Universal Pyramid Adversarial Training for Improved ViT Performance
CoRR(2023)
摘要
Recently, Pyramid Adversarial training (Herrmann et al., 2022) has been shown
to be very effective for improving clean accuracy and distribution-shift
robustness of vision transformers. However, due to the iterative nature of
adversarial training, the technique is up to 7 times more expensive than
standard training. To make the method more efficient, we propose Universal
Pyramid Adversarial training, where we learn a single pyramid adversarial
pattern shared across the whole dataset instead of the sample-wise patterns.
With our proposed technique, we decrease the computational cost of Pyramid
Adversarial training by up to 70
on clean performance and distribution-shift robustness. In addition, to the
best of our knowledge, we are also the first to find that universal adversarial
training can be leveraged to improve clean model performance.
更多查看译文
AI 理解论文
溯源树
样例
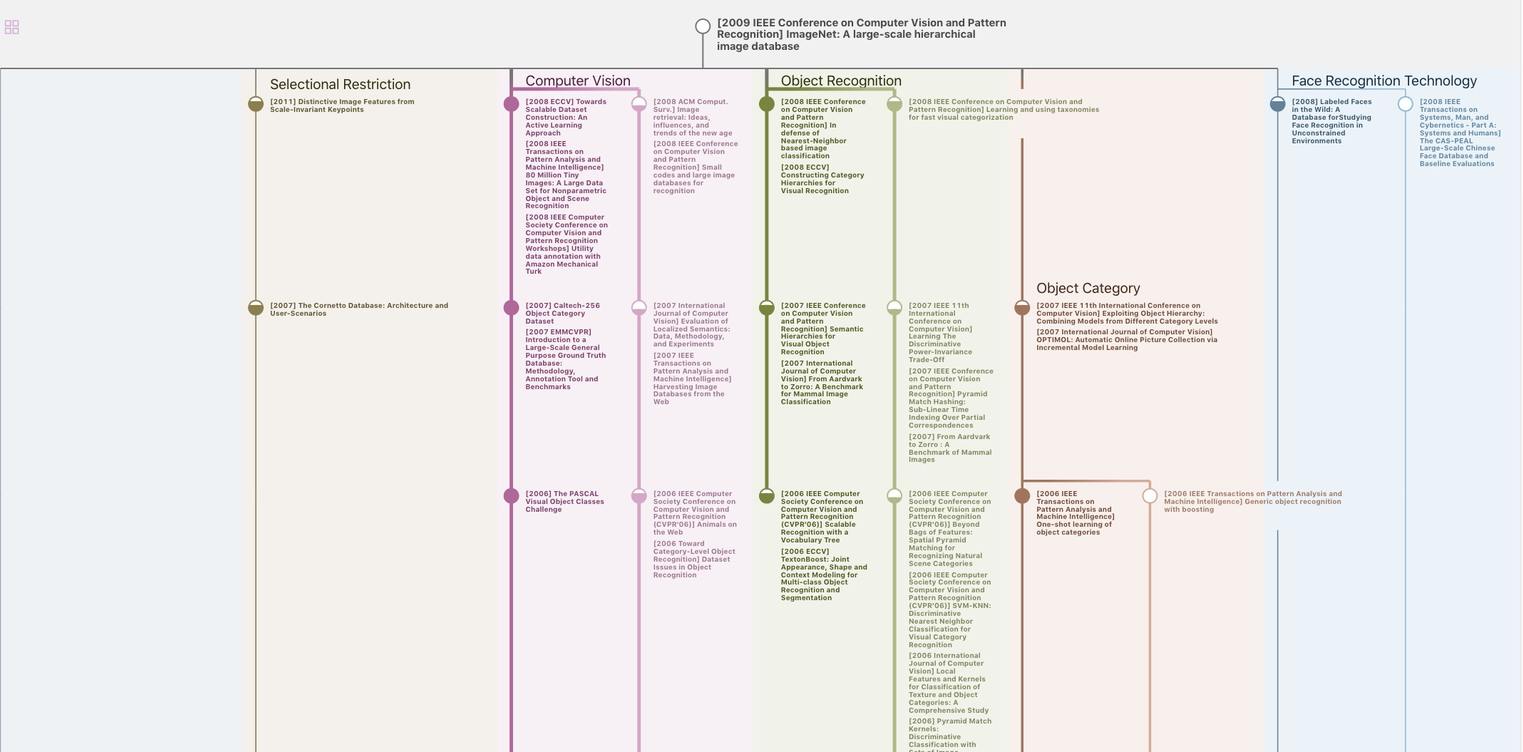
生成溯源树,研究论文发展脉络
Chat Paper
正在生成论文摘要