A Dual Perspective of Human Motion Analysis-3D Pose Estimation and 2D Trajectory Prediction
2023 IEEE/CVF INTERNATIONAL CONFERENCE ON COMPUTER VISION WORKSHOPS, ICCVW(2023)
摘要
Anticipating human motion based on given sequences is a challenging and crucial task in computer vision and machine learning, enabling machines to understand human behaviors effectively. Precise prediction of human pose and motion trajectory holds great significance for various applications, including autonomous driving, robotics, and virtual reality. This paper presents a novel approach to address the interconnected tasks of estimating human motion, represented as 3D poses or 2D trajectories, and predicting future motions using 2D images and human pose/position sequences jointly. We propose an encoder-decoder architecture that leverages Transformer networks with a selfattention mechanism, utilizing visual context features, combined with an LSTM to model human motion kinematics. Our approach demonstrates consistent and remarkable improvements over existing methods, both quantitatively and qualitatively. Extensive experiments conducted on diverse public datasets, such as GTA-IM and PROX for 3D human pose estimation, and ETH and UCY combined datasets for 2D trajectory prediction, showcase that our method substantially reduces prediction errors compared to the current state-of-the-art methods.
更多查看译文
AI 理解论文
溯源树
样例
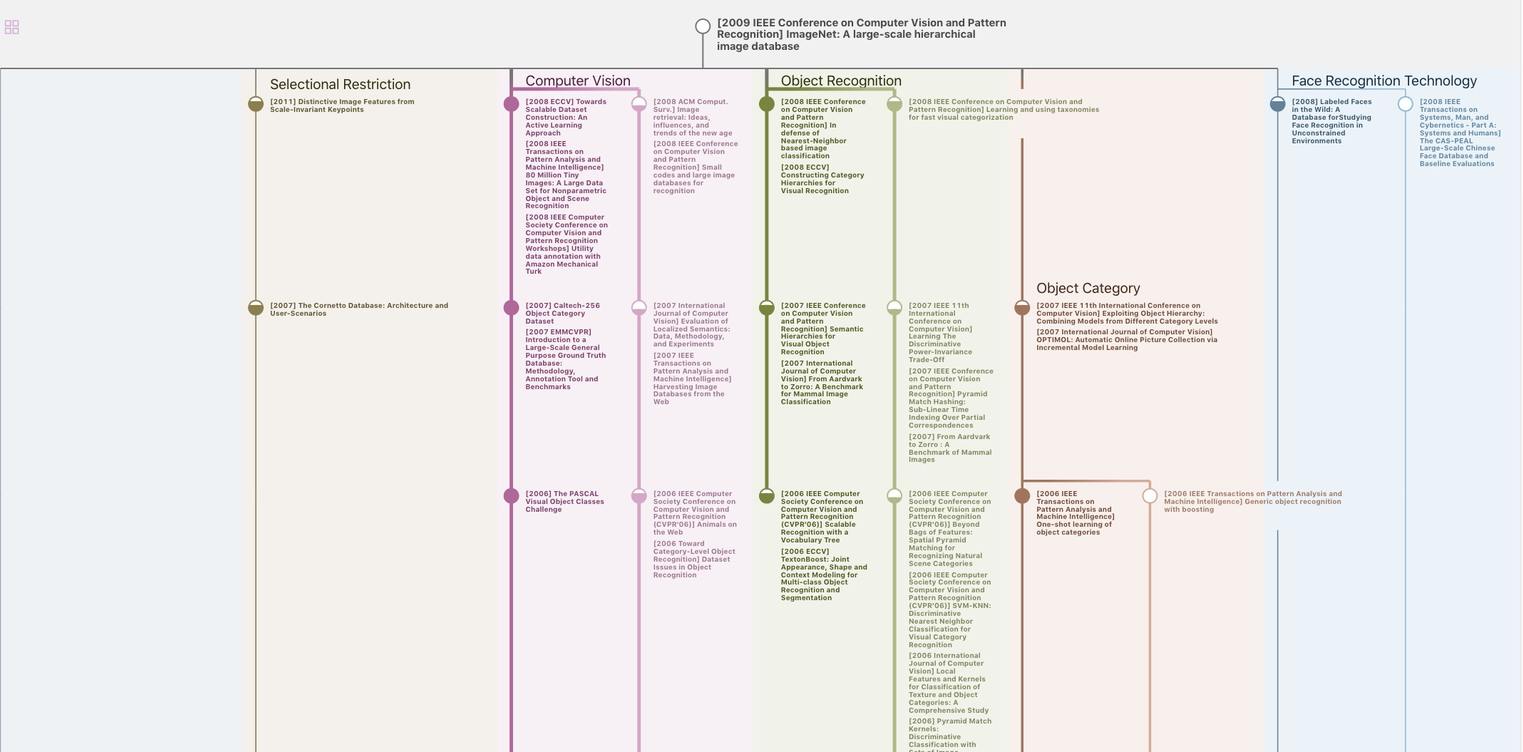
生成溯源树,研究论文发展脉络
Chat Paper
正在生成论文摘要