Enhancing Semi-Supervised Fetal Brain MRI Segmentation with Uncertainty-Guided Virtual Adversarial Training
2023 International Annual Conference on Complex Systems and Intelligent Science (CSIS-IAC)(2023)
摘要
The lateral ventricle is a key structure for diagnosis in fetal brain MRI scans, and its accurate segmentation is crucial. However, annotating medical images is time-consuming and complex. Semi-supervised learning has shown promise in a variety of medical imaging tasks. In an extension of Virtual Adversarial Training (VAT) [1], we introduce a novel method that amplifies the efficacy of adversarial perturbations by utilizing model uncertainty as a guiding mechanism during the perturbation process. Specifically, our method focuses perturbations in areas of high model uncertainty, thereby encouraging the model to yield consistent predictions for both the original and adversarially perturbed images. This adds regularization constraints to the data, steering the model's attention towards decision boundaries. We assessed the performance of our technique using a curated dataset of fetal brain MRI images, comparing it with six contemporary semi-supervised methods. Experimental results across three semi-supervised configurations substantiate the superiority of our approach in the context of fetal brain MRI image analysis.
更多查看译文
关键词
Virtual Adversarial Training,Uncertainty,Medical Images Segmentation
AI 理解论文
溯源树
样例
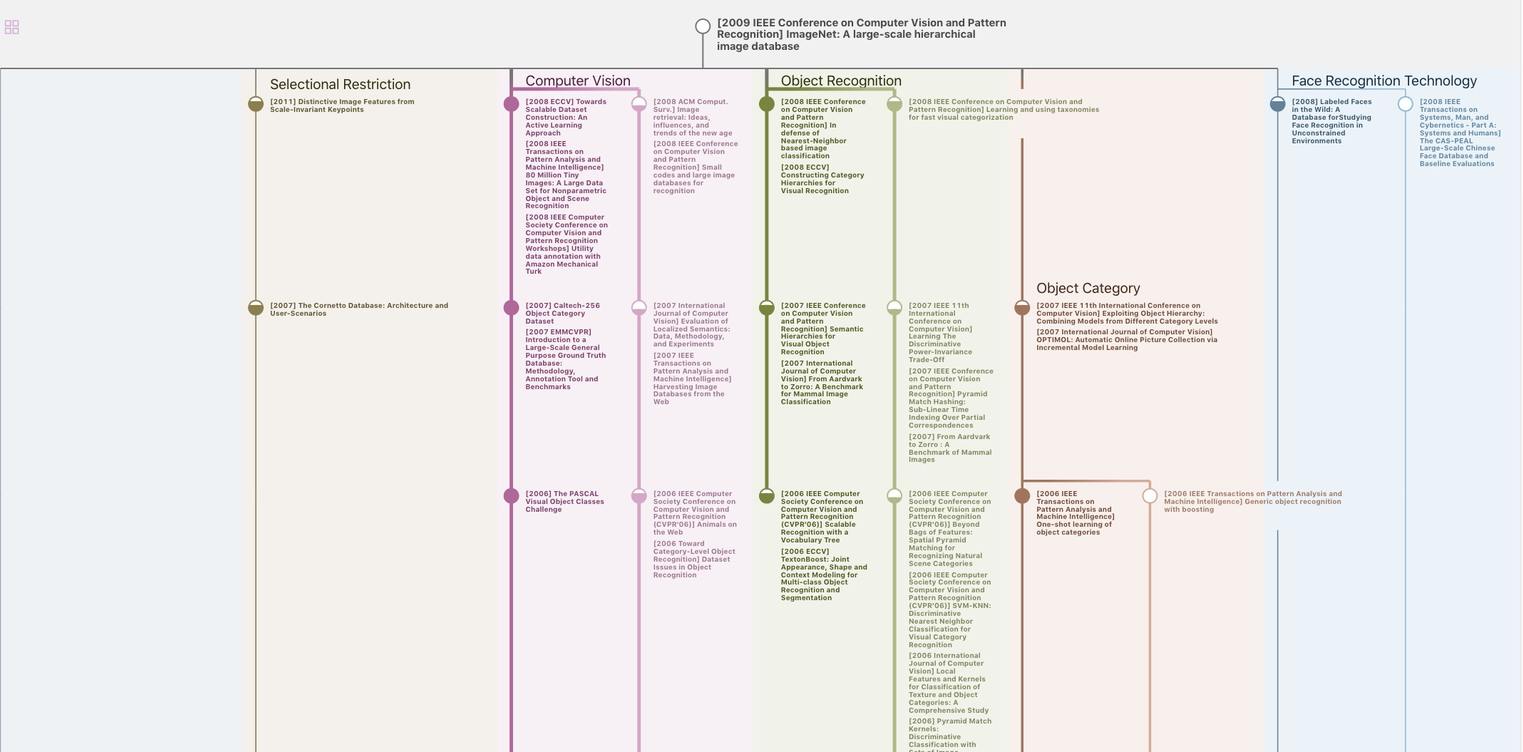
生成溯源树,研究论文发展脉络
Chat Paper
正在生成论文摘要