On-the-Fly Learning With Mixed-Mode Spiking Neural Network and Passive Memristive Array: Application to Neuromorphic Cameras
IEEE ACCESS(2023)
摘要
The massive deployment of the Internet of Things (IoT) combined with the need to reduce its impact on global energy consumption calls for the design of intelligent sensors. These sensors must be able to process information in situ to reduce the amount of data to be transferred, and to perform computing at low energy cost. Therefore, the design of intelligent sensors requires a compromise between performance and energy consumption. Spiking neural networks (SNNs) are good candidates for achieving the goal of an efficient intelligent sensor, as they use event-based computation. Interest for hardware implementation of SNNs has bloomed since the first experimental observation of memristors in 2008. In this paper, we study, by simulation means, the impact of the main technological parameters of Ferroelectric Tunnel Junctions (FTJs) synapses and of analog Leaky Integrate-and-Fire (LIF) neurons on the system learning capabilities. This allows us to determine which parameters are critical for the design of such systems and to suggest mitigation solutions as well as guidelines on how to build a SNN-based smart vision sensor in the context of unsupervised or reward-modulated learning. In particular, we show that splitting up the passive crossbar array of memristors could help dealing with the detrimental effect of the input voltage offset of the postsynaptic neurons.
更多查看译文
关键词
Edge computing,memristors,passive crossbar,reward-modulated learning,spiking neural networks,unsupervised learning
AI 理解论文
溯源树
样例
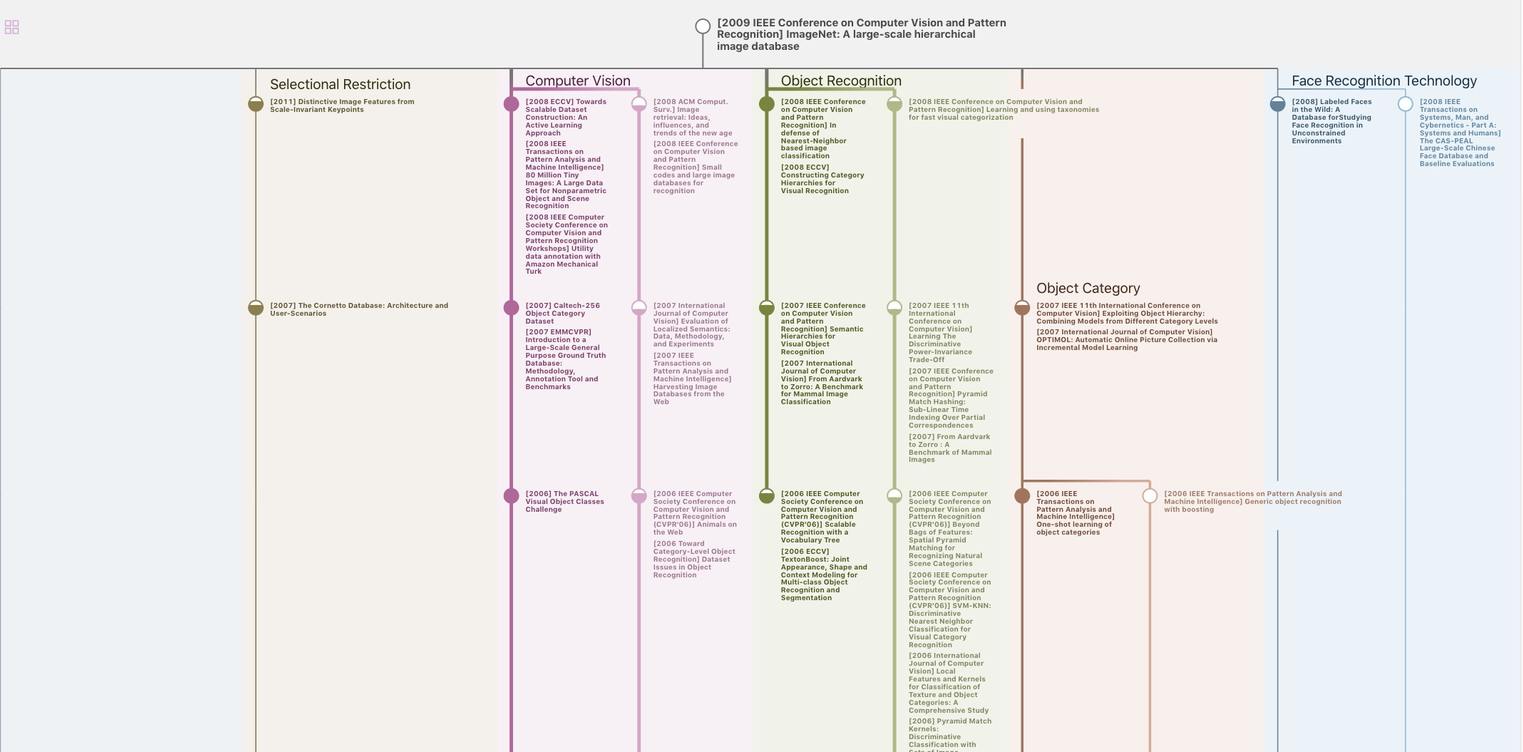
生成溯源树,研究论文发展脉络
Chat Paper
正在生成论文摘要