Hybrid Crowd-AI Learning for Human-Interpretable Symbolic Rules in Image Classification.
2023 14th IIAI International Congress on Advanced Applied Informatics (IIAI-AAI)(2023)
摘要
Explainable AI is an indispensable goal for an AI-based society with trust, and deriving human-interpretable symbolic rules is one of the promising ways to verify whether the decision is appropriate. This paper explores a hybrid crowd-AI approach to develop white-box ML models associated with human-interpretable symbolic rules. The key idea of the proposed method is to discover human-interpretable latent features from trained neural networks by leveraging human abductive reasoning. The proposed method automatically generates crowdsourcing tasks that display subsets of images corresponding to each latent feature and ask crowd workers to provide the semantics of the features in natural language. The obtained semantics allow us to use the latent features as human-interpretable predicates that form symbolic rules to define target classes. We provide experimental results showing that the proposed approach can obtain interpretable symbolic rules and explanations.
更多查看译文
关键词
XAI,Human-in-the-loop,Image Classification
AI 理解论文
溯源树
样例
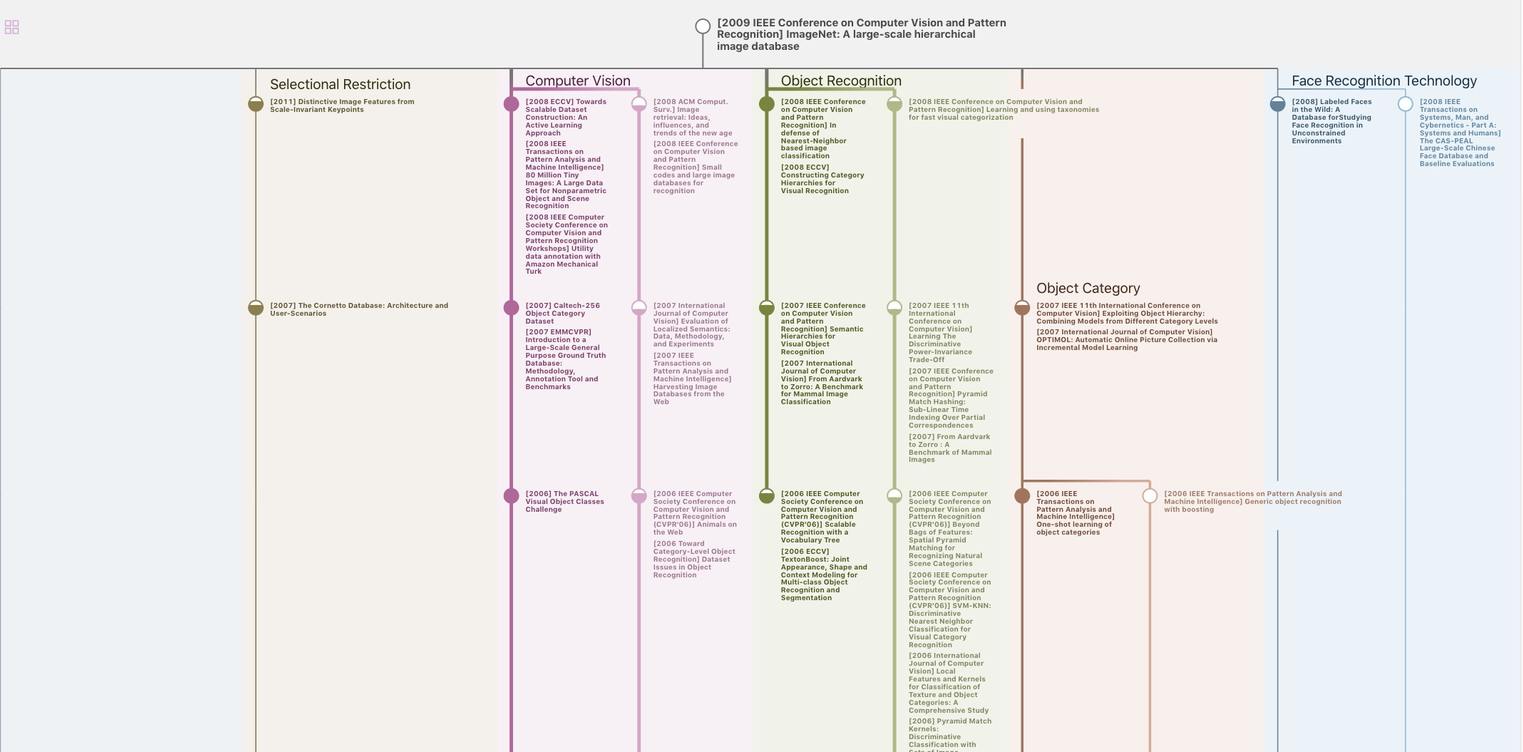
生成溯源树,研究论文发展脉络
Chat Paper
正在生成论文摘要