Enhancing Continual Deep Open-Set Recognition with Perceptive Unknown Feature Search
2023 6th International Conference on Applied Computational Intelligence in Information Systems (ACIIS)(2023)
摘要
Open-Set Recognition (OSR) and Continual Learning (CL) have recently gained attention from researchers aiming to advance sustainable Artificial Intelligence (AI). However, there has been limited research focused on the performance of rejecting unknown classes in continual OSR models. This paper provides empirical evidence that continual OSR models tend to experience a decline in their ability to reject unknown classes over successive task periods. To address this issue, we propose an initial solution of using two memory replays: one for samples of known features and another for pseudo-features associated with unknown classes. For generating features of these unknown classes, we propose a method called Perceptive Unknown Feature Search (PUFS) that involves locating features based on the positions of existing prototypes and then feeding them into an inverse network to obtain backbone features. To enhance the model, we modify the loss function by incorporating contrastive learning for unknown features. This improved model, named as Class-Incremental Quad-Channel Contrastive Prototype Networks (CI-QCCPN), outperforms its predecessor QCCPN as well as softmax-based classifiers with memory replay and achieves the highest average AUROC scores across various tasks and datasets. Our source code is available on https://github.com/gusti-alfarisy/ContinualDeepOSR.
更多查看译文
关键词
open-set recognition,continual learning,out-of-distribution detection,prototype learning,class-incremental learning
AI 理解论文
溯源树
样例
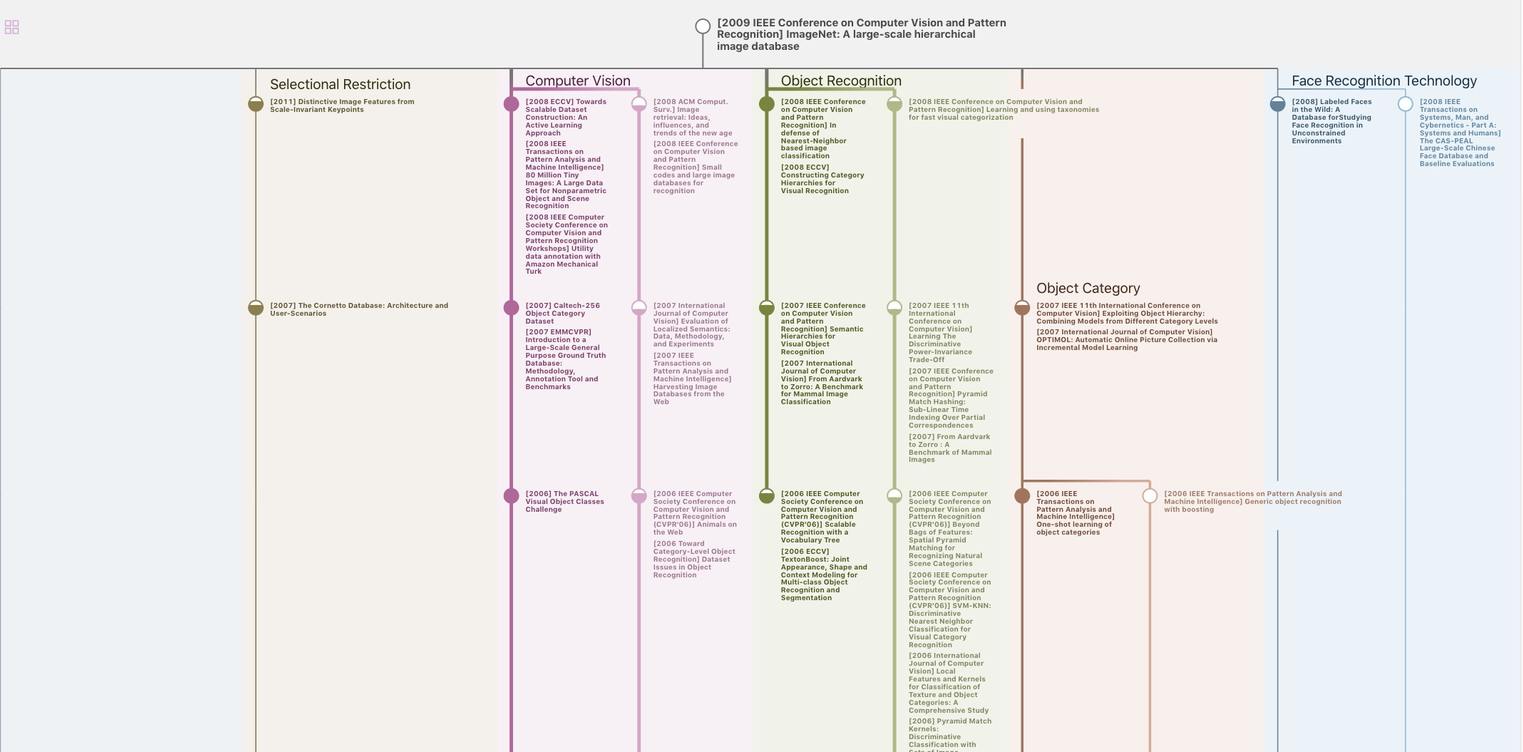
生成溯源树,研究论文发展脉络
Chat Paper
正在生成论文摘要