Clipping Noise Mitigation for Coherent OFDM Systems Using Decision-Aided Reconstruction Combined with Neural Networks.
2023 33rd International Telecommunication Networks and Applications Conference(2023)
摘要
Clipping noise constitutes a prominent challenge within transmission systems employing orthogonal frequency division multiplexing (OFDM) due to its high peak-to-average power ratio (PAPR). This paper introduces a novel algorithm for mitigating clipping noise at the receiver end of a coherent transmission system. The approach utilizes a recurrent neural network (RNN), specifically based on long short-term memory (LSTM) cells, to reduce the initial symbol error probability by reconstructing the clipped signal in time domain. Subsequently, an adapted iterative decision-aided reconstruction (DAR) algorithm is proposed. This algorithm incorporates two major improvements: Oversampling in time domain, which allows the filtering of residual out-of-band clipping noise after the nonlinear combination, and a novel method for phase management during reconstruction within a coherent system. Numerical simulations show the superior performance of the neural network in comparison to model-based strategies in terms of reducing the initial symbol error probability. Moreover, the application of oversampling and filtering achieves an additional performance gain. Finally, the symbol error ratio can be further reduced by exclusively combining magnitudes during a nonlinear combination process while retaining the phase of the received signal. The interaction of the neural network with the proposed algorithm is able to reduce the symbol error probability by up to five orders of magnitude, solely by processing the identical received signal.
更多查看译文
关键词
OFDM,PAPR,clipping noise,mitigation,neural network,DAR
AI 理解论文
溯源树
样例
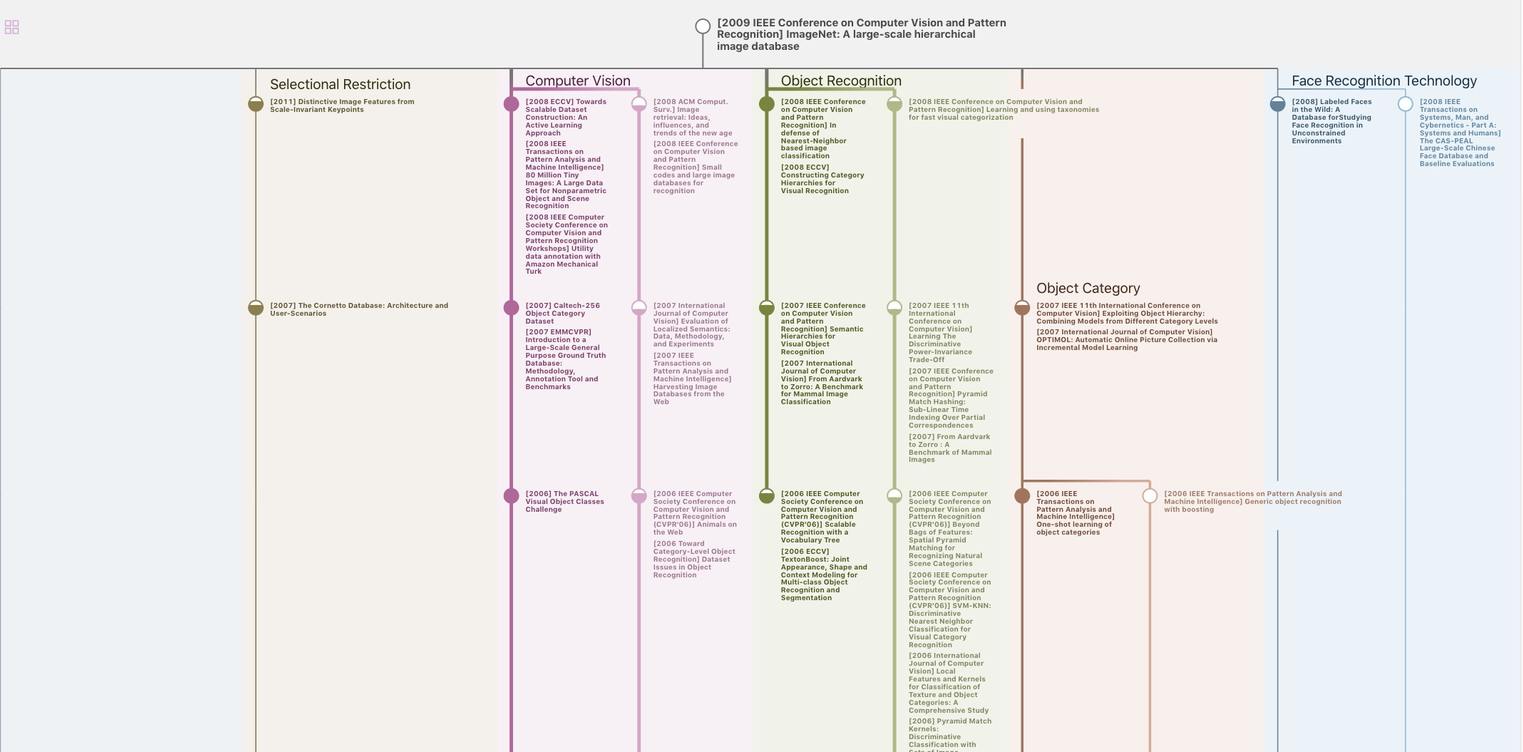
生成溯源树,研究论文发展脉络
Chat Paper
正在生成论文摘要