Rotation Equivariant Proximal Operator for Deep Unfolding Methods in Image Restoration.
IEEE transactions on pattern analysis and machine intelligence(2024)
摘要
The deep unfolding approach has attracted significant attention in computer vision tasks, which well connects conventional image processing modeling manners with more recent deep learning techniques. Specifically, by establishing a direct correspondence between algorithm operators at each implementation step and network modules within each layer, one can rationally construct an almost "white box" network architecture with high interpretability. In this architecture, only the predefined component of the proximal operator, known as a proximal network, needs manual configuration, enabling the network to automatically extract intrinsic image priors in a data-driven manner. In current deep unfolding methods, such a proximal network is generally designed as a CNN architecture, whose necessity has been proven by a recent theory. That is, CNN structure substantially delivers the translational symmetry image prior, which is the most universally possessed structural prior across various types of images. However, standard CNN-based proximal networks have essential limitations in capturing the rotation symmetry prior, another universal structural prior underlying general images. This leaves a large room for further performance improvement in deep unfolding approaches. To address this issue, this study makes efforts to suggest a high-accuracy rotation equivariant proximal network that effectively embeds rotation symmetry priors into the deep unfolding framework. Especially, we deduce, for the first time, the theoretical equivariant error for such a designed proximal network with arbitrary layers under arbitrary rotation degrees. This analysis should be the most refined theoretical conclusion for such error evaluation to date and is also indispensable for supporting the rationale behind such networks with intrinsic interpretability requirements. Through experimental validation on different vision tasks, including blind image super-resolution, medical image reconstruction, and image de-raining, the proposed method is validated to be capable of directly replacing the proximal network in current deep unfolding architecture and readily enhancing their state-of-the-art performance. This indicates its potential usability in general vision tasks. The code of our method is available at https://github.com/jiahong-fu/Equivariant-Proximal-Operator.
更多查看译文
关键词
Deep unfolding network,image deraining,image super-resolution,medical image reconstruction,proximal operator,rotation symmetry prior
AI 理解论文
溯源树
样例
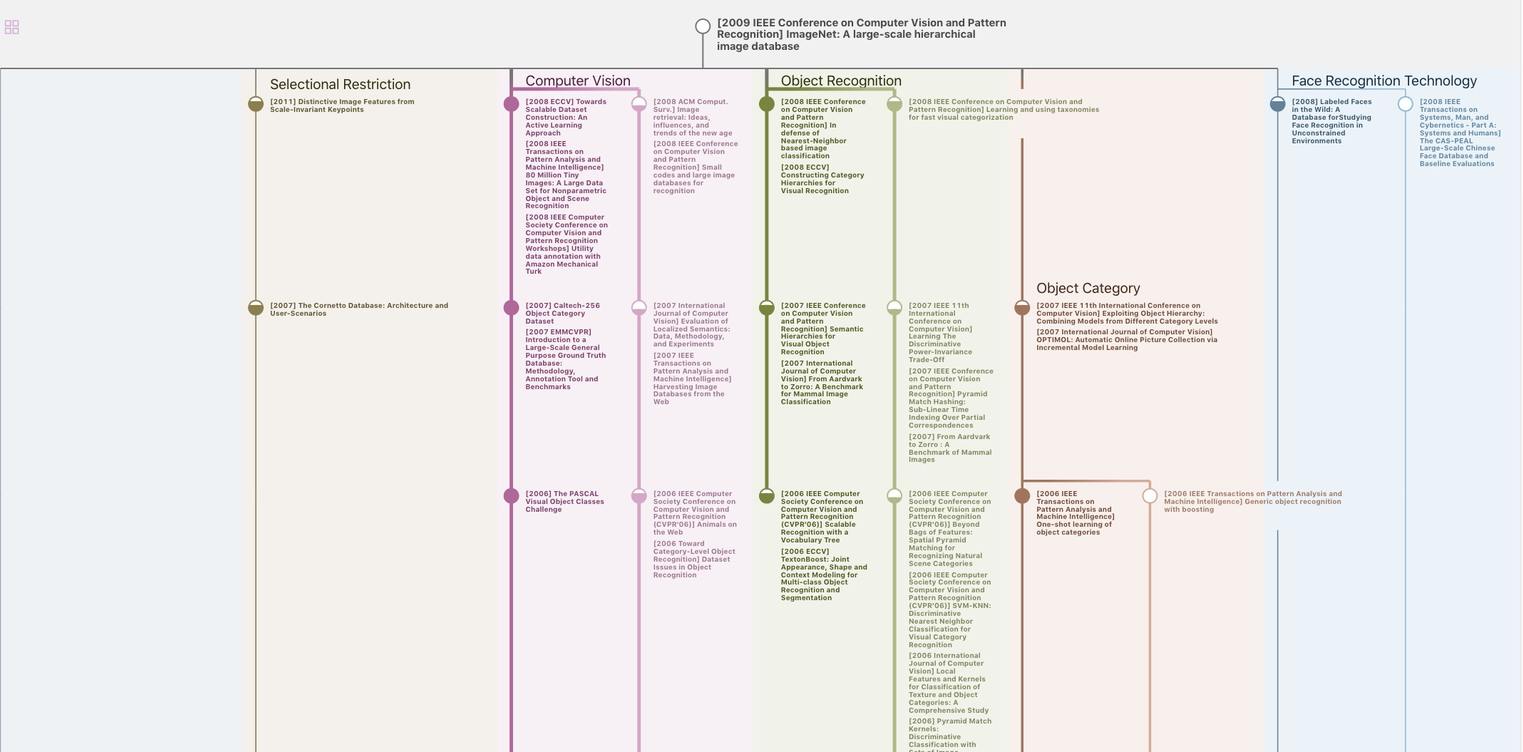
生成溯源树,研究论文发展脉络
Chat Paper
正在生成论文摘要