IoT-Based Strawberry Disease Detection With Wall-Mounted Monitoring Cameras.
IEEE Internet of Things Journal(2024)
摘要
This article proposes StrawberryTalk, an Internet of Things (IoT) platform for image-based strawberry disease detection. StrawberryTalk reuses the wall-mounted monitoring cameras without extra hardware cost. The contributions of StrawberryTalk are the utilization of IoT for automatic photo shoot and the wind detection mechanism to eliminate the obscure photos due to the wind effects. Also, the data preprocessing and the infection detection models are manipulated as IoT devices to simplify the implementation of the multicascade artificial intelligence (AI) models. We derive the relationship between the camera zoom factor and the distance between the camera and the strawberries for optimal disease detection. Accuracy of detection may be affected by obscure photos. In terms of eliminating obscure photos due to wind effect, we analytically derive the relationship between the wind alert delay and the number of obscure photos that must be retaken. For the greenhouses in the Bao Mountain, we only need to retake one photo. Based on the experiments, the mean average precision (mAP) of StrawberryTalk (to detect exact spots in a leaf) can be up to 92.37%, which is better than the previous approaches. To detect if a pot has infected leaves, the accuracy of StrawberryTalk can be up to 97.92% at the zoom factor
$30\times $
. In commercial operation, it is important to detect all infected strawberry pots. StrawberryTalk is able to detect all infected pots (i.e., recall is 100%) with a camera of zoom factor of
$12\times $
. The accuracy is 96.88%.
更多查看译文
关键词
Disease detection,hybrid deep learning,Internet of Things (IoT),machine learning,strawberry
AI 理解论文
溯源树
样例
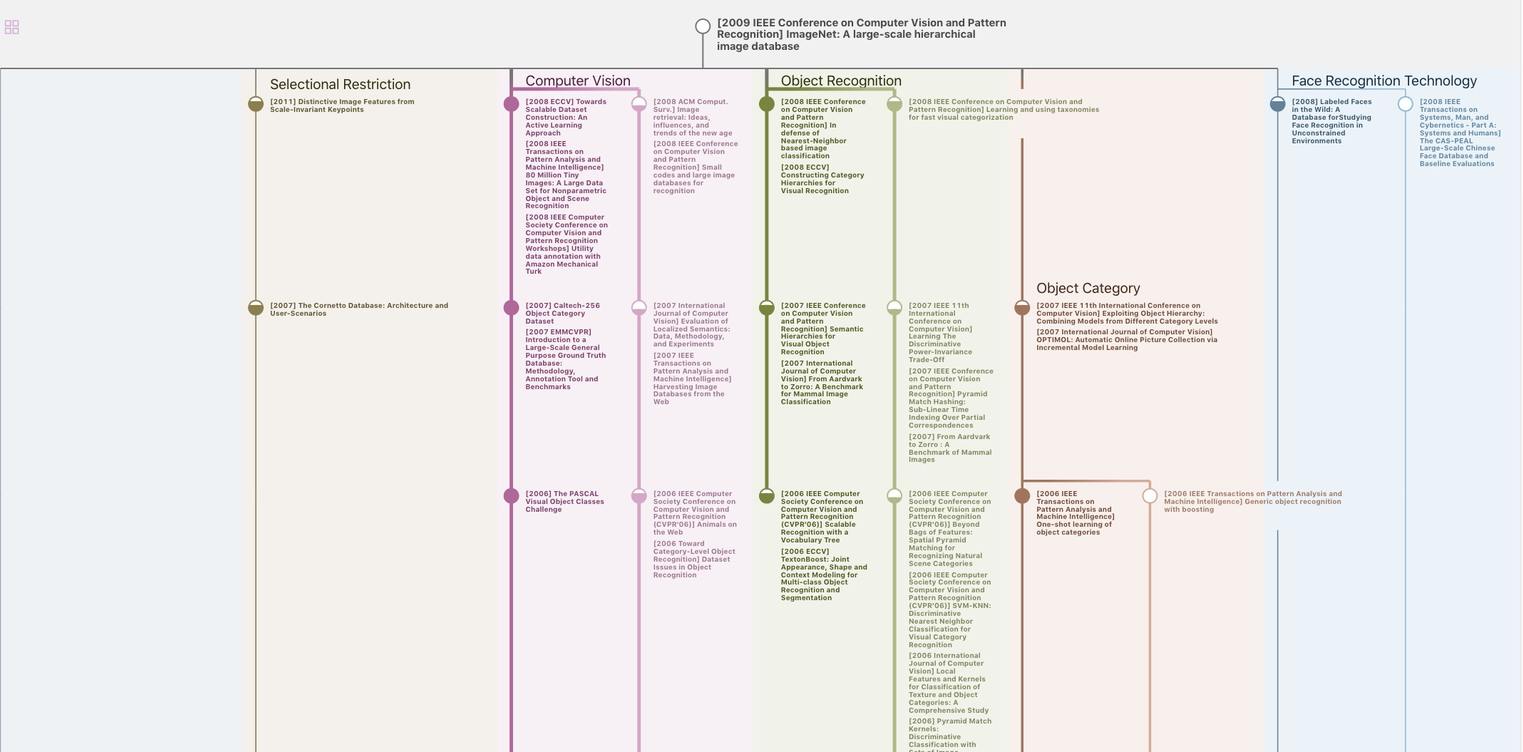
生成溯源树,研究论文发展脉络
Chat Paper
正在生成论文摘要