Feature Selection on Anonymized Datasets.
2023 IEEE Intl Conf on Dependable, Autonomic and Secure Computing, Intl Conf on Pervasive Intelligence and Computing, Intl Conf on Cloud and Big Data Computing, Intl Conf on Cyber Science and Technology Congress (DASC/PiCom/CBDCom/CyberSciTech)(2023)
摘要
Privacy concerns have become increasingly prominent in data analysis, leading to adoption of privacy-preserving approaches including anonymization techniques. However, the application of anonymization techniques may impact the significance of features in the anonymized dataset, potentially affecting the accuracy and reliability of subsequent machine learning tasks. In this paper, we present a comprehensive comparative study to investigate the preservation of feature significance in the original dataset versus its anonymized version. We conduct a set of experiments to provide valuable insights into the implications of anonymization techniques on preserving the features' relevancy, which advances privacy-aware data analysis in various domains.
更多查看译文
关键词
Feature Selection,k-anonymity,R-diversity,t-closeness
AI 理解论文
溯源树
样例
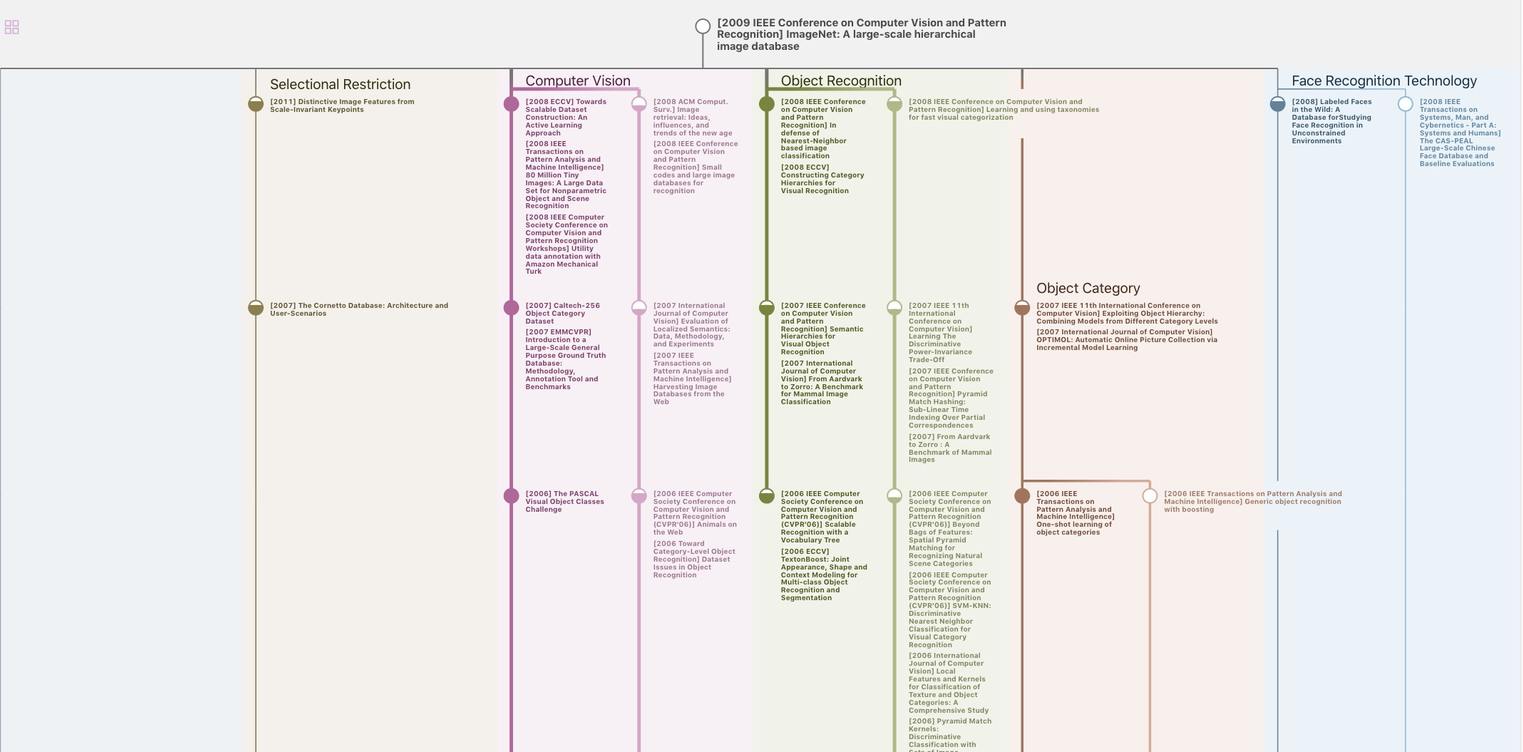
生成溯源树,研究论文发展脉络
Chat Paper
正在生成论文摘要