Segmentation-Guided Context Learning Using EO Object Labels for Stable SAR-to-EO Translation
IEEE GEOSCIENCE AND REMOTE SENSING LETTERS(2024)
摘要
Recently, the analysis and use of synthetic aperture radar (SAR) imagery have become crucial for surveillance, military operations, and environmental monitoring. A common challenge with SAR images is the presence of speckle noise, which can hinder their interpretability. To enhance the clarity of SAR images, this letter introduces a novel SAR-to-electro-optical (EO) image translation (SET) network, called SGCL-SET, which first incorporates EO object label information for stable translation. We use a pretrained segmentation network to provide the segmentation regions with their labels into learning the SET. Our SGCL-SET can be trained to effectively learn the translation for the regions of confusing contexts using the segmentation and label information. Through comprehensive experiments on our KOMPSAT dataset, our SGCL-SET significantly outperforms all the previous methods with large margins across nine image quality evaluation metrics.
更多查看译文
关键词
Image segmentation,Training,Radar polarimetry,Synthetic aperture radar,Generators,Electo-optic effects,Speckle,Generative adversarial network,SAR-to-EO translation,synthetic aperture radar (SAR) image
AI 理解论文
溯源树
样例
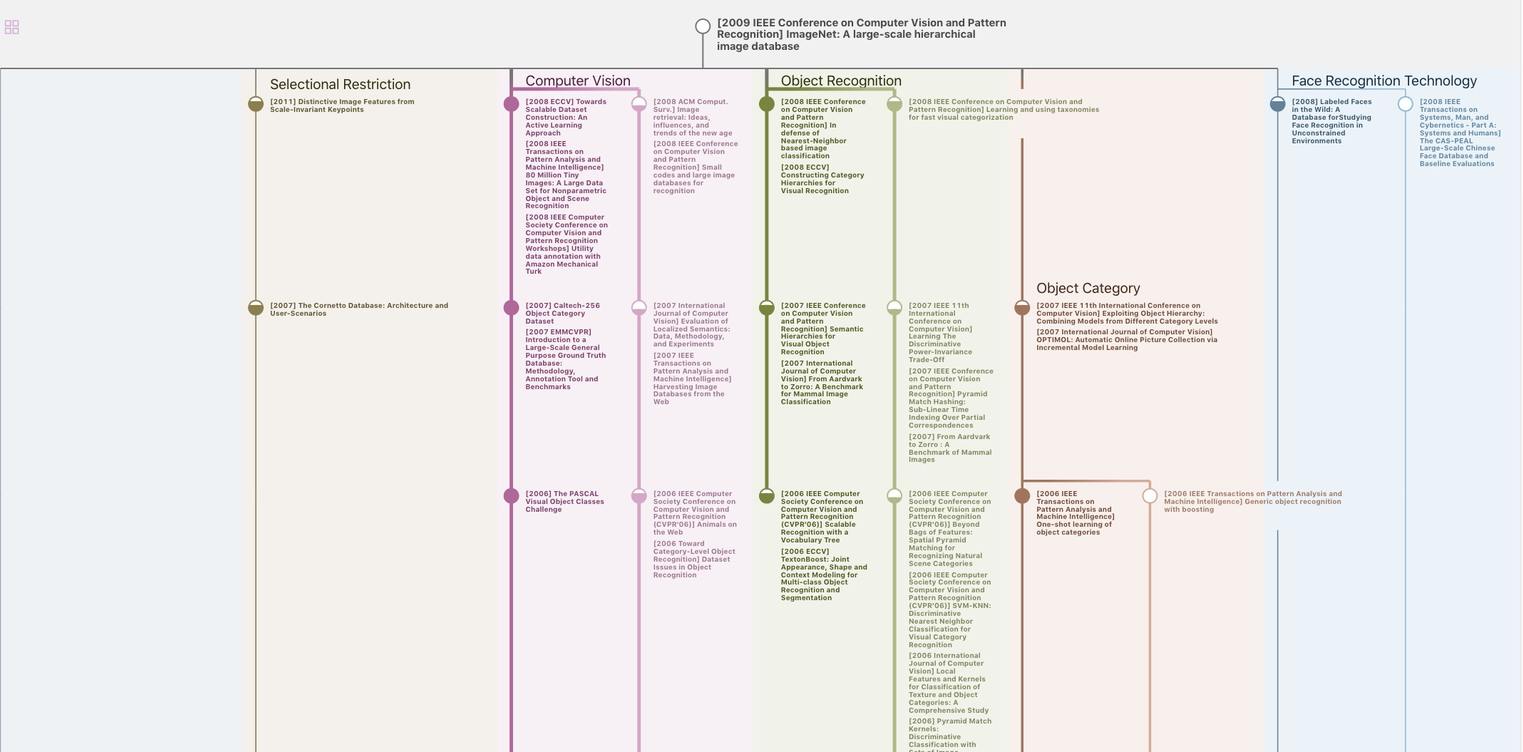
生成溯源树,研究论文发展脉络
Chat Paper
正在生成论文摘要