Module with Deep Recurrent Autoencoder
2023 IEEE Energy Conversion Congress and Exposition (ECCE)(2023)
摘要
The timely and quantitative evaluation of the degradation is crucial for traction inverter systems in railway applications. The implementation in the industry is impeded by two major challenges including the varying operational profiles and the scalability for system-level applications. This paper proposes a deep recurrent autoencoder-based degradation evaluation method, to assess the degradation level of the traction power module online. The recurrent structure is embedded for processing multivariate time series condition monitoring data stream, in order to exploit the inherent time dependence to improve the accuracy and robustness. The autoencoder-based framework enables the scalability of the proposed method to system-level applications and can be applied under varying operating conditions. The method is experimentally demonstrated on an FPGA-based hardware platform.
更多查看译文
关键词
Power module,inverter system,deep learning,autoencoder,degradation,prognostics and health management
AI 理解论文
溯源树
样例
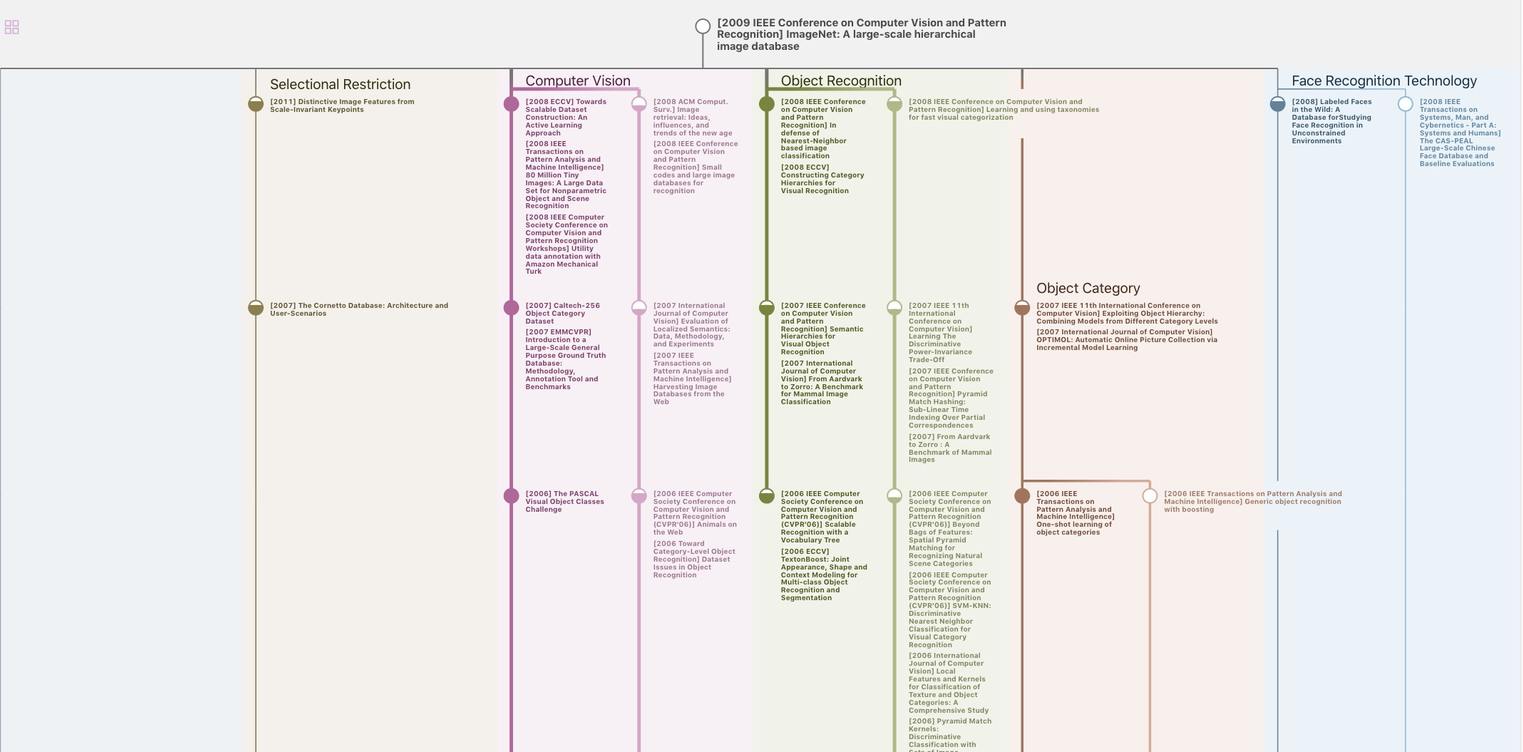
生成溯源树,研究论文发展脉络
Chat Paper
正在生成论文摘要