Graph Coarsening via Convolution Matching for Scalable Graph Neural Network Training
CoRR(2023)
摘要
Graph summarization as a preprocessing step is an effective and complementary technique for scalable graph neural network (GNN) training. In this work, we propose the Coarsening Via Convolution Matching (CONVMATCH) algorithm and a highly scalable variant, A-CONVMATCH, for creating summarized graphs that preserve the output of graph convolution. We evaluate CONVMATCH on six real-world link prediction and node classification graph datasets, and show it is efficient and preserves prediction performance while significantly reducing the graph size. Notably, CONVMATCH achieves up to 95 performance of GNNs on node classification while trained on graphs summarized down to 1 tasks, CONVMATCH consistently outperforms all baselines, achieving up to a 2x improvement.
更多查看译文
AI 理解论文
溯源树
样例
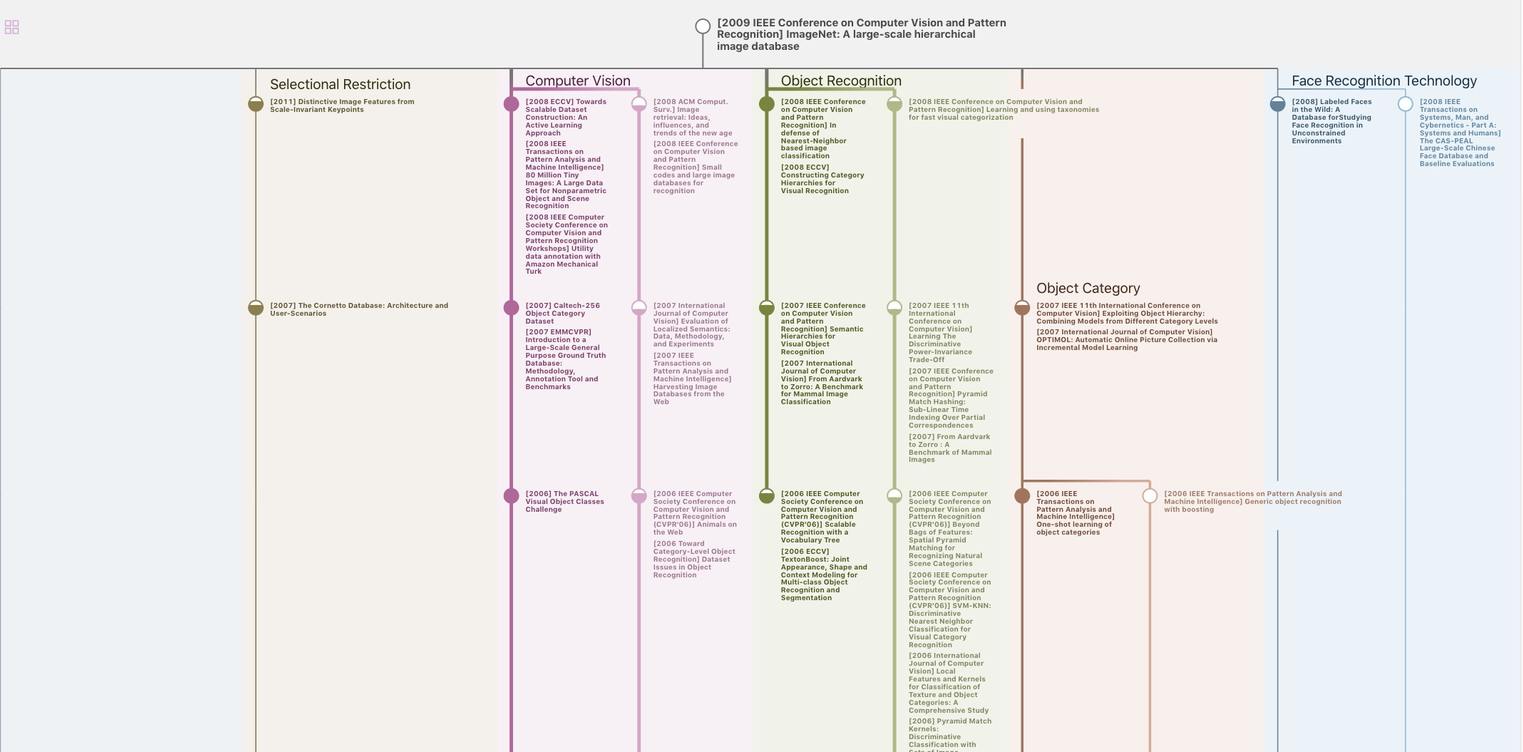
生成溯源树,研究论文发展脉络
Chat Paper
正在生成论文摘要