Machine Learning-Based Probabilistic Forecasting of Wind Power Generation: A Combined Bootstrap and Cumulant Method
IEEE TRANSACTIONS ON POWER SYSTEMS(2024)
摘要
Probabilistic forecasting provides complete probability information of renewable generation and load, which assists the diverse decision-making tasks in power systems under uncertainties. Conventional machine learning-based probabilistic forecasting methods usually consider the predictive uncertainty following prior distributional assumptions. This article develops a novel combined bootstrap and cumulant (CBC) method to generate nonparametric predictive distribution using higher order statistics for probabilistic forecasting. The CBC method successfully integrates machine learning with conditional moments and cumulants to describe the overall predictive uncertainty. A bootstrap-based conditional moment estimation method is proposed to quantify both the epistemic and aleatory uncertainties involved in machine learning. Higher order cumulants are utilized for overall uncertainty quantification based on the estimated conditional moments with its unique additivity. Three types of series expansions including Gram-Charlier, Edgeworth, and Cornish-Fisher expansions are adopted to improve the overall performance and the generalization ability. Comprehensive numerical studies using the actual wind power data validate the effectiveness of the proposed CBC method.
更多查看译文
关键词
Uncertainty,Forecasting,Wind power generation,Predictive models,Probabilistic logic,Estimation,Machine learning,Probabilistic forecasting,bootstrap,cumulant,machine learning,uncertainty quantification,wind power
AI 理解论文
溯源树
样例
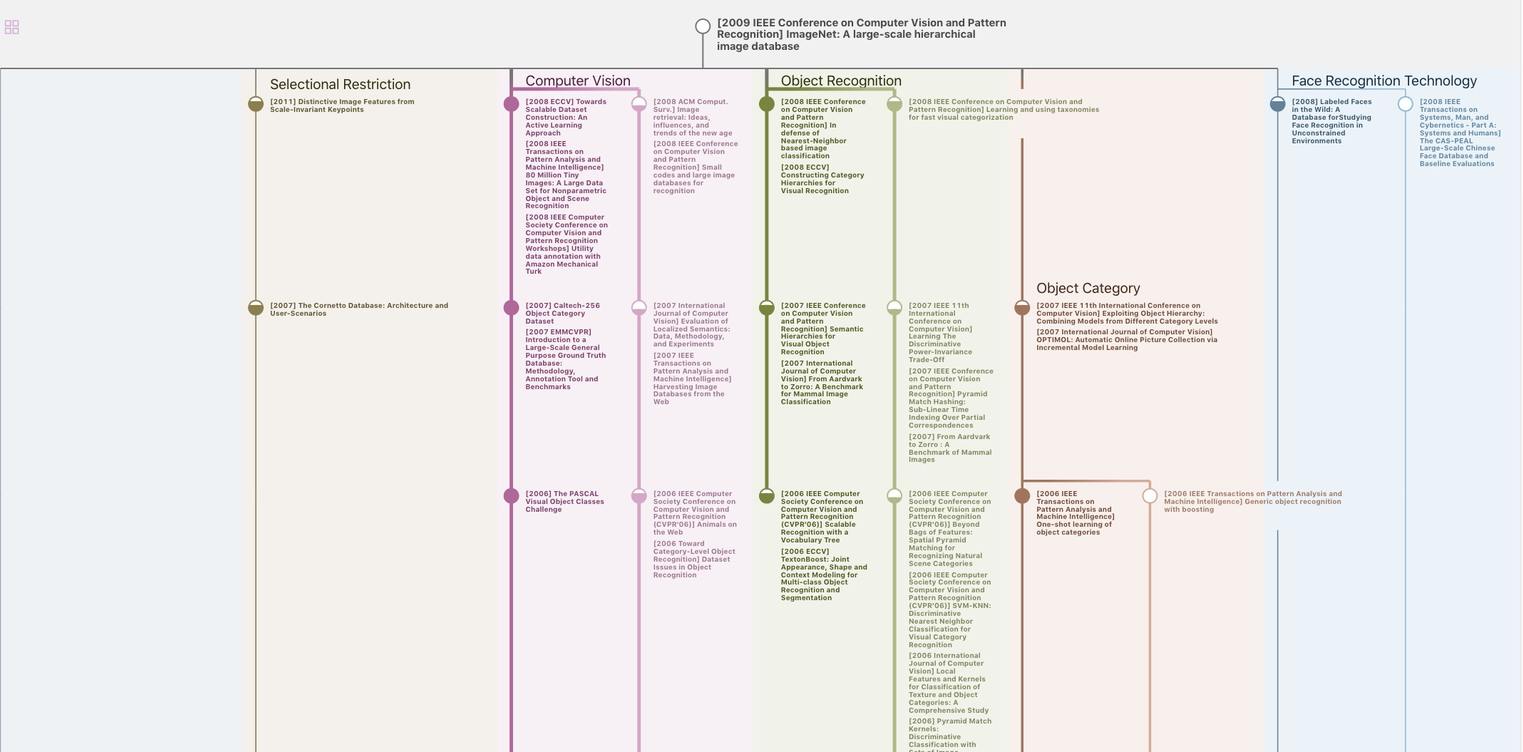
生成溯源树,研究论文发展脉络
Chat Paper
正在生成论文摘要