CaliProb: Probability-based Calibration Model for Robust Predictions in Environments with Data Biases.
2023 33rd International Telecommunication Networks and Applications Conference(2023)
摘要
Various studies have focused on solving data bias problems in artificial intelligence. However, conventional approaches have primarily focused on data biases originating from imbalanced data distributions between sensor nodes and algorithms owing to overfitting. However, methods handling the biases between sensors have rarely been considered. In general, when data are trained using multiple heterogeneous devices, a device bias occurs due to process, voltage, and temperature variations, differences in the preprocessing methods for each device and the aging of specific devices, resulting in a data bias. In this study, we prove that a data bias can occur due to a device bias, which deteriorates the training performance. In response, we propose the probability-based calibration (CaliProb) model. This model compares the predicted probabilities for each piece of data, selects the data with the best training performance as the reference data, and calibrates the data bias using these reference data. We experimentally verify the CaliProb model. It exhibits an accuracy greater than that of the uncalibrated general model in situations with low and high data biases and achieves an accuracy that is approximately 12.2% higher than that of the Non-calibrated model in a case with abundant data bias.
更多查看译文
关键词
Device bias,Data bias,Machine learning,Data calibration
AI 理解论文
溯源树
样例
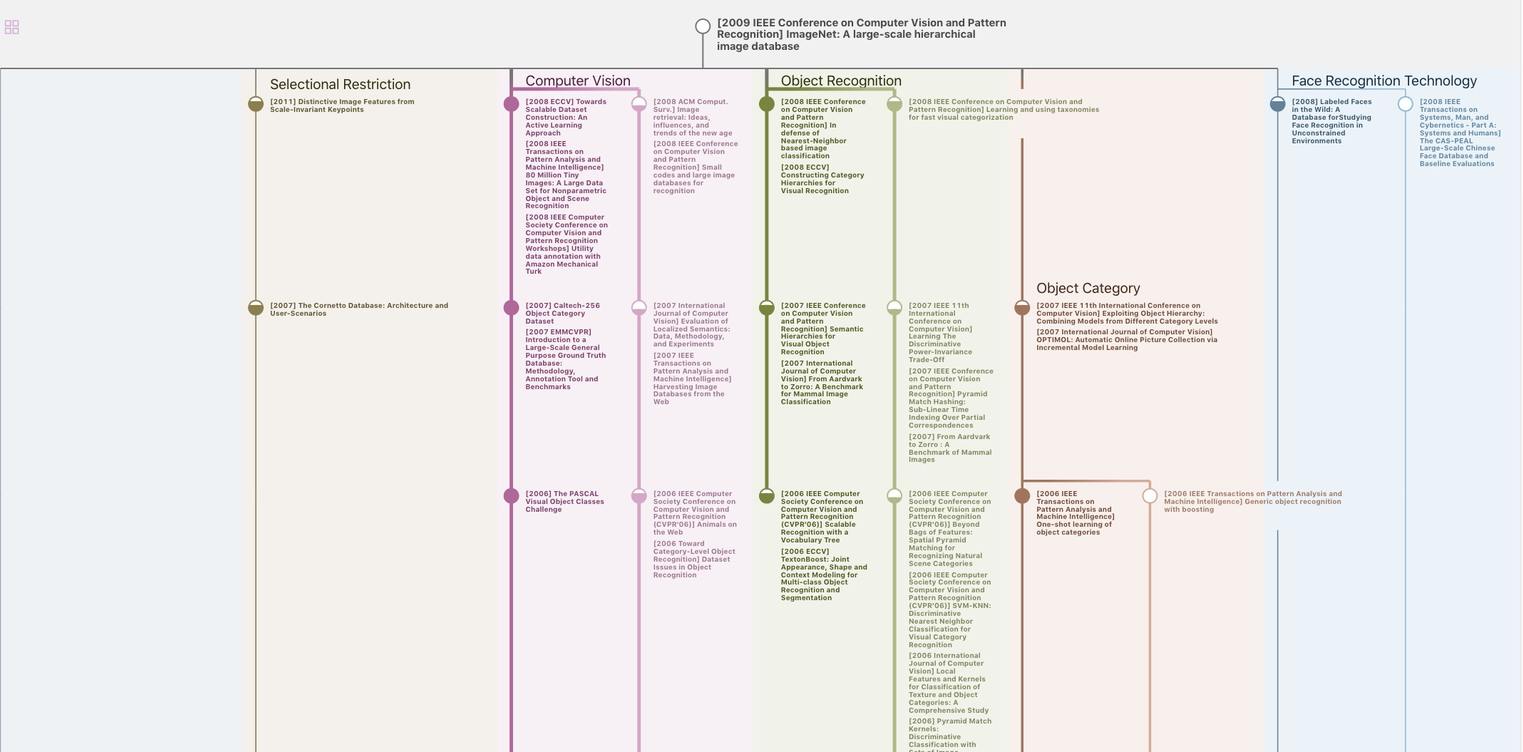
生成溯源树,研究论文发展脉络
Chat Paper
正在生成论文摘要