Multi-vehicle collaborative perception driven by parallel intelligence under communication constraints.
DTPI(2023)
摘要
Multi-vehicle collaborative perception can provide more comprehensive information for intelligent vehicles by sharing sensory data. This helps overcome issues like blind spots and occlusion, realizing safer driving decisions. However, real-world scenarios often have limited communication resources. Existing collaborative perception methods treat communication solely as the means for transmitting information, without considering the interplay between the spatial and temporal perception needs of vehicles and the available communication resources, which affects the collaborative effectiveness. In this paper, we propose a multi-vehicle collaborative perception method based on parallel intelligence, which maps channel conditions, vehicle spatio-temporal location and vehicle perception demand into virtual space. We utilized parallel intelligence to train the resource allocation decision model, which takes into account the deep coupling between the perception demand of vehicles and the communication resources. Then guides the vehicles to achieve distributed independent decision-making by using resource allocation decision model. To evaluate our method, we implemented it on the OpenCDA autonomous driving simulation platform. The experimental results show that the parallel-intelligence collaborative perception algorithm proposed in this paper can autonomously learn the timing and content of the collaboration through training. Compared to the traditional centralized allocation strategies, our method improves perceptual satisfaction by 12.1% and reduces communication loss by 1.8%, which effectively improves the collaborative perception effect.
更多查看译文
关键词
Parallel intelligence,Autonomous driving,Collaborative perception,Distributed decision
AI 理解论文
溯源树
样例
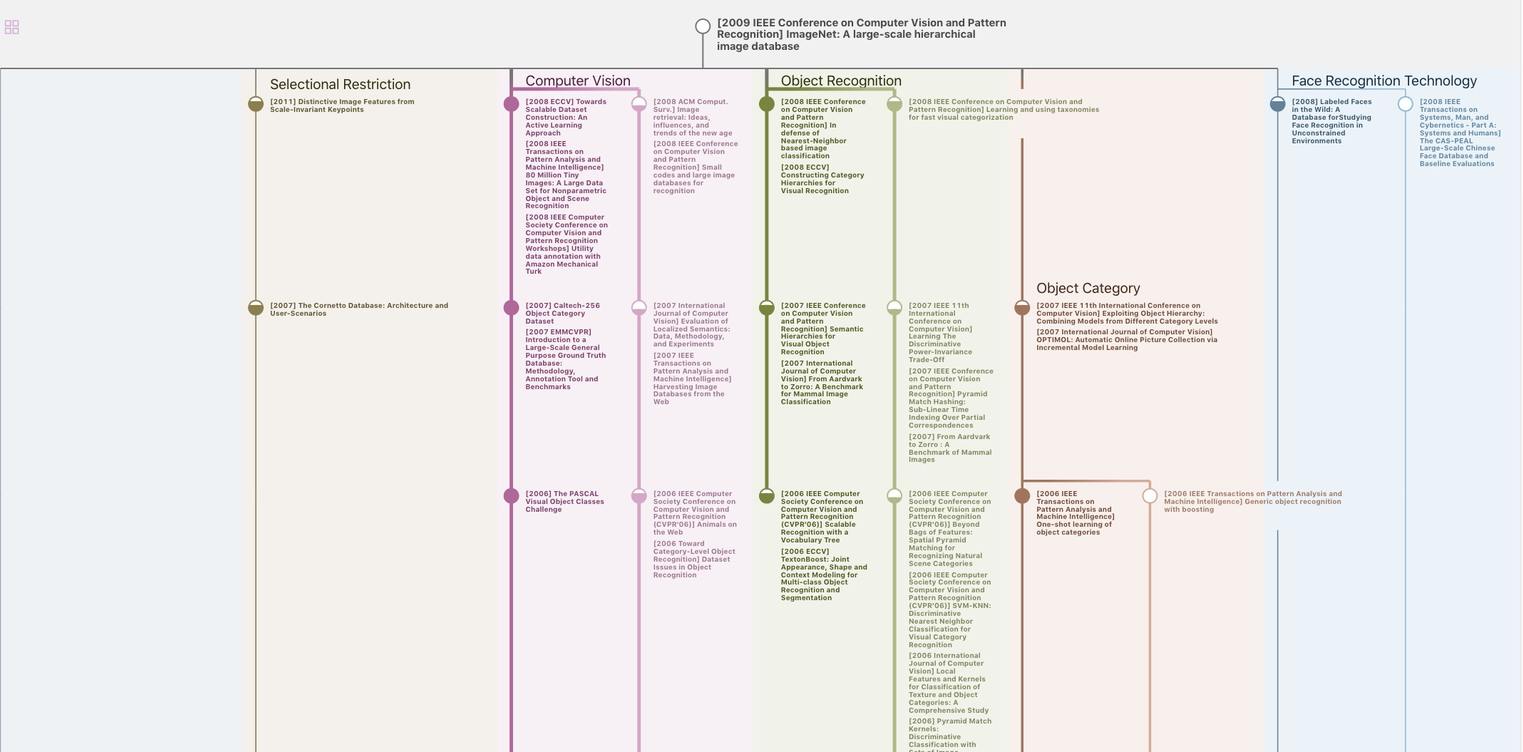
生成溯源树,研究论文发展脉络
Chat Paper
正在生成论文摘要