Parameter Estimation for Personalized Cardiac Models via Active Learning.
2023 Computing in Cardiology (CinC)(2023)
摘要
Personalized cardiac models are crucial intervention tools for a multitude of cardiac health issues. As cardiac simulations become more complex and expensive, machine learning (ML) models demonstrated the potential to enable efficient model personalization and cardiac tissue parameter estimation. A common approach depends on “globally” accurate ML models trained with large simulation data to predict tissue parameters. Such a global ML model is not only expensive to train, but its success also relies on the assumption that real-world data would fall within the range of the training data. We establish a novel active-learning method for cardiac parameter estimation by steering the training of the ML model towards the unknown region of interest in the parameter space.
更多查看译文
AI 理解论文
溯源树
样例
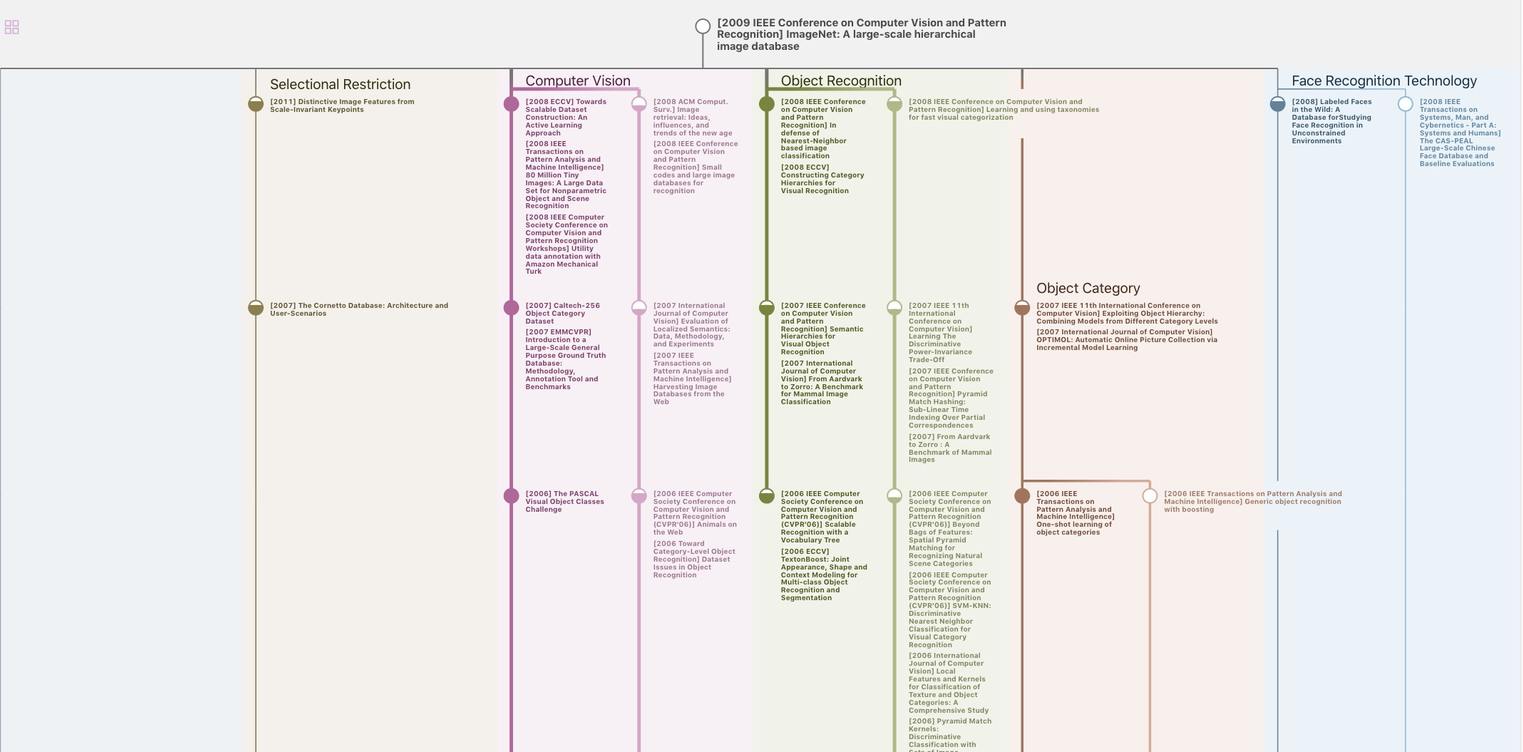
生成溯源树,研究论文发展脉络
Chat Paper
正在生成论文摘要