Flashback for Continual Learning
2023 IEEE/CVF INTERNATIONAL CONFERENCE ON COMPUTER VISION WORKSHOPS, ICCVW(2023)
摘要
To strike a delicate balance between model stability and plasticity of continual learning, previous approaches have adopted strategies to guide model updates on new data to preserve old knowledge while implicitly absorbing new information through task objective function (e.g. classification loss). However, our goal is to achieve this balance more explicitly, proposing a bi-directional regularization that guides the model in preserving existing knowledge and actively absorbing new knowledge. To address this, we propose the Flashback Learning (FL) algorithm, a two-stage training approach that seamlessly integrates with diverse methods from different continual learning categories. FL creates two knowledge bases; one with high plasticity to control learning and one conservative to prevent forgetting, then it guides the model update using these two knowledge bases. FL significantly improves baseline methods on common image classification datasets such as CIFAR-10, CIFAR-100, and Tiny ImageNet in various settings.
更多查看译文
AI 理解论文
溯源树
样例
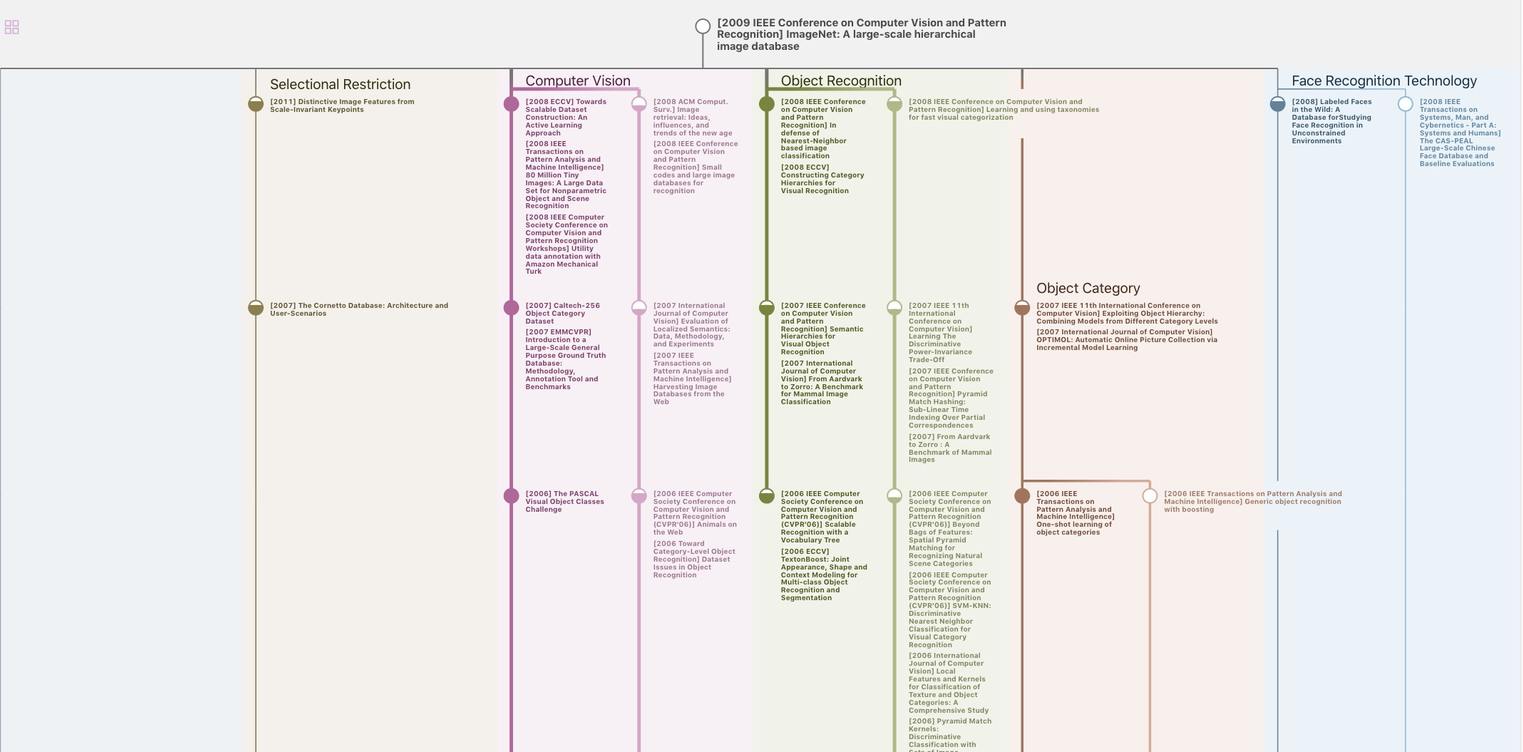
生成溯源树,研究论文发展脉络
Chat Paper
正在生成论文摘要