Quantum Annealing-Based Machine Learning for Battery Health Monitoring Robust to Adversarial Attacks
2023 IEEE Energy Conversion Congress and Exposition (ECCE)(2023)
摘要
Battery health monitoring methods including machine learning (ML) models rely on trustworthiness of battery sensor data and features. As more battery systems require network connectivity for intelligent health monitoring, cyberattacks become a serious threat. A notable attack is an adversarial attack that generates stealthier input data misleading the ML results. This paper introduces an adversarial attack targeting battery health monitoring system and explores a quantum annealing (QA)-based machine learning method to mitigate the adversarial attacks. In this paper, a Quantum Boost (QBoost)-based state of health (SOH) estimation algorithm for electric vehicles is developed and trained by D-Wave QA processor and the performance is compared with a state-of the art random forest (RF)-based SOH estimation method. The experimental results show that the proposed QBoost method is more robust to the adversarial attack than the RF method, while the RF method provides better accuracy during normal operation.
更多查看译文
关键词
Adversarial attack,battery health monitoring,quantum annealing processor,quantum boost,quantum machine learning
AI 理解论文
溯源树
样例
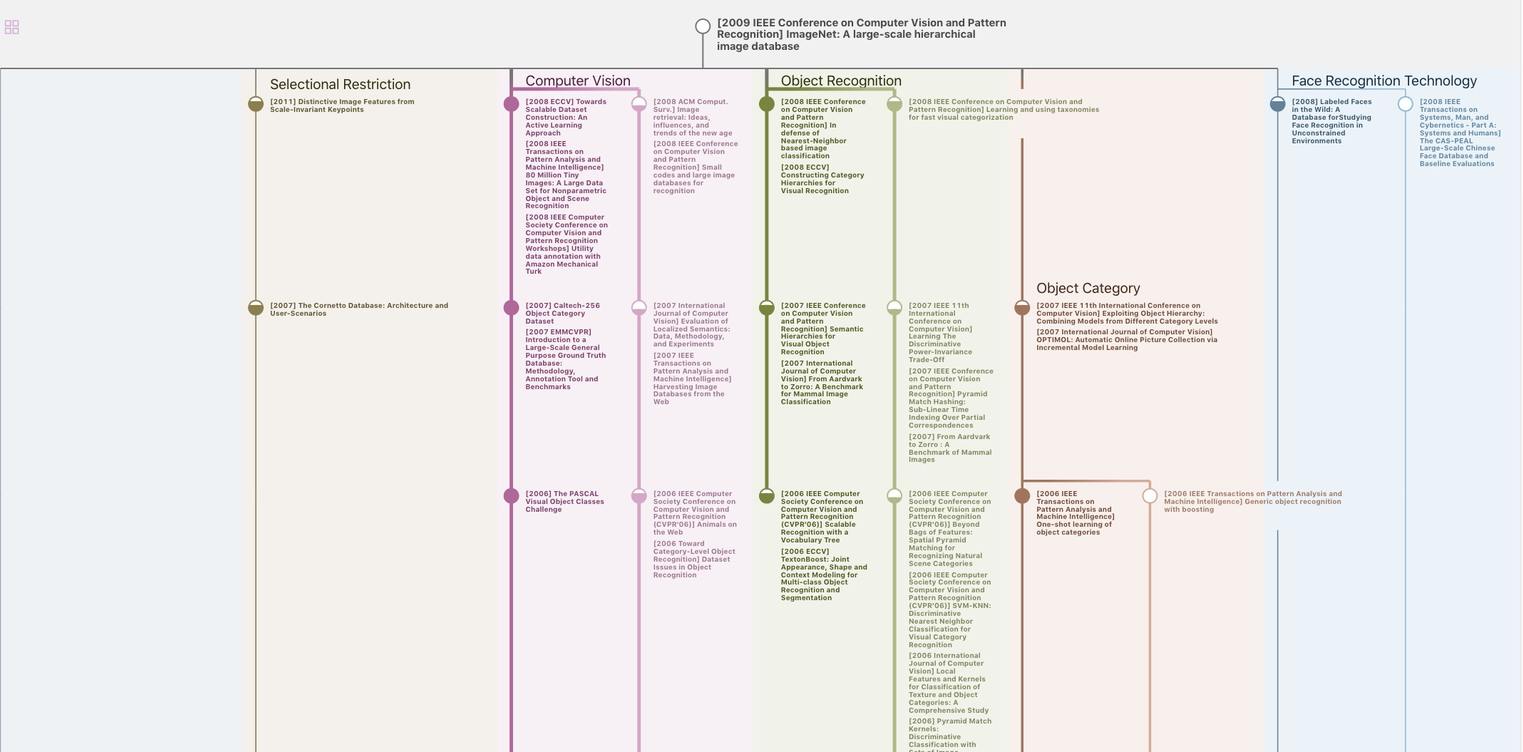
生成溯源树,研究论文发展脉络
Chat Paper
正在生成论文摘要