Nonintrusive Load Identification Considering Unknown Load Based on Bimodal Fusion and One-Class Classification
IEEE TRANSACTIONS ON INSTRUMENTATION AND MEASUREMENT(2024)
摘要
Nonintrusive load monitoring (NILM) enables the real-time monitoring and data analysis of energy consumption, providing more accurate, efficient, and intelligent data support for energy management. However, traditional NILM models can only identify known load (KL) presented in the training dataset; they cannot perform unknown load (UKL) detection, and they have problems with feature selection, including information loss and limitations in capturing load signal information, which make them less suitable for the user side. In this article, a new method based on bimodal fusion and one-class (OC) classification is proposed for NILM. The bimodal features include 2-D image features and multidimensional sequence features. To enrich the feature representation, the bimodal features are fused by long short-term memory (LSTM) network. To address UKL detection, a simple and lightweight OC model based on deep support vector data description (DSVDD) is innovatively proposed. The proposed model is also validated on multiple publicly available datasets, demonstrating its significant advantages and applicability both on KL identification and UKL detection.
更多查看译文
关键词
Feature extraction,Load modeling,Trajectory,Training,Data models,Computational modeling,Steady-state,Bimodal feature fusion (BMFF),deep support vector data description (DSVDD),long short-term memory (LSTM),nonintrusive load monitoring (NILM),unknown load (UKL) detection
AI 理解论文
溯源树
样例
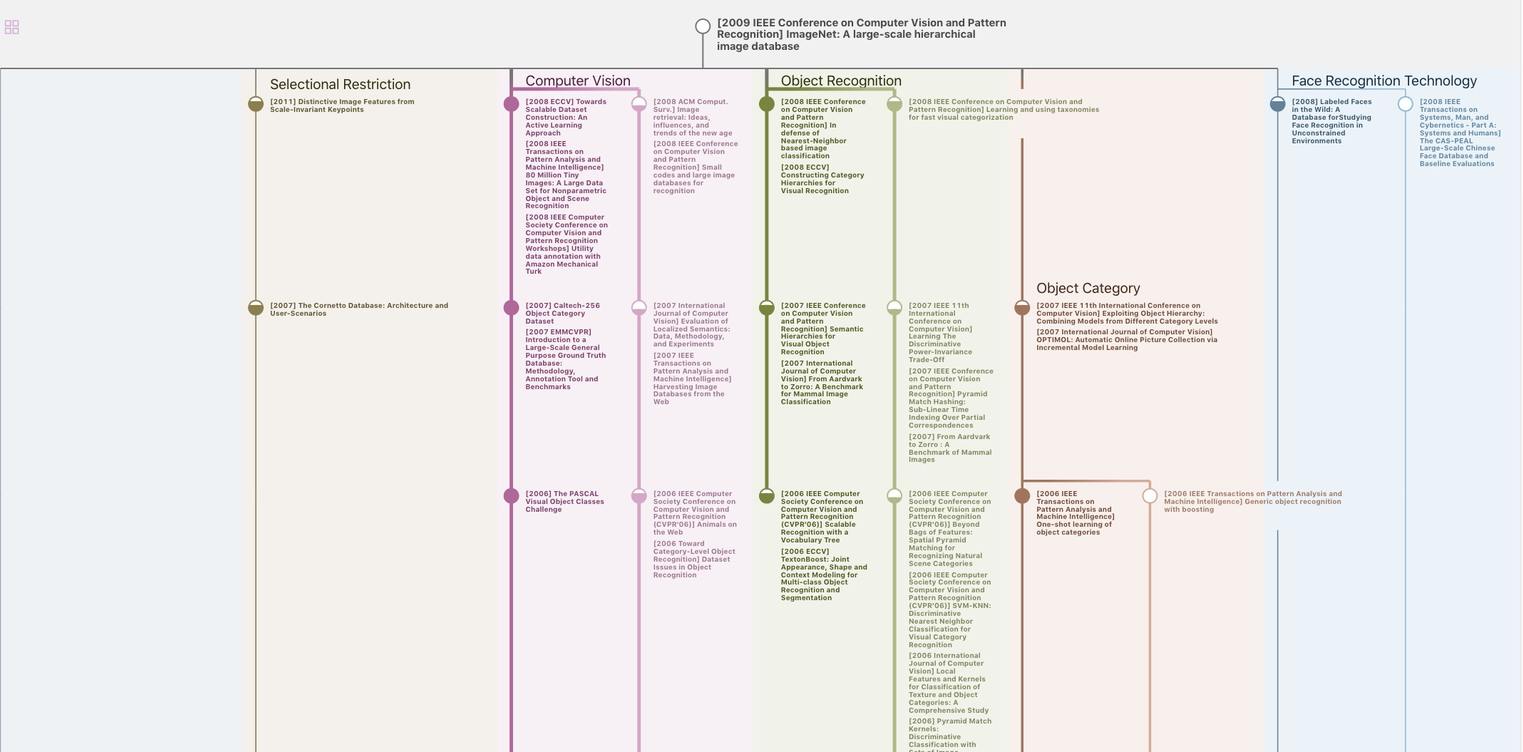
生成溯源树,研究论文发展脉络
Chat Paper
正在生成论文摘要