A sequential three-way decision model for classification with multilevel information gain and regret value optimization
INFORMATION SCIENCES(2024)
摘要
The sequential three-way decision (S3WD) model plays a vital role in solving classification problems. This model comprises three fundamental elements, namely, conditional probability, cost function, and decision thresholds. The typical conditional probability estimation methods are bifurcated into two branches: equivalence class-based methods, which coincide with human recognition, and machine learning-based methods that rely on big data for high precision. This study synergizes two branches by introducing a novel algorithm that integrates the K-nearest neighbor method and Bayes rule for estimating conditional probabilities, enhancing both interpretability and precision. Regarding the cost function and decision thresholds, the inherent weak point in existing cost-sensitive S3WD models is the computation of three pairwise adjacent regions with subjectively given cost values and decision thresholds. To overcome the subjective arbitrariness bottleneck, an objective function is established by extending the definitions of information gain and regret value across a multilevel granularity structure. Subsequently, an adapted particle swarm optimization algorithm is utilized to optimize the decision thresholds and tri-partitions. Accordingly, a novel framework of the S3WD model is constructed. Finally, extensive experimental results substantiate the effectiveness and superiority of the proposed model in the realm of classification, as evidenced from both technical and empirical standpoints.
更多查看译文
关键词
Sequential three-way decision,Information gain,Regret value,Granularity,Classification
AI 理解论文
溯源树
样例
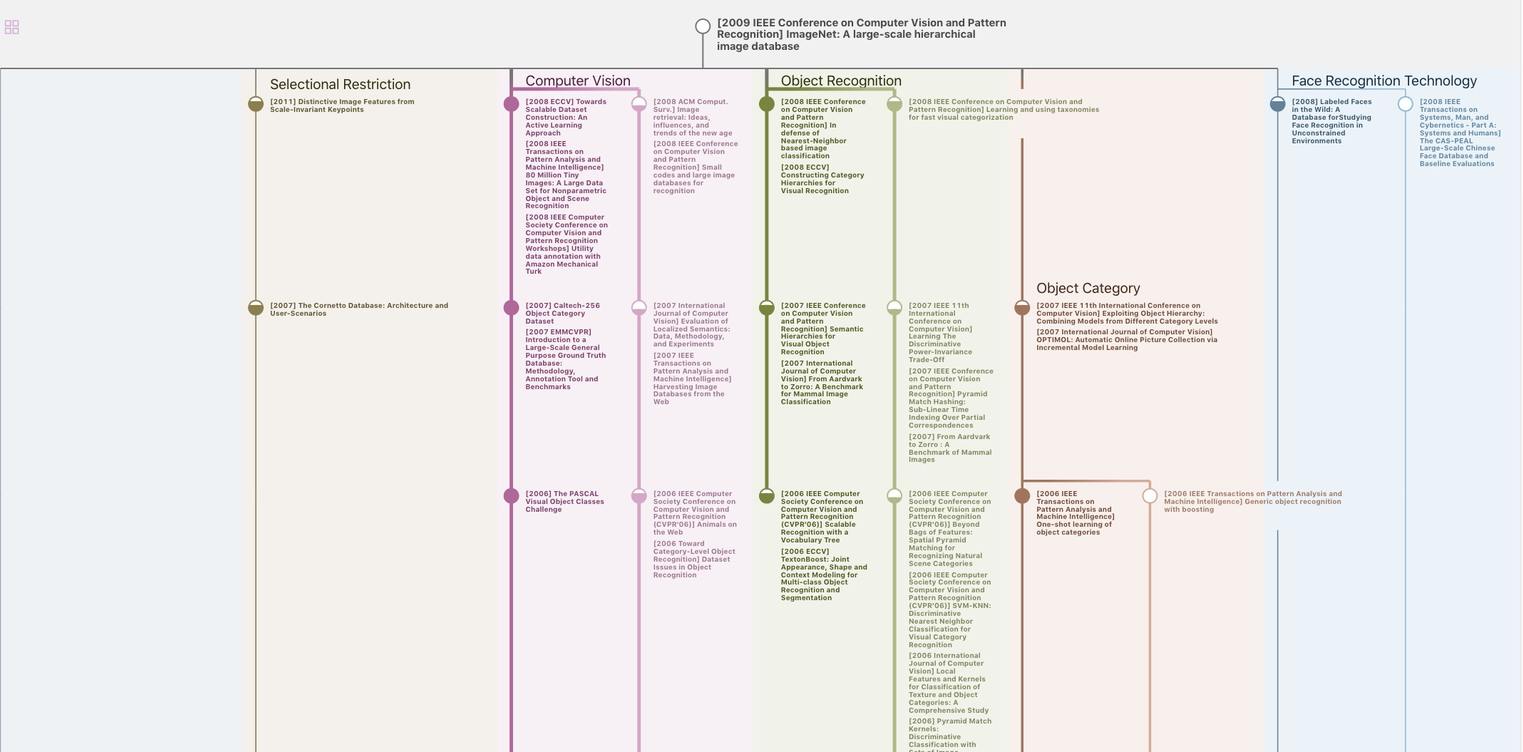
生成溯源树,研究论文发展脉络
Chat Paper
正在生成论文摘要