Bearing Fault Diagnosis with DDCNN Based on Intelligent Feature Fusion Strategy in Strong Noise
CMC-COMPUTERS MATERIALS & CONTINUA(2023)
摘要
Intelligent fault diagnosis in modern mechanical equipment maintenance is increasingly adopting deep learning technology. However, conventional bearing fault diagnosis models often suffer from low accuracy and unstable performance in noisy environments due to their reliance on a single input data. Therefore, this paper proposes a dual-channel convolutional neural network (DDCNN) model that leverages dual data inputs. The DDCNN model introduces two key improvements. Firstly, one of the channels substitutes its convolution with a larger kernel, simplifying the structure while addressing the lack of global information and shallow features. Secondly, the feature layer combines data from different sensors based on their primary and secondary importance, extracting details through small kernel convolution for primary data and obtaining global information through large kernel convolution for secondary data. Extensive experiments conducted on two-bearing fault datasets demonstrate the superiority of the two-channel convolution model, exhibiting high accuracy and robustness even in strong noise environments. Notably, it achieved an impressive 98.84% accuracy at a Signal to Noise Ratio (SNR) of -4 dB, outperforming other advanced convolutional models.
更多查看译文
关键词
Fault diagnosis,dual-data,dual-channel,feature fusion,noise-resistance
AI 理解论文
溯源树
样例
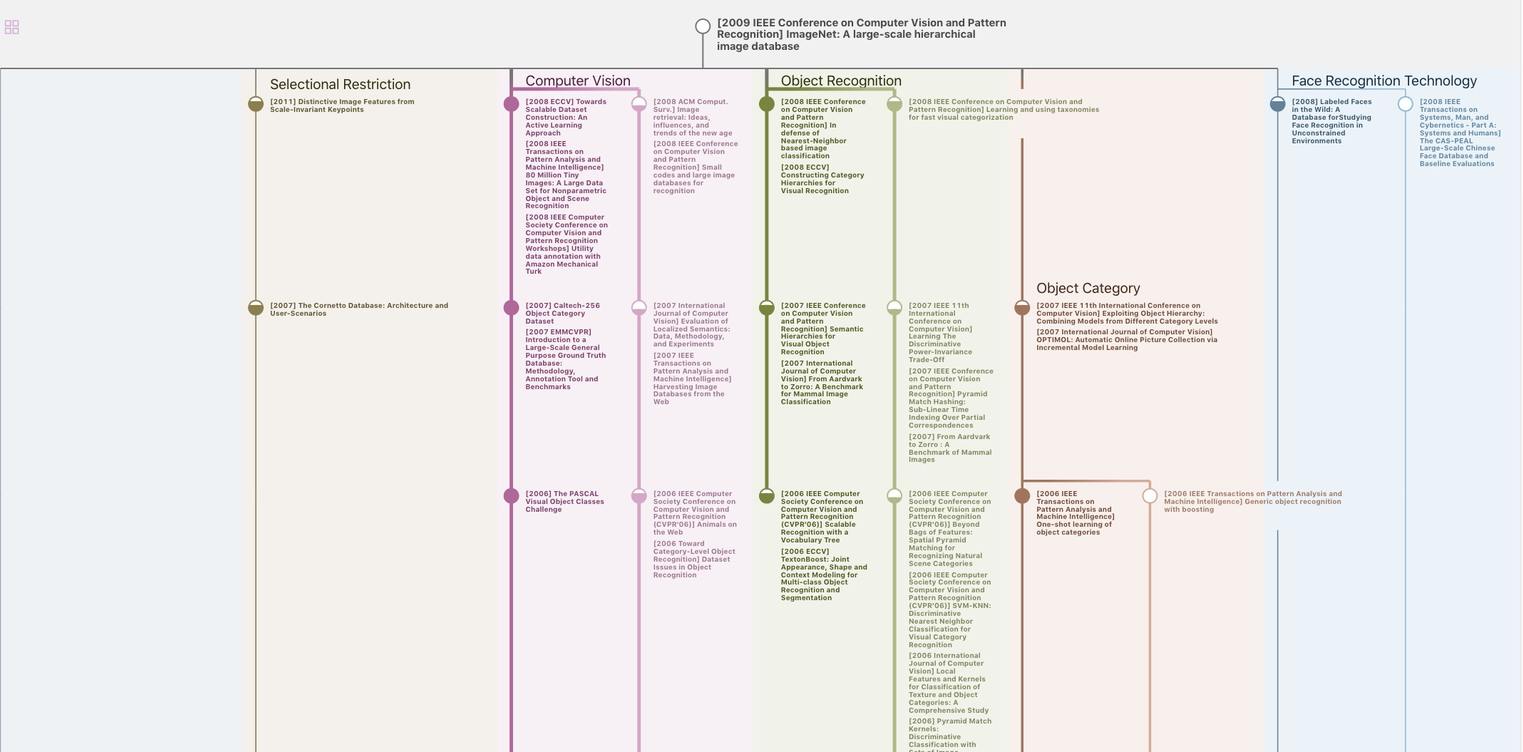
生成溯源树,研究论文发展脉络
Chat Paper
正在生成论文摘要