Dual Consistency-Constrained Learning for Unsupervised Visible-Infrared Person Re-Identification
IEEE TRANSACTIONS ON INFORMATION FORENSICS AND SECURITY(2024)
摘要
Unsupervised visible-infrared person re-identification (US-VI-ReID) aims at learning a cross-modality matching model under unsupervised conditions, which is an extremely important task for practical nighttime surveillance to retrieve a specific identity. Previous advanced US-VI-ReID works mainly focus on associating the positive cross-modality identities to optimize the feature extractor by off-line manners, inevitably resulting in error accumulation of incorrect off-line cross-modality associations in each training epoch due to the intra-modality and inter-modality discrepancies. They ignore the direct cross-modality feature interaction in the training process, i.e., the on-line representation learning and updating. Worse still, existing interaction methods are also susceptible to inter-modality differences, leading to unreliable heterogeneous neighborhood learning. To address the above issues, we propose a dual consistency-constrained learning framework (DCCL) simultaneously incorporating off-line cross-modality label refinement and on-line feature interaction learning. The basic idea is that the relations between cross-modality instance-instance and instance-identity should be consistent. More specifically, DCCL constructs an instance memory, an identity memory, and a domain memory for each modality. At the beginning of each training epoch, DCCL explores the off-line consistency of cross-modality instance-instance and instance-identity similarities to refine the reliable cross-modality identities. During the training, DCCL finds credible homogeneous and heterogeneous neighborhoods with on-line consistency between query-instance similarity and query-instance domain probability similarities for feature interaction in one batch, enhancing the robustness against intra-modality and inter-modality variations. Extensive experiments validate that our method significantly outperforms existing works, and even surpasses some supervised counterparts. The source code is available at https://github.com/yangbincv/DCCL.
更多查看译文
关键词
Training,Cameras,Feature extraction,Data mining,Surveillance,Annotations,Task analysis,Person re-identification,visible-infrared,unsupervised learning,cross-modality
AI 理解论文
溯源树
样例
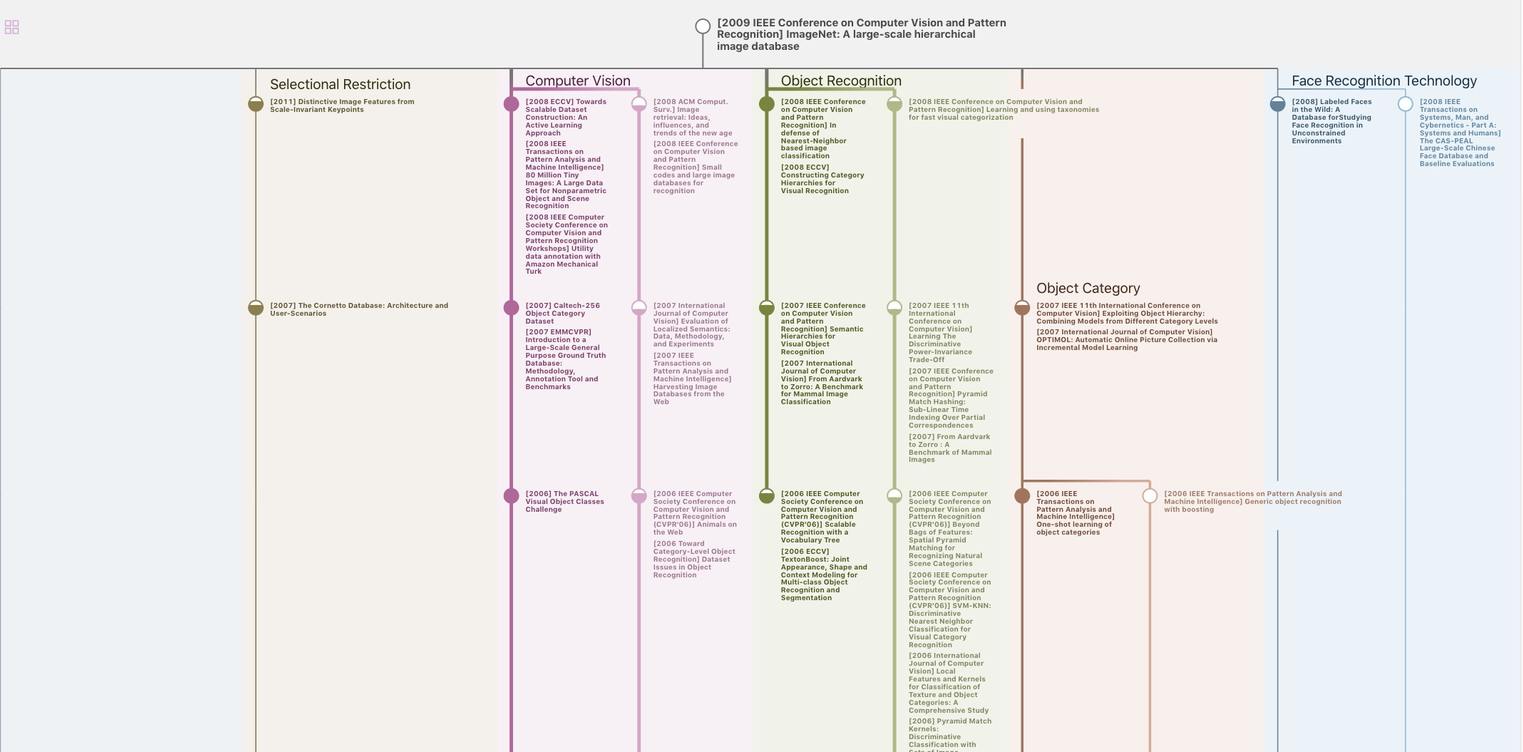
生成溯源树,研究论文发展脉络
Chat Paper
正在生成论文摘要