Diff3DHPE: A Diffusion Model for 3D Human Pose Estimation
2023 IEEE/CVF INTERNATIONAL CONFERENCE ON COMPUTER VISION WORKSHOPS, ICCVW(2023)
摘要
Accurately estimating 3D human pose (3D HPE) and joint locations using only 2D keypoints is challenging. The noise in the predictions produced by conventional 2D human pose estimators often impeded the accuracy. In this paper, we present a diffusion-based model for 3D pose estimation, named Diff3DHPE, inspired by diffusion models' noise distillation abilities. The proposed model takes a temporal sequence of 2D keypoints as the input of a GNN backbone model to extract the 3D pose from Gaussian noise using a diffusion process during training. The model then refines it using a reverse diffusion process. To overcome over-smoothing issues in GNNs, Diff3DHPE is integrated with a discretized partial differential equation, which makes it a particular form of Graph Neural Diffusion (GRAND). Extensive experiments show that our model outperforms current state-of-the-art methods on two benchmark datasets, Human3.6M and MPI-INF-3DHP, achieving up to 39.1% improvement in MPJPE on MPI-INF-3DHP. The code is available at https://github.com/socoolzjm/Diff3DHPE.
更多查看译文
AI 理解论文
溯源树
样例
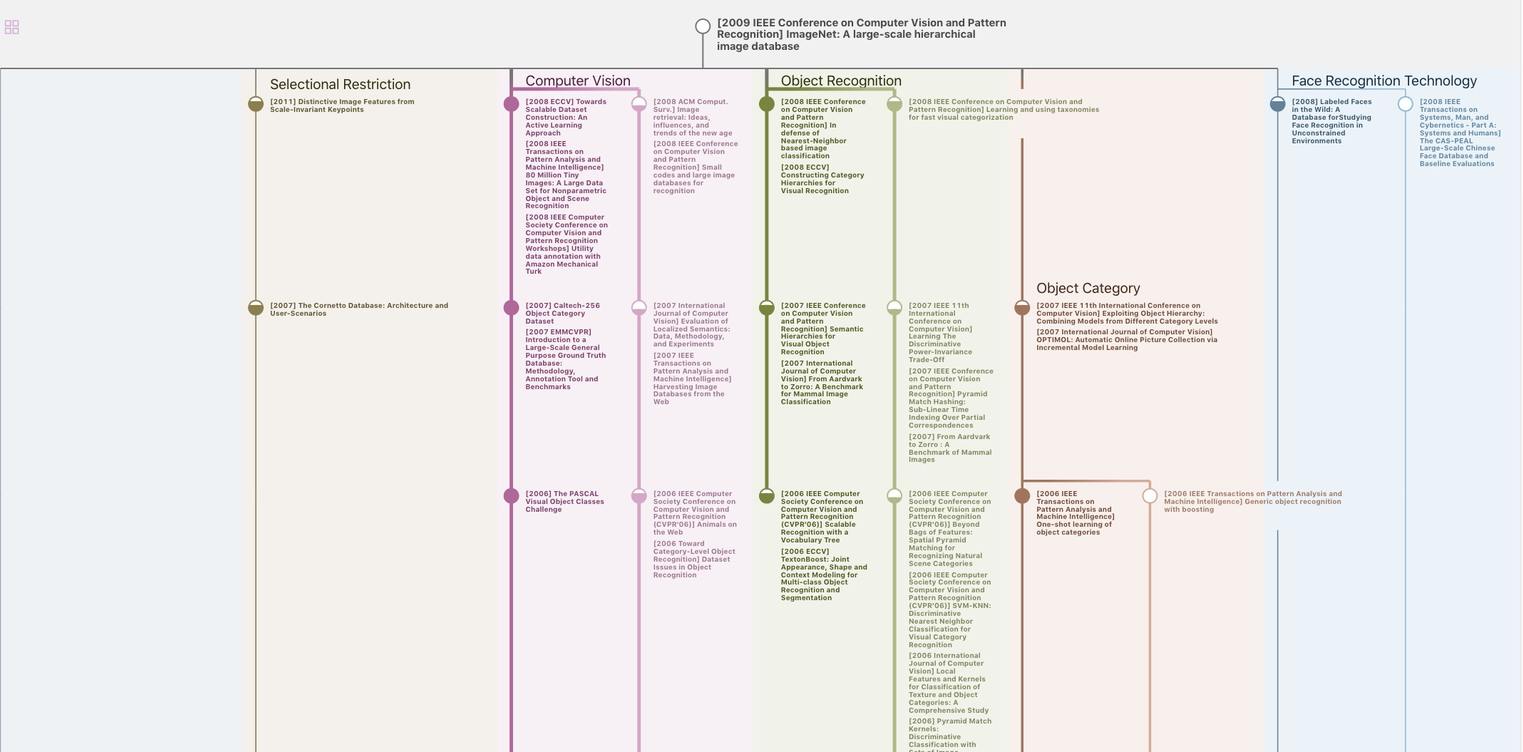
生成溯源树,研究论文发展脉络
Chat Paper
正在生成论文摘要