A General Purpose Anomaly-Based Method for Detecting Cheaters in Online Courses.
IEEE Transactions on Learning Technologies(2024)
摘要
This article presents a general-purpose method for detecting cheating in online courses, which combines anomaly detection and supervised machine learning. Using features that are rooted in psychometrics and learning analytics literature, and capture anomalies in learner behavior and response patterns, we demonstrate that a classifier that is trained on labeled data of users who performed a certain type of cheating (copying using multiple accounts) can detect users who performed a different type of cheating (unauthorized collaboration), as well as to detect cheaters in different contexts—future runs of the same course and another course that is on a different subject matter. This exploits the fact that we have dedicated algorithms for detecting these two methods of cheating, which enable us to compute ground truth labels on real student interaction data and, then, use these labeled data to validate the proposed general-purpose algorithm. The empirical setting is based on five runs of two MITx massive open online courses in physics and biology from 2014 to 2016. The main contribution of this article is twofold: first is proposing, for the first time, a general method for detecting cheating in online courses; and second is providing a validation that is based on real cheating data, as opposed to previous research on cheating detection, that with the absence of ground truth, relied on either simulations or indirect measures.
更多查看译文
关键词
Anomaly detection,cheating,learning analytics,massive open online courses (MOOCs)
AI 理解论文
溯源树
样例
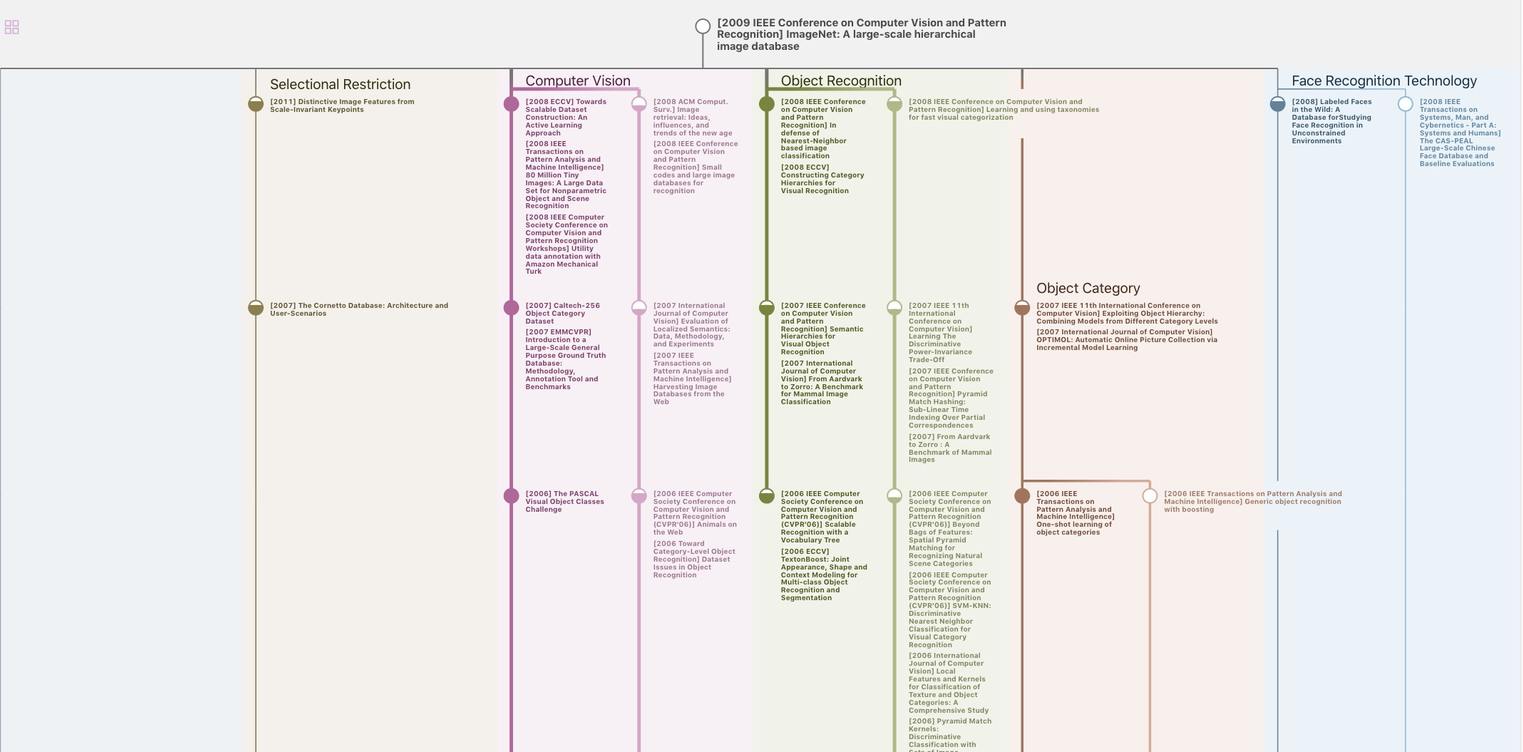
生成溯源树,研究论文发展脉络
Chat Paper
正在生成论文摘要