An Improved Nested Training Approach to Mitigate Clean-label Attacks against Malware Classifiers
MILCOM 2023 - 2023 IEEE MILITARY COMMUNICATIONS CONFERENCE(2023)
摘要
Machine Learning (ML) models are being adopted as state of the art tools to defend systems against cybersecurity threats. Despite their high accuracy, such models remain vulnerable to various types of adversarial attacks. In cybersecurity, ML models are frequently trained on crowd-sourced datasets, which naturally allows attackers to introduce backdoor samples into malware detectors by using the recently proposed class of clean-label poisoning attacks. The presence of such backdoor samples in training datasets can later be used by attackers to modify a trained model's behavior. Nested Training is an ensemble-based method for detecting poisoned samples by leveraging disagreements within the outputs of a diverse set of ensemble models on training data points. In the paper, we adapt the Nested Training approach to various cyber domains such as Android APKs and PDF Files, in which the distributions of data and the effects of poisoning have quite different properties. We then compare the resulting improved Nested Training approach with existing methods and demonstrate the ability of the approach to mitigate the effects of clean-label poisoned attacks and to recover an original Machine Learning model's accuracy.
更多查看译文
关键词
Machine Learning,Adversarial Machine Learning,Malware Detection Systems
AI 理解论文
溯源树
样例
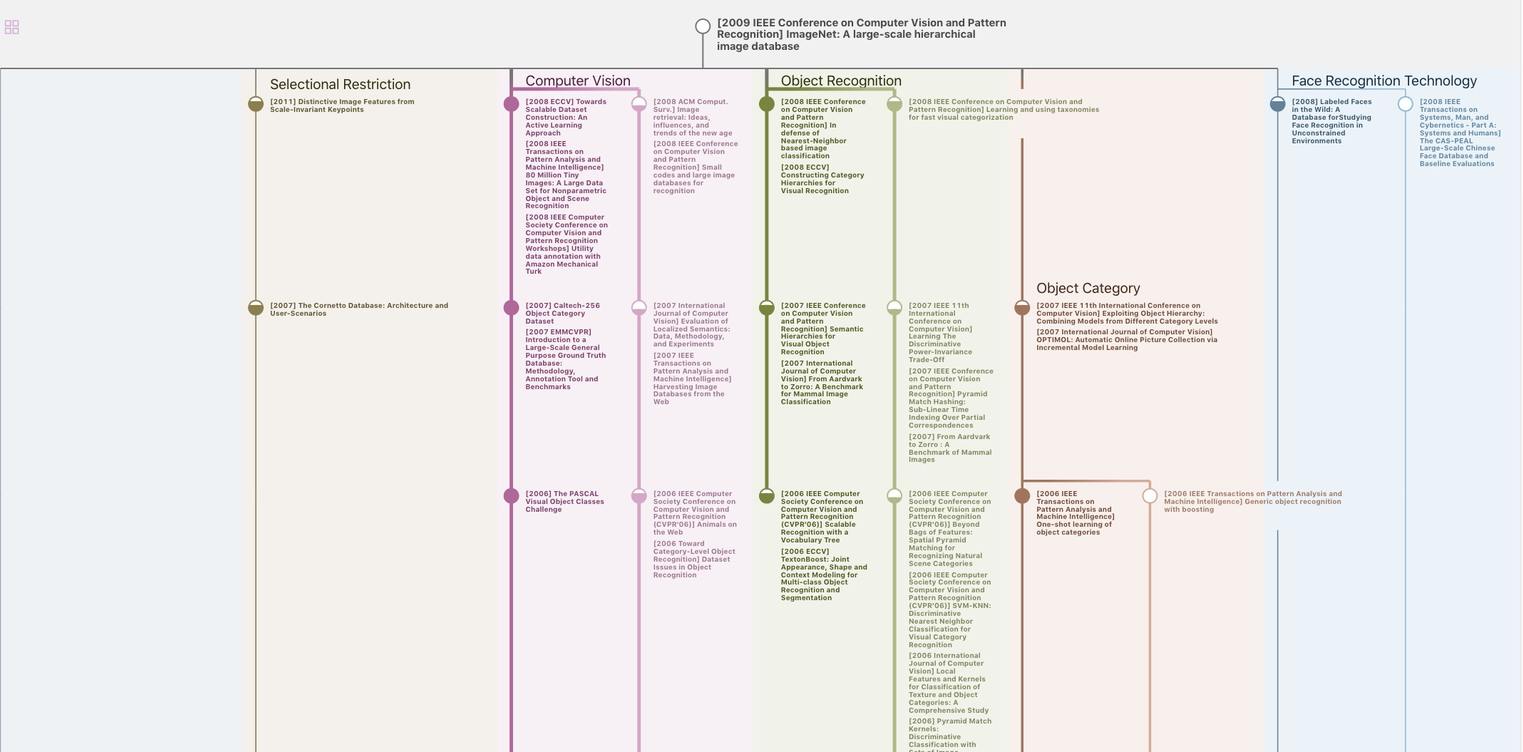
生成溯源树,研究论文发展脉络
Chat Paper
正在生成论文摘要