Imbalanced data augmentation for pipeline fault diagnosis: A multi-generator switching adversarial network
CONTROL ENGINEERING PRACTICE(2024)
摘要
Pipeline fault diagnosis is of practical necessity for the stable operation of large-scale oil and gas transportation systems. Despite the remarkable achievements of existing intelligent fault diagnosis methods, these studies have encountered noticeable performance degradation when directly applied in real industrial scenarios due to the absence of sufficient and balanced datasets. To this end, this paper proposes a novel multi-generator switching adversarial network (MGSAN) to augment the pipeline data under different health states, especially faulty ones. First, a multi-mode generator is designed to produce various candidate solutions, where a parameter-sharing scheme is employed to ensure consistency among modes at the parameter level. After that, a mode switching strategy is presented to maintain a proper tradeoff between the generator and the discriminator, which is capable of changing the state of the generator from one mode to another according to the performance (i.e., quality and diversity) of the candidate solutions. Finally, extensive experimental results on visualization and quantitative evaluation show that the MGSAN algorithm has more stable behavior and stronger exploration capabilities than well-known GAN variants. Additionally, the proposed MGSAN algorithm is successfully exploited to improve the accuracy of pipeline fault diagnosis.
更多查看译文
关键词
Multi-generator switching adversarial network,Data augmentation,Pipeline fault diagnosis,Mode switching strategy,Imbalanced data,Parameter-sharing scheme
AI 理解论文
溯源树
样例
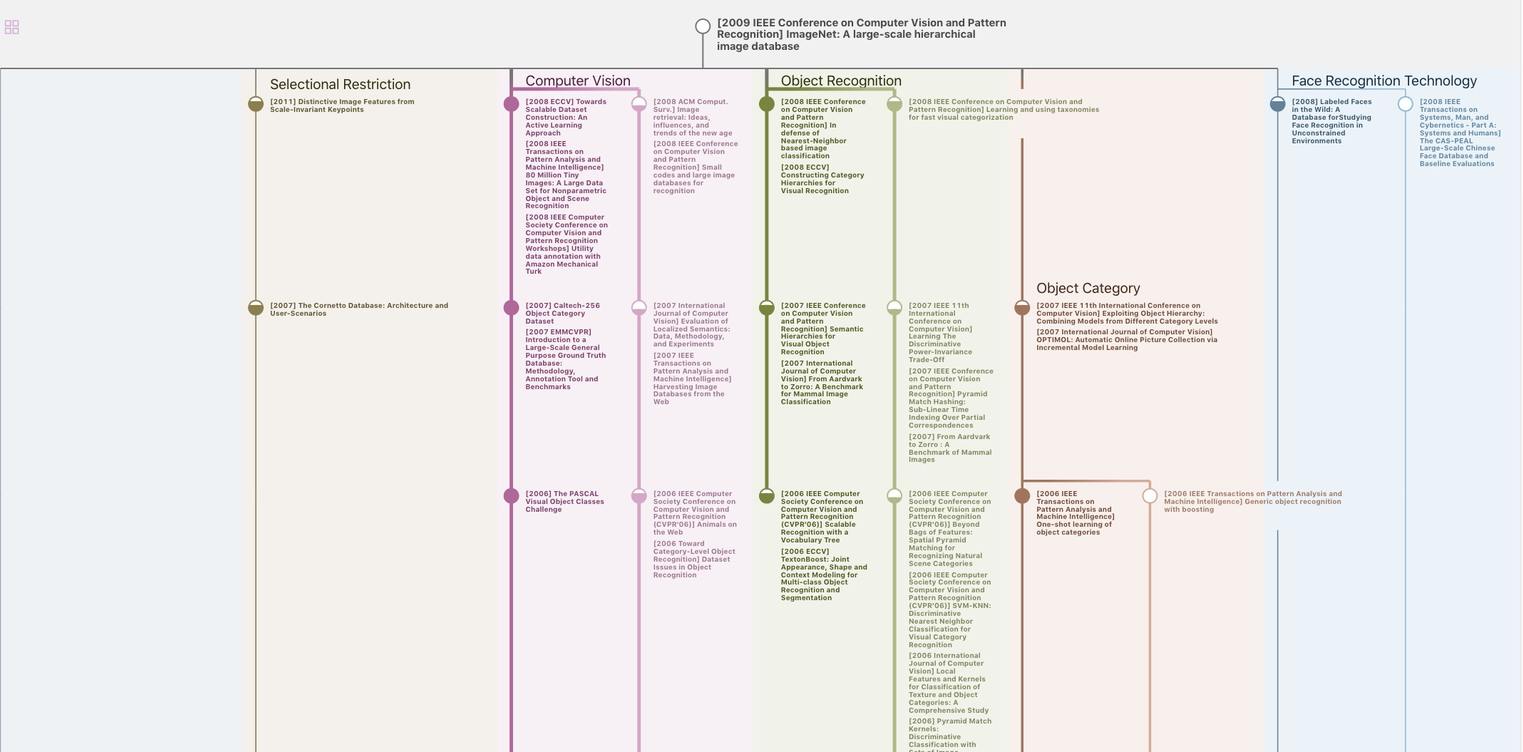
生成溯源树,研究论文发展脉络
Chat Paper
正在生成论文摘要