A scalable, distributed framework for significant subgroup discovery
KNOWLEDGE-BASED SYSTEMS(2024)
摘要
Subgroup discovery is a supervised data mining technique having many applications in medical domains, market basket analysis, and social media analysis. It helps in mining subgroups (or patterns) with a high association to a target property, measured using a quality function. However, the process is computationally intensive as it is necessary to go through the search space of all subgroups to find the top-k interesting ones w.r.t. the quality function. Further, as we verify many associations, it is quite possible that a certain level of association might be achieved by chance. To address this issue, the state-of-the-art TopKWY algorithm employs permutation testing to control false discoveries. Still, testing multiple subgroups against thousands of permuted target labels further increases computational complexity. Additionally, TopKWY is limited to a specific quality function and lacks a parallel/distributed implementation to handle scalability challenges. In this paper, we propose a parallel and distributed framework for subgroup discovery named ParaDiS that extends permutation testing to a broader class of quality functions. ParaDiS scales to large datasets while effectively controlling the false discovery rate. It features different optimizations to reduce communication/computation overheads and a distributed best-first search strategy to improve pruning across different workers. We compare its performance for several real-world datasets and achieve an order of magnitude reduction in the execution time compared to the sequential approach.
更多查看译文
关键词
Distributed subgroup discovery,Irregular structure,Significant subgroup,Closed pattern
AI 理解论文
溯源树
样例
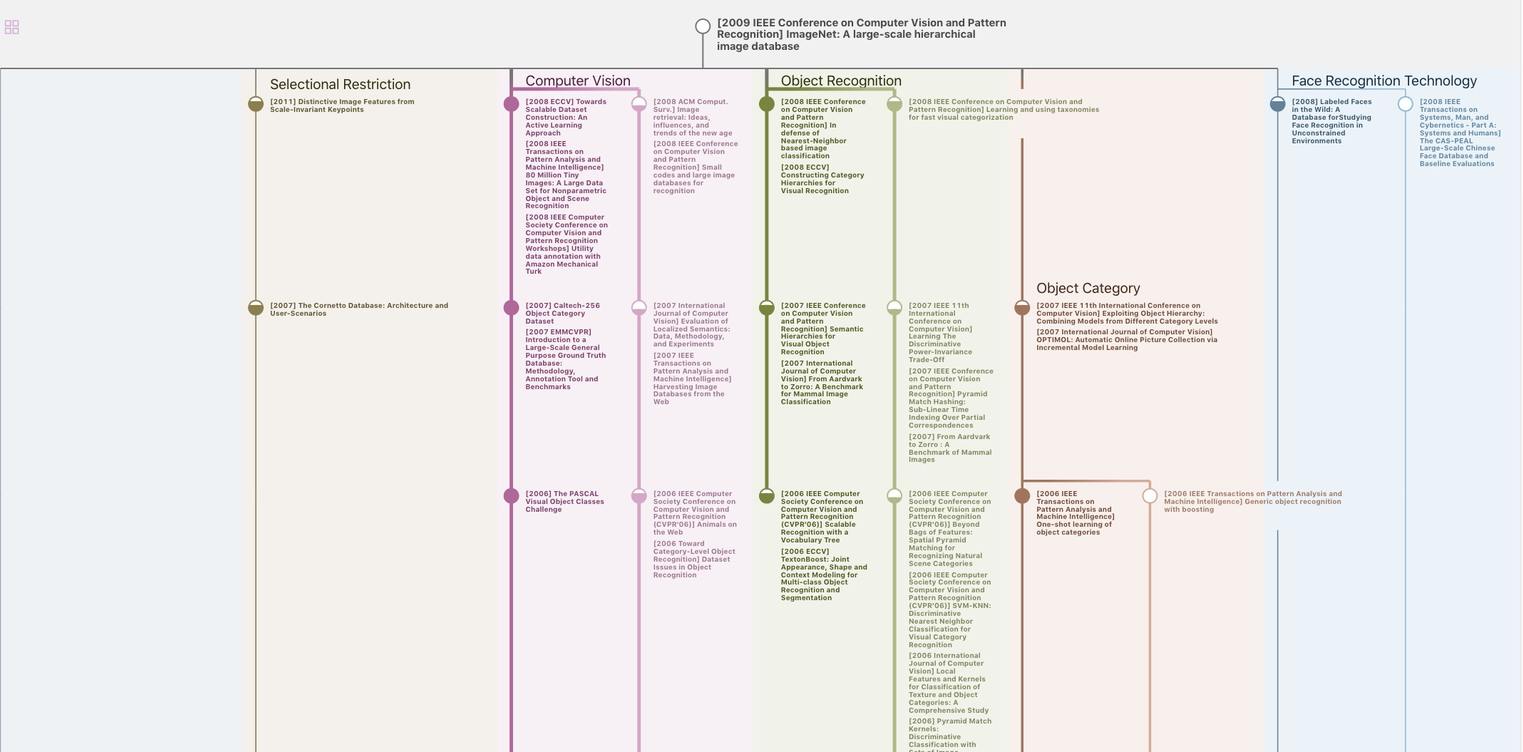
生成溯源树,研究论文发展脉络
Chat Paper
正在生成论文摘要