NeuralPUMA: Learning to Phase Unwrap Through Differentiable Graph Cuts
IEEE TRANSACTIONS ON SIGNAL PROCESSING(2024)
摘要
Deep learning solutions have recently demonstrated remarkable performance in phase unwrapping by approaching the problem as a semantic segmentation task. However, these solutions lack explainability and robustness to unseen conditions, and they often need a large amount of data for training. By contrast, traditional phase unwrapping algorithms, such as PUMA, rely on principled pipelines that estimate the phase through optimization solvers, despite often failing under severe noise conditions. In this work, we show how to exploit the benefits of both approaches by proposing a way to combine deep neural networks with iterative energy minimization algorithms based on graph cuts. We implement a differentiable version of the PUMA algorithm, NeuralPUMA, which we integrate into a traditional deep learning pipeline to implicitly learn to preprocess the wrapped phase into an intermediate representation that improves the algorithm solution. Through extensive experiments, we show that our approach effectively improves the performance of PUMA in noisy conditions and outperforms recent deep learning methods, while also requiring less training data and simpler neural architectures.
更多查看译文
关键词
Phase unwrapping,deep learning,energy minimization,graph cuts,submodularity,implicit differentiation.
AI 理解论文
溯源树
样例
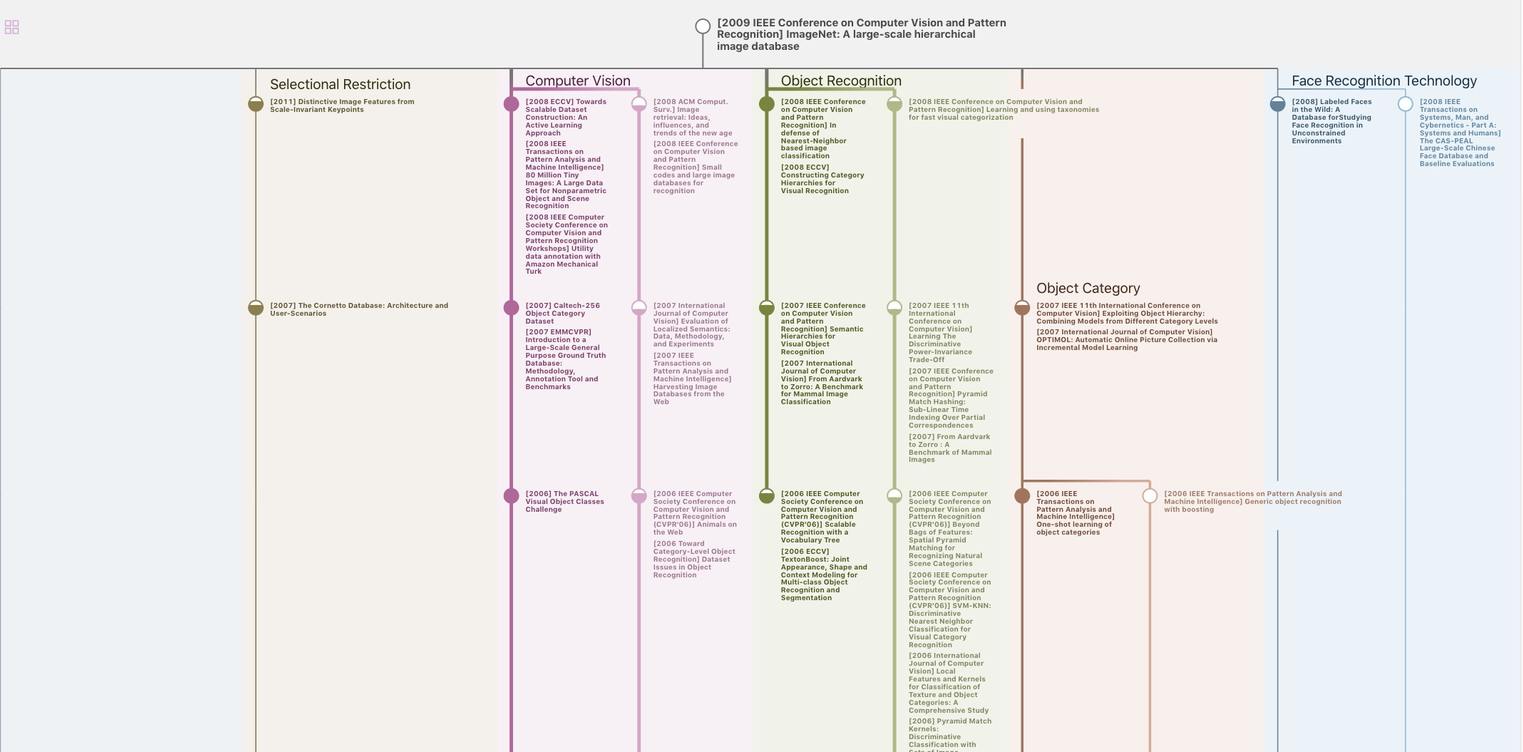
生成溯源树,研究论文发展脉络
Chat Paper
正在生成论文摘要