Predicting Comatose Patient's Outcome Using Brain Functional Connectivity with a Random Forest Model.
2023 Computing in Cardiology (CinC)(2023)
摘要
As part of the ‘Predicting Neurological Recovery from Coma After Cardiac Arrest: The George B. Moody PhysioNet Challenge 2023’, our team DEIB_POLIMI explored the predictive power of graph topological features extracted from brain connectivity networks, computed using electroencephalogram (EEG) recordings. We investigated the performance of two different phase synchronization measures on the delta band to compute channel-wise EEG connectivity, the weighted phase lagging index and the corrected imaginary phase locking value (ciPLV). Using ciPLV, we computed patients' functional brain networks and characterized their topology by extracting centrality, efficiency, and clusterization graph measures, resulting in 60 features. These features were then concatenated with the mean synchronization of each channel, and patients' clinical information, for a total of 85 features. Using a random forest model we achieved an official Challenge Score of 0.431 (ranked 23rd out of 36 teams) on the hidden test set.
更多查看译文
AI 理解论文
溯源树
样例
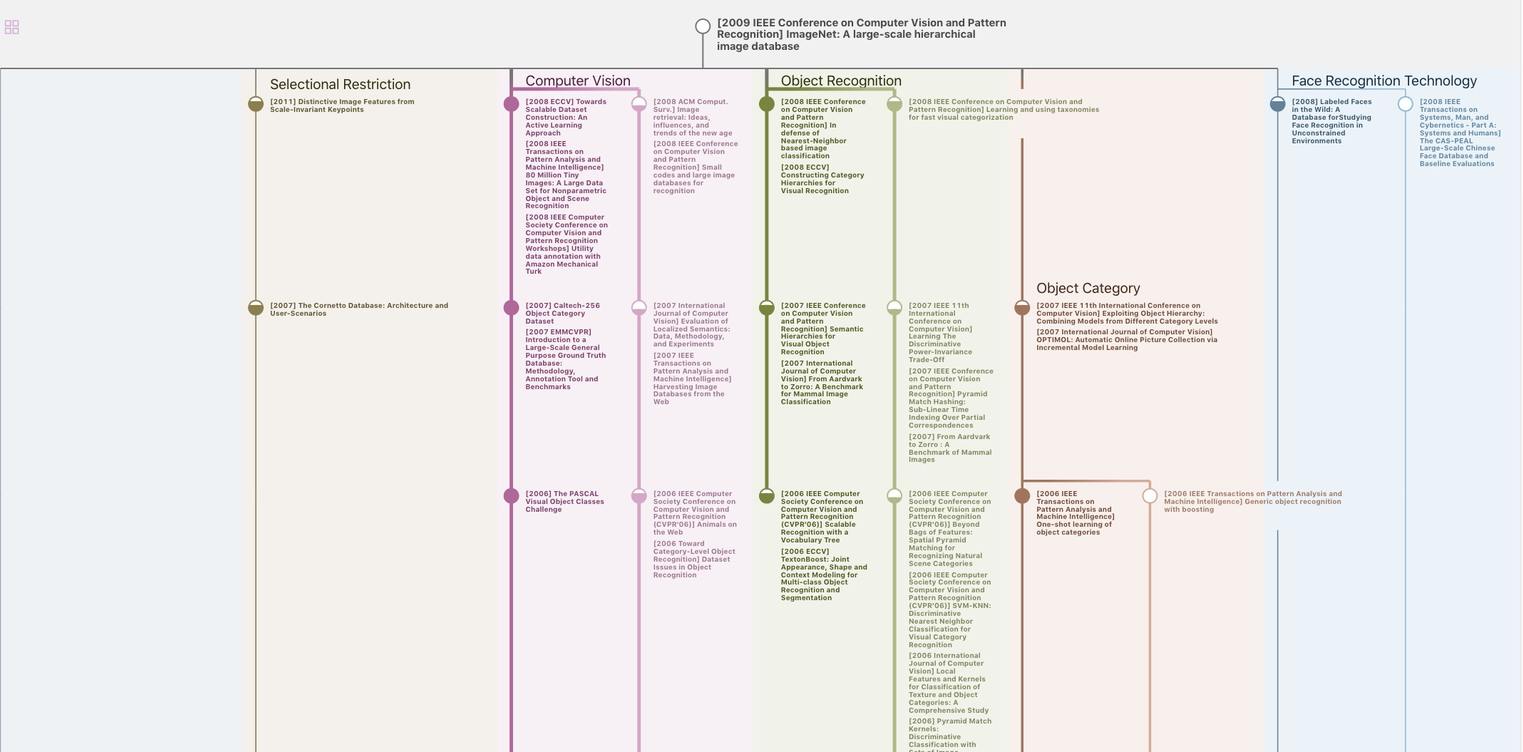
生成溯源树,研究论文发展脉络
Chat Paper
正在生成论文摘要