Disentangled Representations in Local-Global Contexts for Arabic Dialect Identification
IEEE-ACM TRANSACTIONS ON AUDIO SPEECH AND LANGUAGE PROCESSING(2024)
摘要
In this article, we propose a locally and globally informed disentanglement network for Arabic dialect identification (ADI). Our proposed disentanglement network aims to detach all irrelevant information (e.g., speaker, gender and channel) from the source utterance and extract only dialect-related representations fitted for the ADI problem. The proposed network consists of local convolutional backbone modules to learn low-resolution feature maps and self-attention-based bottleneck transformers to efficiently aggregate the local information to represent the global context as the learned dialect embeddings. We propose a novel supervised clustering loss to minimize intra-class variations and maximize inter-class variations in a latent space. Our model achieves state-of-the-art results in qualitative and quantitative evaluations by outperforming other competitive solutions on ADI-17 datasets. Specifically, we have shown that local-global awareness from our proposed network boosts feature representation and enhances identification performance.
更多查看译文
关键词
Arabic dialect identification,disentangled representation,supervised clustering,global context,transformer
AI 理解论文
溯源树
样例
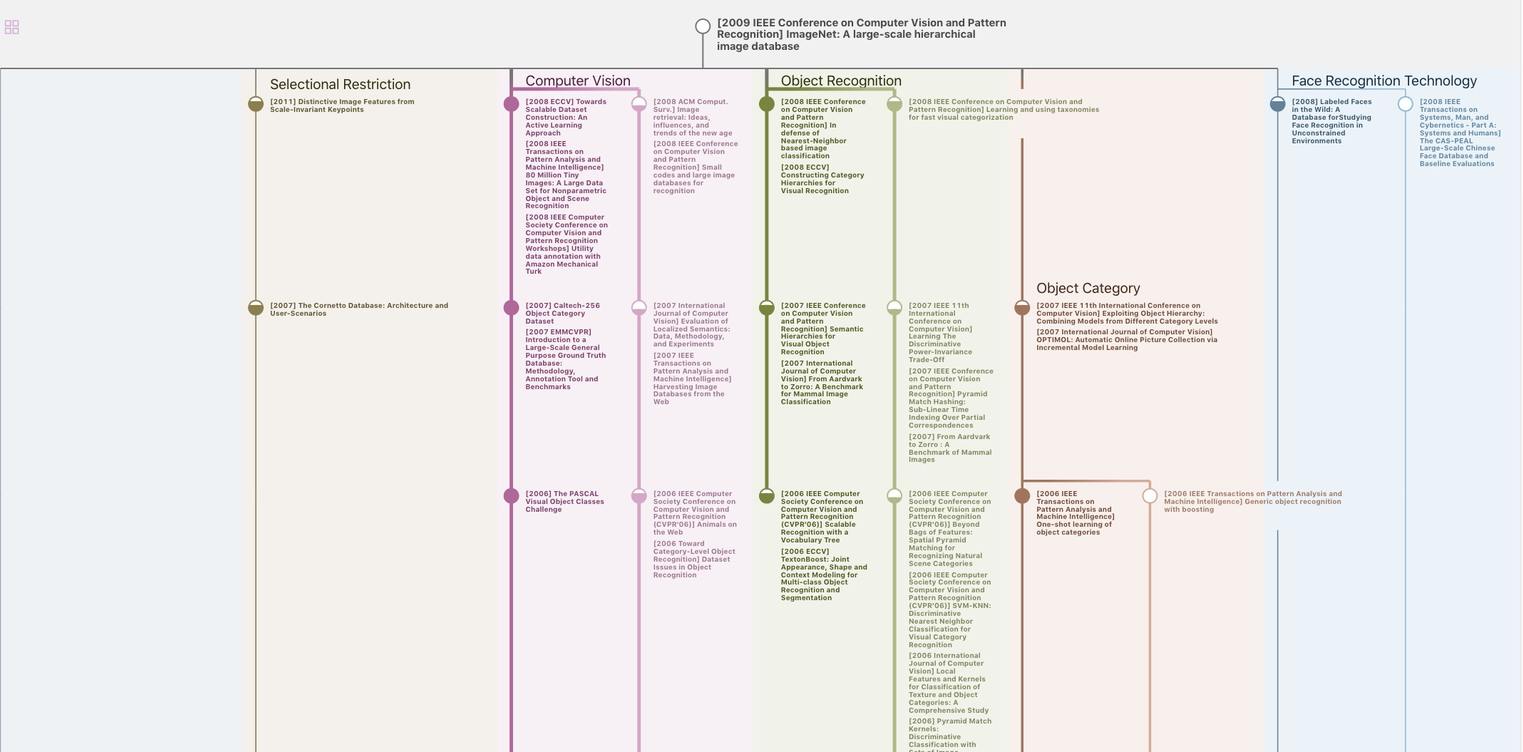
生成溯源树,研究论文发展脉络
Chat Paper
正在生成论文摘要