Feature Selection for High-Dimensional Neural Network Potentials with the Adaptive Group Lasso
arxiv(2023)
摘要
Neural network potentials are a powerful tool for atomistic simulations, allowing to accurately reproduce ab initio potential energy surfaces with computational performance approaching classical force fields. A central component of such potentials is the transformation of atomic positions into a set of atomic features in a most efficient and informative way.In this work, a feature selection method is introduced for high dimensional neural network potentials, based on the Adaptive Group Lasso (AGL) approach. It is shown that the use of an embedded method, taking into account the interplay between features and their action in the estimator, is necessary to optimize the number of features. The method's efficiency is tested on three different monoatomic systems, including Lennard-Jones as a simple test case, Aluminium as a system characterized by predominantly radial interactions, and Boron as representative of a system with strongly directional interactions. The AGL is compared with unsupervised filter methods and found to perform consistently better in reducing the number of features needed to reproduce the reference simulation data. In particular, our results show the importance of taking into account model predictions in feature selection for interatomic potentials.
更多查看译文
AI 理解论文
溯源树
样例
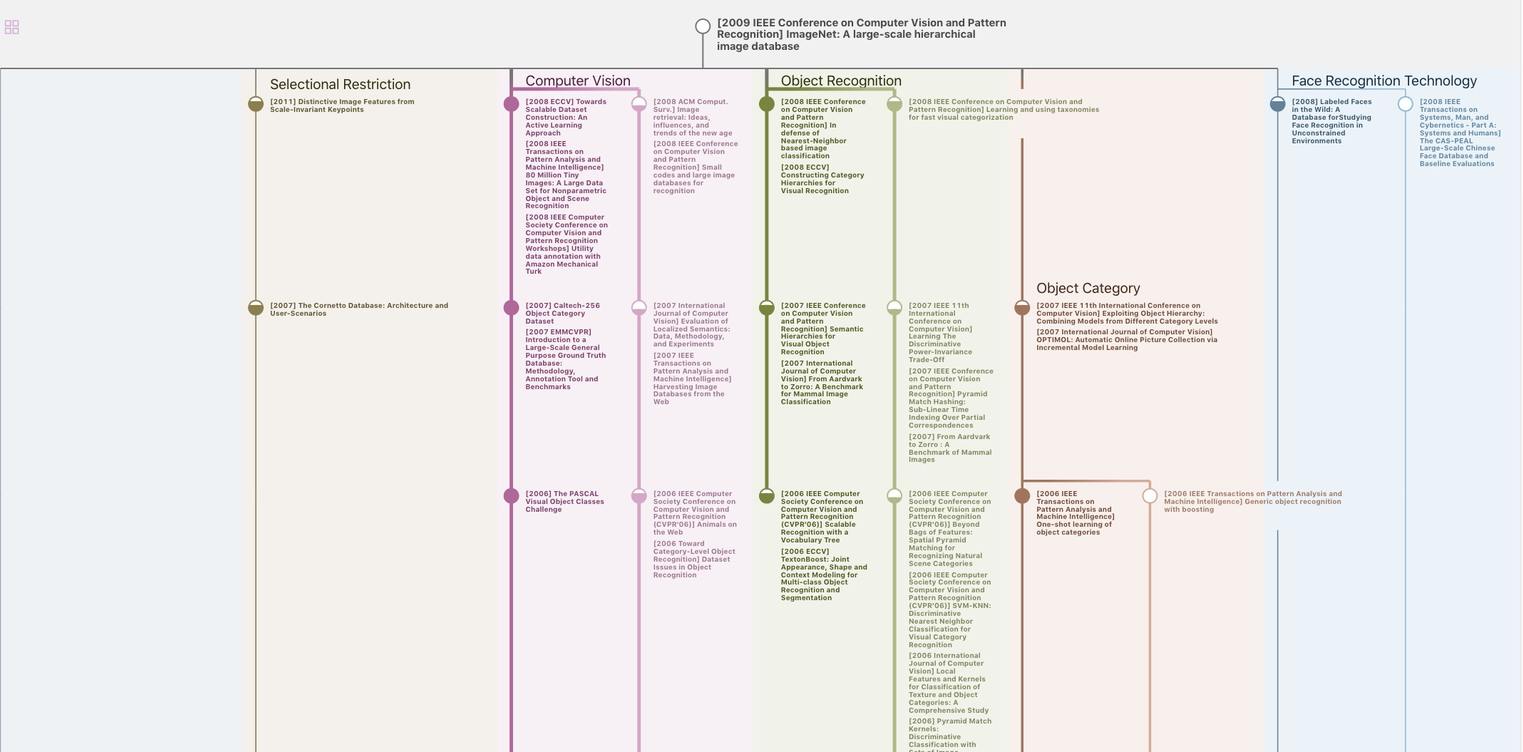
生成溯源树,研究论文发展脉络
Chat Paper
正在生成论文摘要