ADDet: An Efficient Multiscale Perceptual Enhancement Network for Aluminum Defect Detection
IEEE TRANSACTIONS ON INSTRUMENTATION AND MEASUREMENT(2024)
摘要
As an indispensable material, aluminum requires real-time surface defect detection to effectively control quality. However, defects on the surface of aluminum have the inherent properties of tiny size, diverse morphology, and multiscale variations, which present a significant challenge for defect detection. This article aims to improve the performance and efficiency of a defect detection model and proposes an efficient multiscale perceptual enhancement network for aluminum defect detection (ADDet). First, to compensate for the loss of deep features and mitigate information interference from the background, a multilayer semantic-aware enhancement module (MSEM) is proposed for the feature extraction network. Second, a proximity feature pyramid (PFP) structure in the neck is designed to effectively merge multiscale features with low computational cost and enhance feature representation across spatial coordinates, channels, and scales. Finally, a large kernel-size decoupled head (LKSDH) with a large receptive field and multiple detection branches is constructed in the detection head to improve detection accuracy. Experimental results show that the mean average precision (mAP) of the proposed ADDet is 80.85% with a real-time detection speed of 63.41 frames per second (FPS). Compared to YOLOv7, ADDet improves the mAP by 3.02%, and the number of parameters and FLOPs are reduced by 23.8% and 13%, respectively. Moreover, ADDet is shown to be superior to state-of-the-art detection methods in terms of detection accuracy.
更多查看译文
关键词
Feature extraction,Aluminum,YOLO,Surface morphology,Steel,Convolution,Surface treatment,Attention mechanism,multilevel features,multiscale,object detection,surface defect detection
AI 理解论文
溯源树
样例
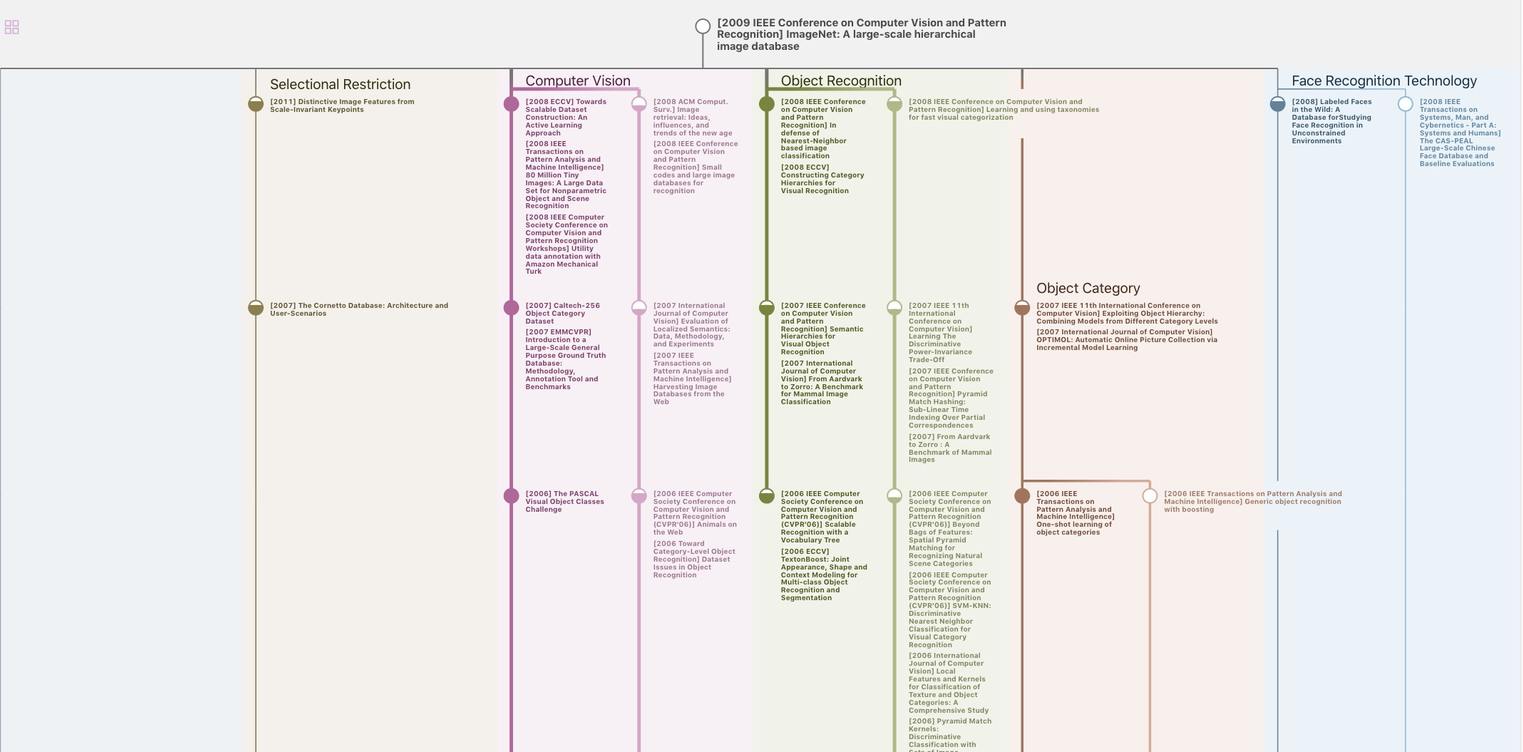
生成溯源树,研究论文发展脉络
Chat Paper
正在生成论文摘要