Hybrid Classification Model for Emotion Prediction from EEG Signals: A Comparative Study
JOURNAL OF UNIVERSAL COMPUTER SCIENCE(2023)
摘要
This paper introduces a novel hybrid algorithm for emotion classification based on electroencephalogram (EEG) signals. The proposed hybrid model consists of two layers: the first layer includes three parallel adaptive neuro-fuzzy inference systems (ANFIS), and the second layer called the adaptive network comprises various models such as radial basis function neural network (RBFNN), probabilistic neural network (PNN), and ANFIS. It is examined that the feature distribution graphs of the dataset, which includes three emotion classes: positive, negative, and neutral, and selected the most appropriate features for classification. The three parallel ANFIS structures were trained using the selected features as input vectors, and the outputs of these models were combined to obtain a new feature vector. This feature vector was then used as the input to the adaptive network, which produced the output of emotion prediction. In addition, it is evaluated the accuracy of the network trained using only the first features of the dataset. The hybrid structure was designed to enhance the system's performance, and the best accuracy result of 96.51% was achieved using the ANFIS-ANFIS model. Overall, this study provides a promising approach for emotion classification based on EEG signals.
更多查看译文
关键词
ANFIS,EEG,Emotion Classification,Hybrid Algorithm,Probabilistic Neural,Network,Radial Basis Function Neural Network
AI 理解论文
溯源树
样例
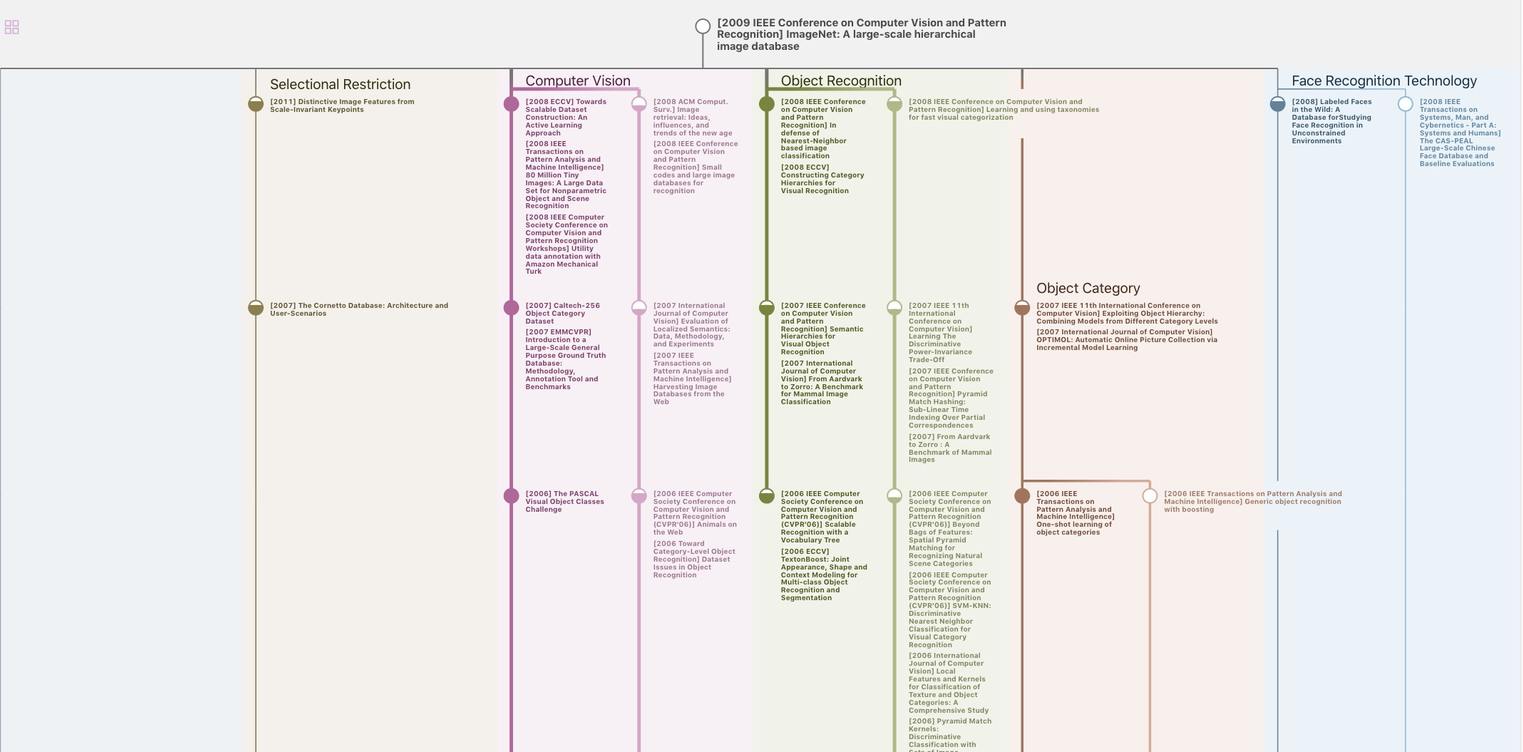
生成溯源树,研究论文发展脉络
Chat Paper
正在生成论文摘要