Token labeling-guided multi-scale medical image classification
PATTERN RECOGNITION LETTERS(2024)
摘要
Vision transformer has been widely used in medical image analysis. However, in most of current methods, only the class token is concerned during training, while the output patch tokens' information is not well utilized. To track this problem, we propose a two-stage token labeling guided multi-scale model for medical image classification. In the first stage, we pre-train a classification model to extract critical areas as token labeling. In the second stage, we adopt coarse and fine branches to encode visual features, which adapts to the various lesions in medical images. Then, the class token output by each branch is fused for classification. The token labeling is used to supervise the representation learning of patch tokens, which can integrate the local information into the learning. The experimental results on Laryngoscope8, ISIC 2018, and REFUGE data sets show that after adding token labeling, this dual-branch classification model achieves significantly better performance than the model using only class token loss, which demonstrates the effectiveness of our method for medical image classification tasks.
更多查看译文
关键词
Medical image classification,Vision transformer,Token labeling
AI 理解论文
溯源树
样例
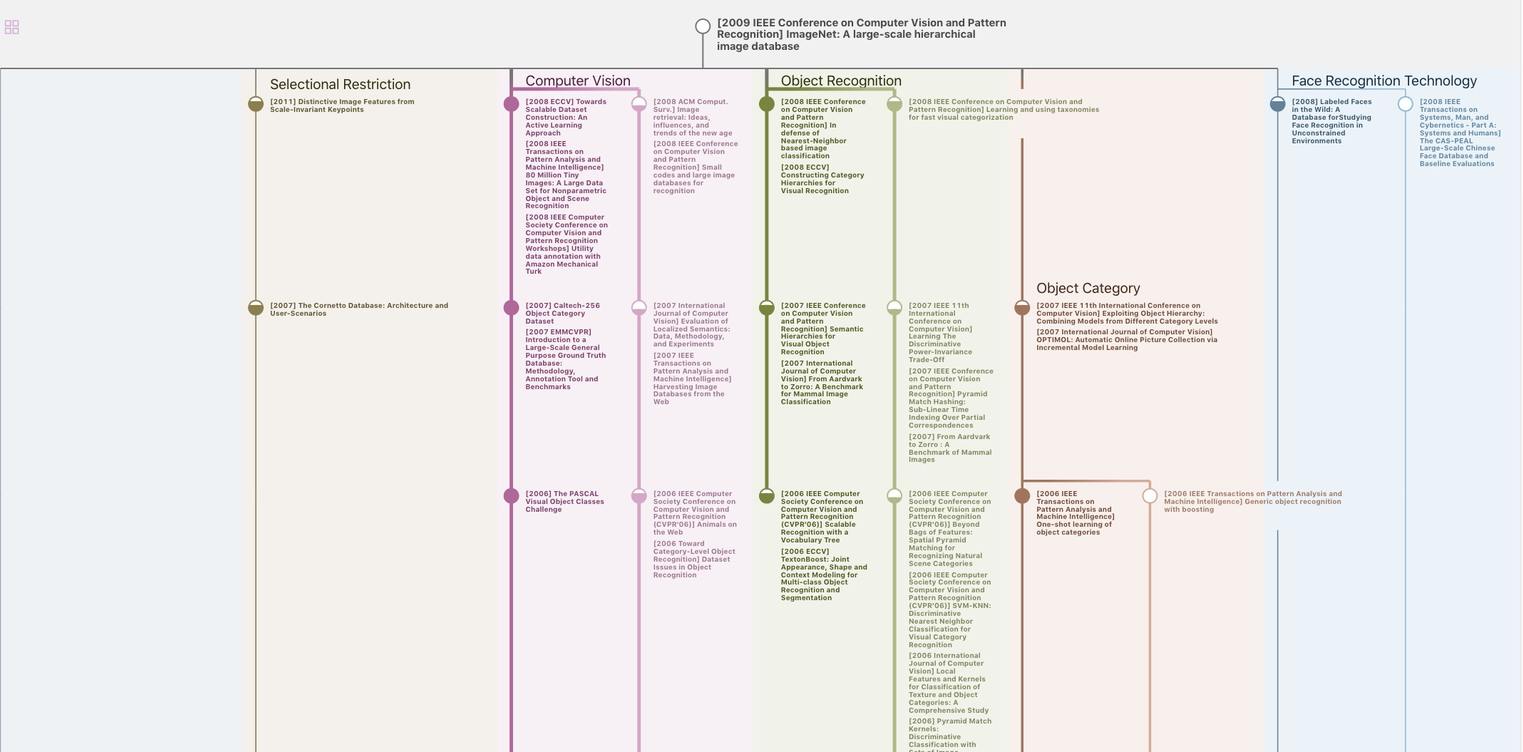
生成溯源树,研究论文发展脉络
Chat Paper
正在生成论文摘要