Precise and Robust Sidewalk Detection: Leveraging Ensemble Learning to Surpass LLM Limitations in Urban Environments
crossref(2023)
摘要
Abstract The study demonstrates the effectiveness of a robust ensemble model specifically designed for accurate sidewalk detection - a crucial aspect of road safety and urban planning. The model's performance was evaluated on Cityscapes, Ade20k, and the Boston Dataset. The results indicate that the ensemble model surpasses the individual models, achieving mIOU scores of 93.1\%, 90.3\%, and 90.6\% on these datasets under ideal conditions. Furthermore, the ensemble model displayed consistent performance levels, even under challenging conditions, with a gradual decrease in efficiency observed only in various noise types such as Salt-and-Pepper and Speckle. In contrast, despite its slight advantage in ideal scenarios, the state-of-the-art ONE-PEACE Large Language Model (LLM) showed a notable drop in performance in noisy environments. These findings highlight the robustness and dependability of the ensemble model, making it a promising tool for enhancing road safety and managing curb space in complex urban environments.
更多查看译文
AI 理解论文
溯源树
样例
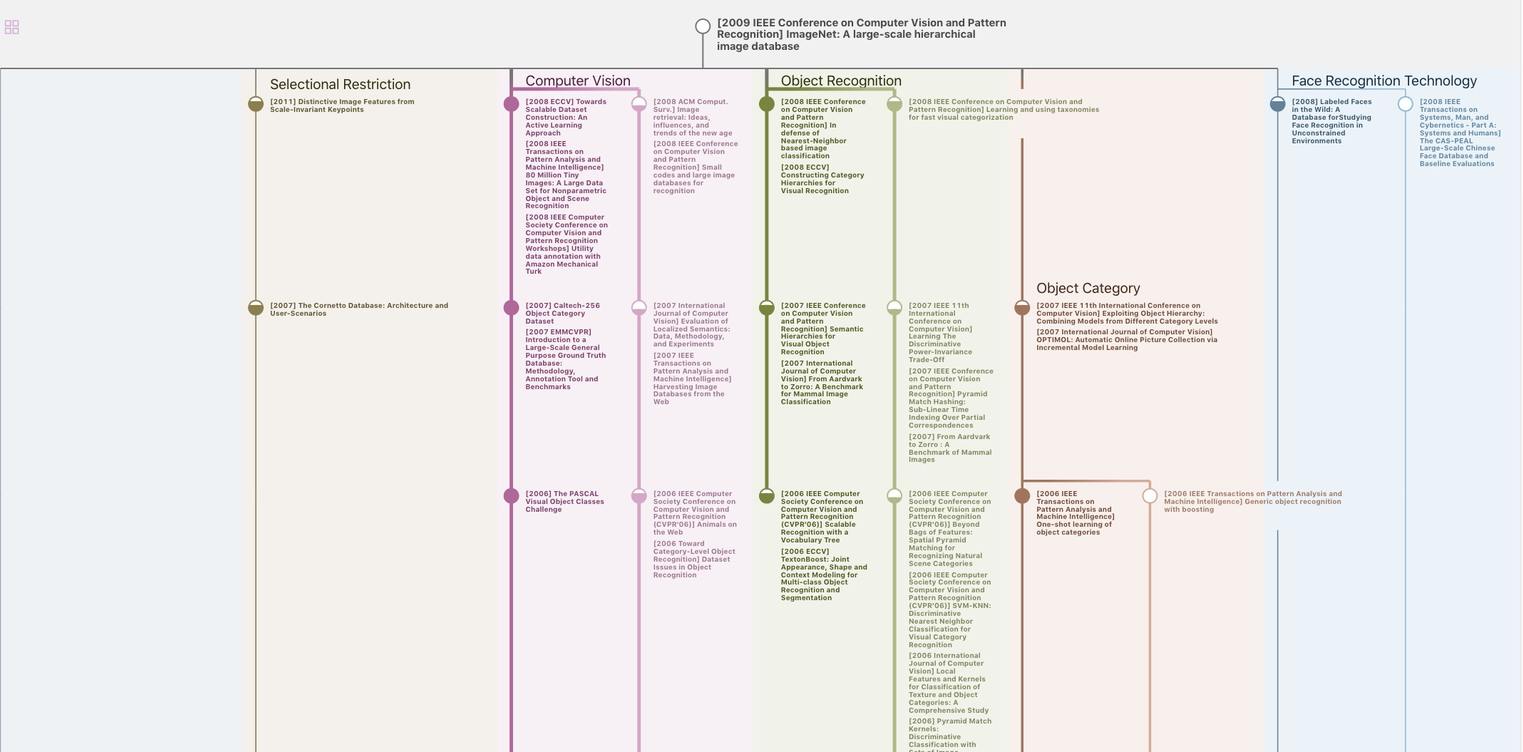
生成溯源树,研究论文发展脉络
Chat Paper
正在生成论文摘要