Evolution-based energy-efficient data collection system for UAV-supported IoT: Differential evolution with population size optimization mechanism
EXPERT SYSTEMS WITH APPLICATIONS(2024)
摘要
In recent years, unmanned aerial vehicles (UAVs) have been broadly employed as a data collection platform to assist in efficiently collecting data from IoT devices. However, the deployment optimization of UAVs has been challenged due to the need to minimize the energy consumption of UAVs and IoT devices. Several algorithms have been recently proposed for tackling this challenge, but they still have room for improvement due to their slow convergence speed and memory-wasting problems. Therefore, in this study, a new energy-aware approach has been proposed for accurately optimizing the entire deployment of UAVs, which could minimize the total energy consumption. This approach is based on presenting a new encoding mechanism, namely an optimized population size mechanism, for representing both location and number of stop points in an effective manner. In this mechanism, similar to some studies in the literature, the whole population is responsible for the entire deployment, and each individual is responsible for a stop point in this deployment. However, this mechanism presents a novel way to optimize the number of stop points based on adding an auxiliary variable to each stop point to determine whether it will be removed, inserted, or replaced in the newly generated deployment. This variable will be optimized by the optimization techniques during the optimization process to search for the optimal choice for each stop point that could achieve a better deployment. Two well-known optimization techniques, known as differential evolution (DE) and gradient-based optimizer (GBO), are adapted using this mechanism to present new variants, namely DEoPS and GBoPS, for accurately tackling the deployment optimization problem. Two energy consumption formulations are used in our work to investigate the performance of DEoPS and GBoPS. Several experiments have been conducted to compare the performance of both DEoPS and GBoPS with several algorithms on eleven instances. The experimental findings show the effectiveness of GBoPS for the first formulation and the effectiveness of DEoPS for the second formulation.
更多查看译文
关键词
UAV,Internet of Things,Differential evolution,Gradient-based optimizer,Deployment optimization,Encoding,Optimized population size
AI 理解论文
溯源树
样例
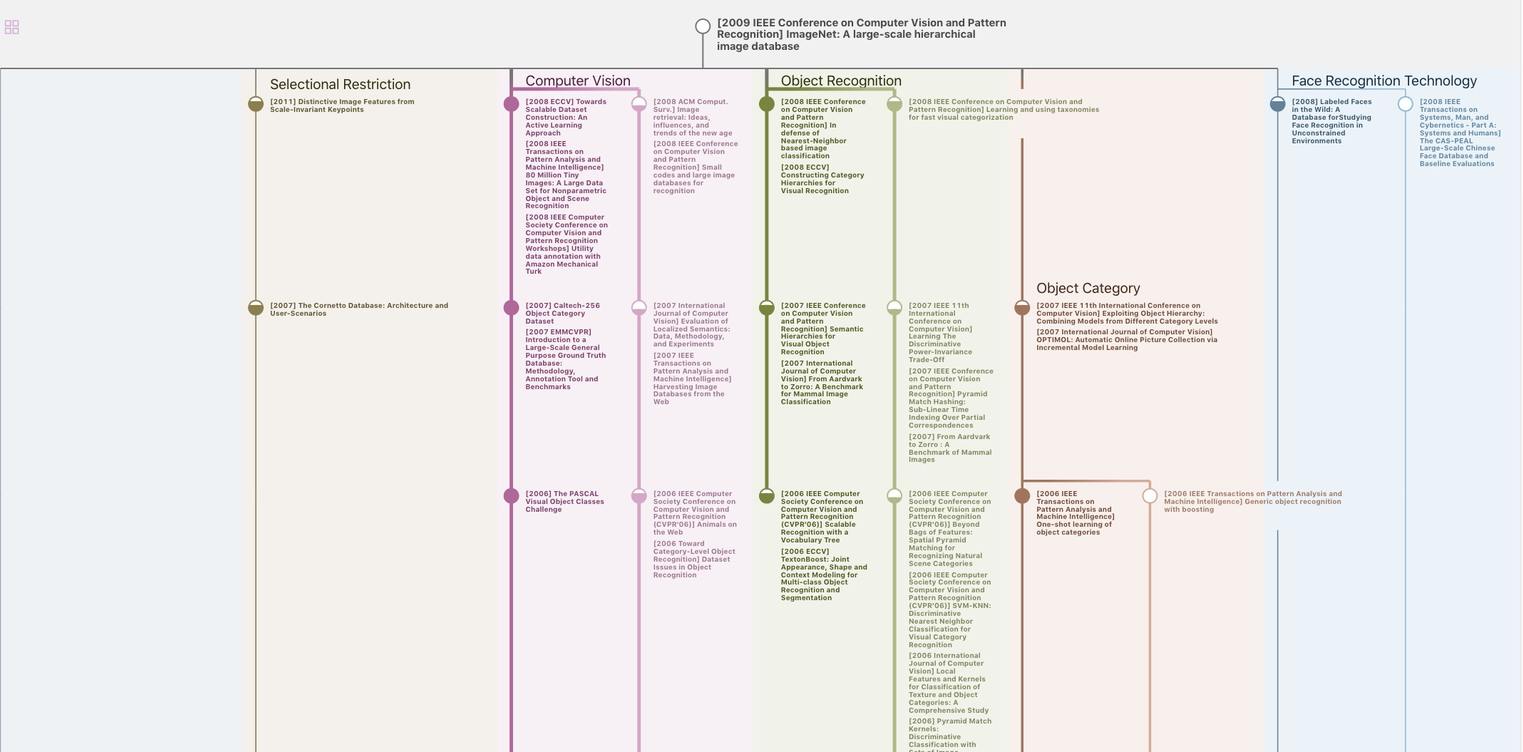
生成溯源树,研究论文发展脉络
Chat Paper
正在生成论文摘要