Computationally Efficient Early Prognosis of the Outcome of Comatose Cardiac Arrest Survivors Using Slow-Wave Activity Features in EEG.
2023 Computing in Cardiology (CinC)(2023)
摘要
This study, part of ‘Predicting Neurological Recovery from Coma After Cardiac Arrest: The George B. Moody PhysioNet Challenge 2023’, evaluated a computationally efficient method in predicting cardiac arrest (CA) survivors' prognoses using electroencephalography (EEG) recordings of a dataset provided for participants. Authors' team Cerenion developed a random forest based machine learning algorithm. A feature set of channel-by-channel root mean square power of a well-described neurophysiological EEG phenomenon called slow-wave activity (SWA), with time elapsed since CA, was used. Five-fold cross-validation, using 80 % of the provided training set of EEG recordings from 607 out of 1020 patients, was used for evaluation. The held-out 20 % of data were used for testing and evaluating a final model trained on the full 80 % of the training data. Cross-validated results, evaluated at 72 hours after CA, for predicting the outcome were: AUROC 70 %, AUPRC 78 %, accuracy 68 %, F-measure 64 %. Evaluating the challenge metric on the training data at times of 12, 24, 48, and 72 hours after CA provided scores of: 0.32, 0.40, 0.64, and 0.58, respectively. The hidden validation and test sets were not used, earning no rank. The results show promise in using SWA power features in predicting the outcomes of comatose CA patients.
更多查看译文
AI 理解论文
溯源树
样例
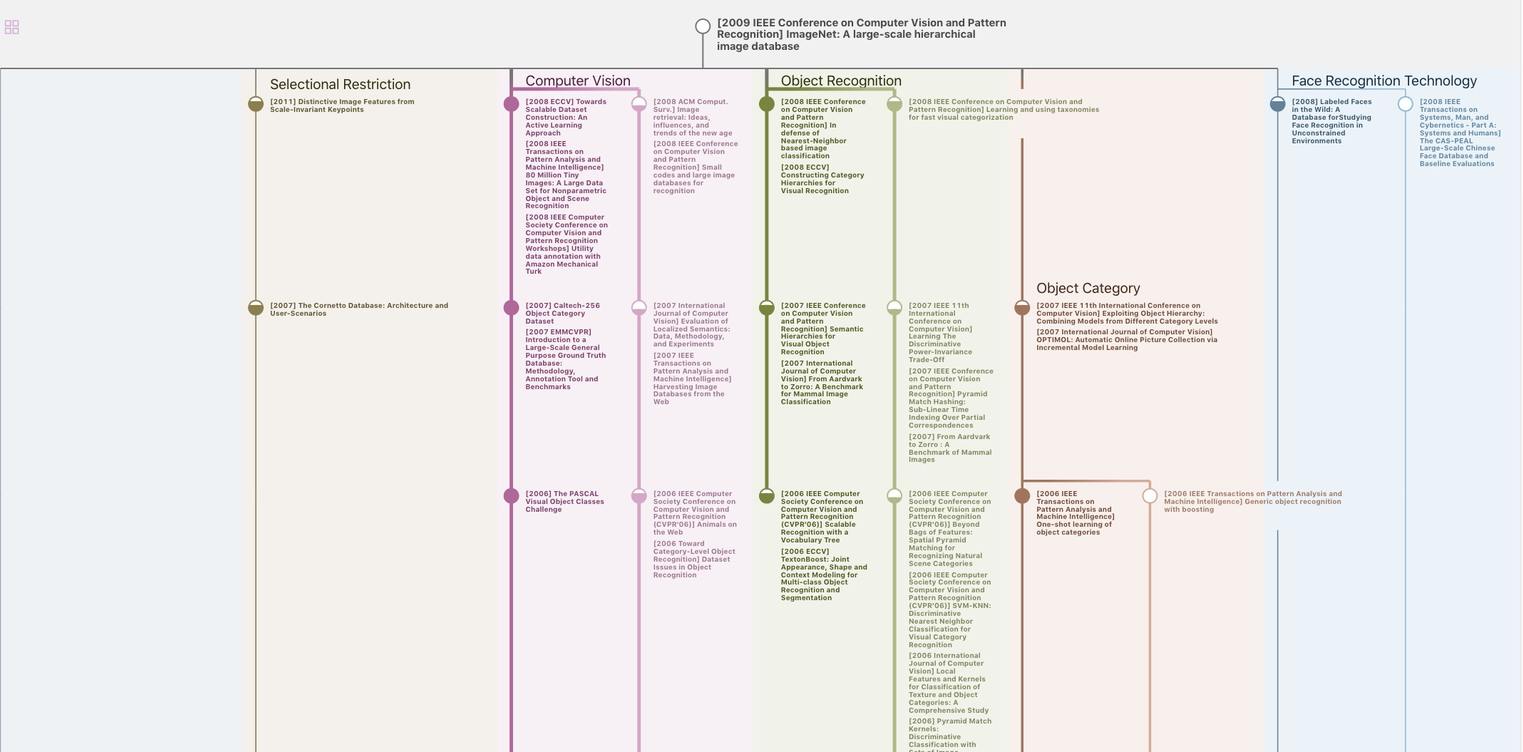
生成溯源树,研究论文发展脉络
Chat Paper
正在生成论文摘要