Spatial Correlation-Constrained Low-Rank Modeling for SAR Image Change Detection
IEEE TRANSACTIONS ON GEOSCIENCE AND REMOTE SENSING(2024)
摘要
Superpixel analysis is showing great potential for high-resolution synthetic aperture radar (SAR) image change detection (CD), as it uses a larger detection granularity and enhances computational efficiency. However, some deficiencies still exist. It is difficult for previous methods to extract the complete change regions from cluttered backgrounds. Meanwhile, the local spatial correlation and structure consistency are not well represented. To address the above problems and achieve better separation of changed and unchanged superpixels in complicated scenarios, we design a novel unsupervised CD framework from the perspective of low-rank matrix decomposition (LRMD) theory. The entire framework is carried out in two stages. First, the l(1)-norm sparsity constraint LRMD model is constructed to decompose change features into a low-rank component associated with background and a sparse component representing changed regions. Then, the local spatial correlation and structure consistency constraint are explicitly modeled by introducing a Laplacian regularization term. The unified model smooths the local similarity superpixels and enlarges the distance between changed regions and the background in the feature subspace. In this stage, the saliency difference image (DI) is generated to indicate the change probabilities of each superpixel. Furthermore, a classification refining (CR) module is designed to learn the projection from the change feature matrix to the saliency DI, which can further fine-tune such obscure regions and boost the binary classification. Extensive experiments on five challenging datasets from the TerraSAR-X sensor demonstrate the effectiveness and superiority of the proposed method.
更多查看译文
关键词
Sparse matrices,Radar polarimetry,Matrix decomposition,Feature extraction,Synthetic aperture radar,Correlation,Computational modeling,Change detection (CD),classification refining (CR),low-rank matrix decomposition (LRMD),synthetic aperture radar (SAR)
AI 理解论文
溯源树
样例
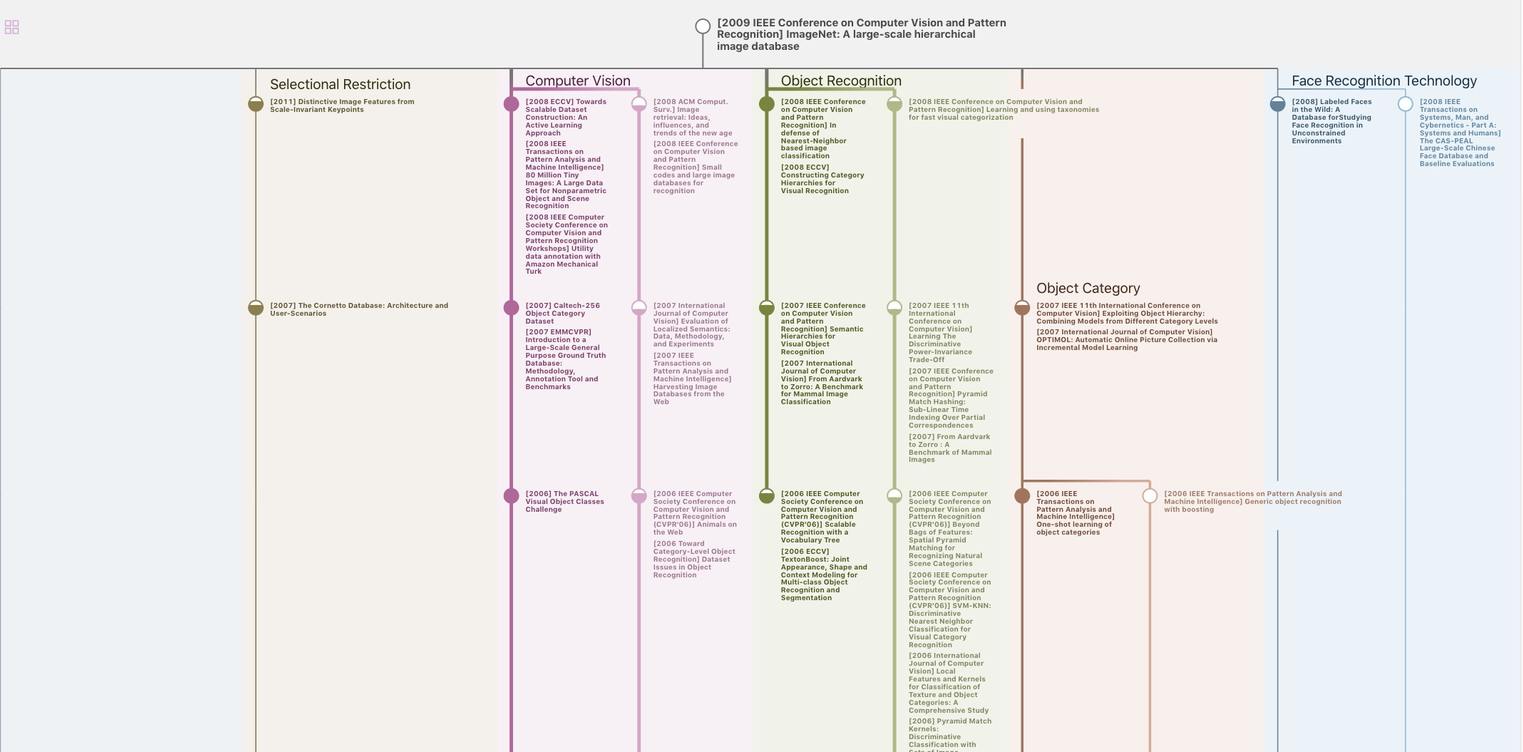
生成溯源树,研究论文发展脉络
Chat Paper
正在生成论文摘要