Extended Ranking Mechanisms for the m-Capacitated Facility Location Problem in Bayesian Mechanism Design
CoRR(2023)
摘要
In this paper, we initiate the study of the m-Capacitated Facility Location Problem (m-CFLP) within a Bayesian Mechanism Design framework. We consider the case in which every agent's private information is their position on a line and assume that every agent's position is independently drawn from a common and known distribution μ. In this context, we propose the Extended Ranking Mechanisms (ERMs), a truthful generalization of the recently introduced Ranking Mechanisms, that allows to handle problems where the total facility capacity exceeds the number of agents. Our primary results pertain to the study of the efficiency guarantees of the ERMs. In particular, we demonstrate that the limit of the ratio between the expected Social Cost of an ERM and the expected optimal Social Cost is finite. En route to these results, we reveal that the optimal Social Cost and the Social Cost of any ERMs can be expressed as the objective value of a suitable norm minimization problem in the Wasserstein space. We then tackle the problem of determining an optimal ERM tailored to a m-CFLP and a distribution μ. Specifically, we aim to identify an ERM whose limit Bayesian approximation ratio is the lowest compared to all other ERMs. We detail how to retrieve an optimal ERM in two frameworks: (i) when the total facility capacity matches the number of agents and (ii) when μ is the uniform distribution and we have two facilities to place. Lastly, we conduct extensive numerical experiments to compare the performance of the ERMs against other truthful mechanisms and to evaluate the convergence speed of the Bayesian approximation ratio. In summary, all our findings highlight that a well-tuned ERM consistently outperforms all other known mechanisms, making it a valid choice for solving the m-CFLP within a Bayesian framework.
更多查看译文
AI 理解论文
溯源树
样例
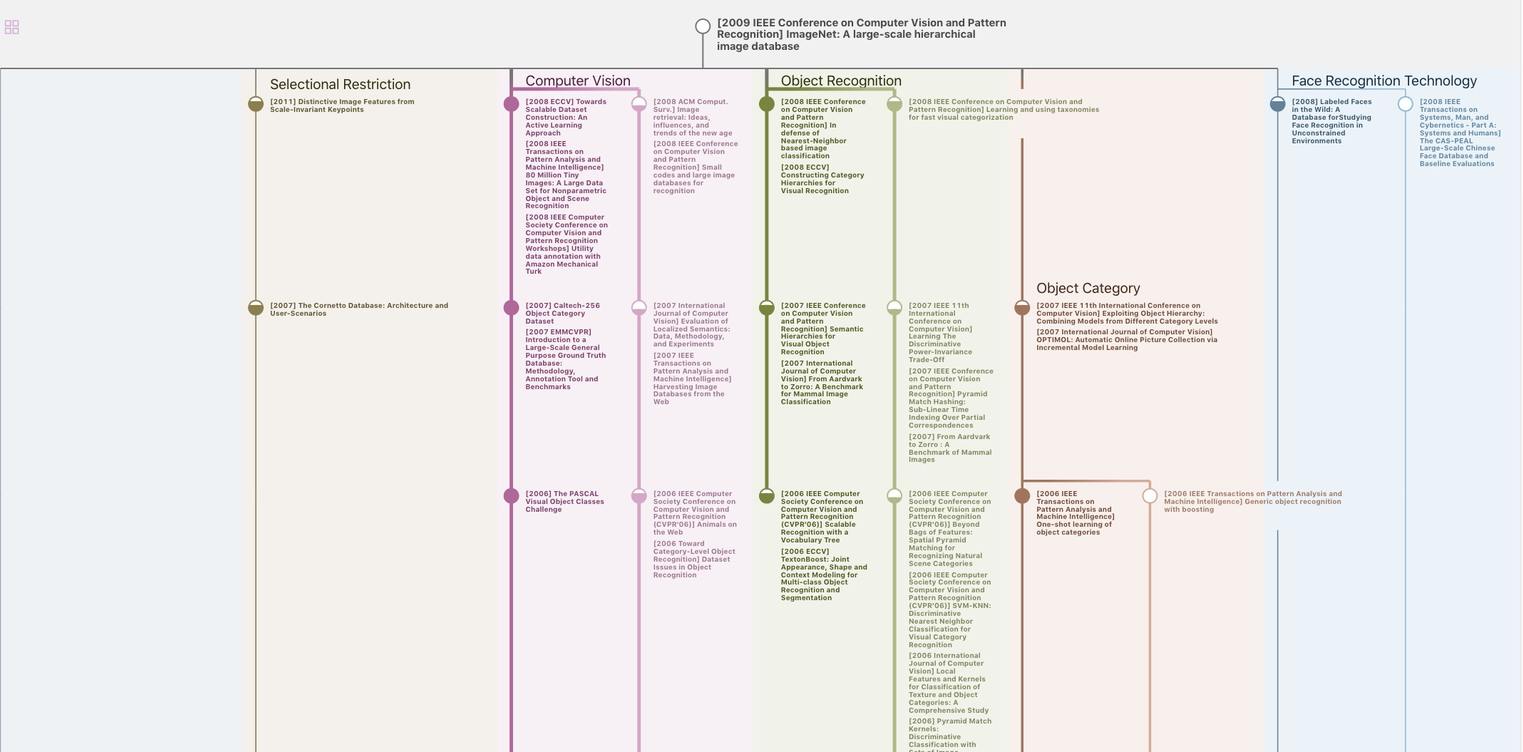
生成溯源树,研究论文发展脉络
Chat Paper
正在生成论文摘要