FoodLMM: A Versatile Food Assistant using Large Multi-modal Model
CoRR(2023)
摘要
Large Multi-modal Models (LMMs) have made impressive progress in many vision-language tasks. Nevertheless, the performance of general LMMs in specific domains is still far from satisfactory. This paper proposes FoodLMM, a versatile food assistant based on LMMs with various capabilities, including food recognition, ingredient recognition, recipe generation, nutrition estimation, food segmentation and multi-round conversation. To facilitate FoodLMM to deal with tasks beyond pure text output, we introduce a series of novel task-specific tokens and heads, enabling the model to predict food nutritional values and multiple segmentation masks. We adopt a two-stage training strategy. In the first stage, we utilize multiple public food benchmarks for multi-task learning by leveraging instruct-following paradigm. In the second stage, we construct a multi-round conversation and a reasoning segmentation datasets to fine-tune the model, enabling it to conduct professional dialogues and generate segmentation masks based on complex reasoning in food domain. Our fine-tuned FoodLMM achieves state-of-the-art results across several food benchmarks. We will make our code, models and datasets publicly available.
更多查看译文
AI 理解论文
溯源树
样例
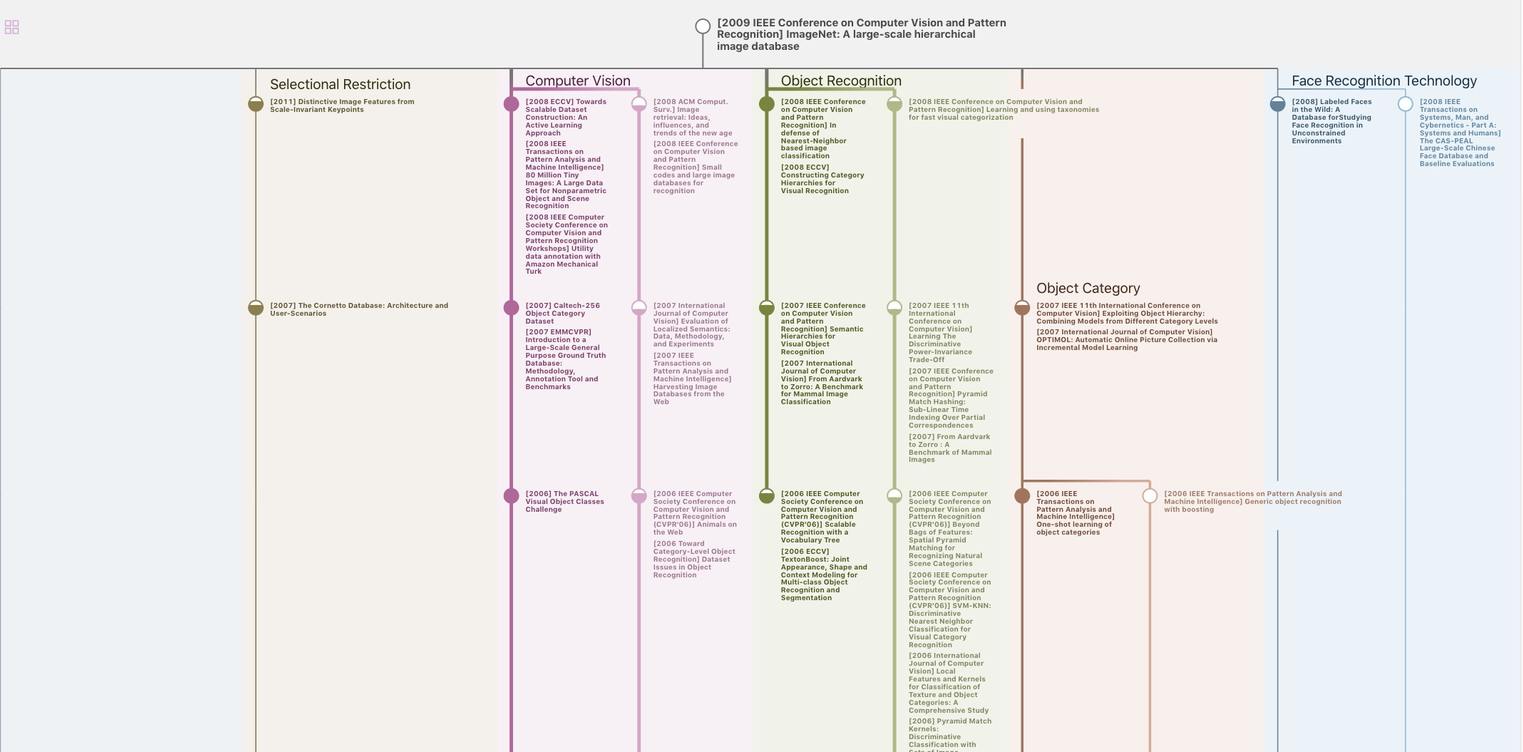
生成溯源树,研究论文发展脉络
Chat Paper
正在生成论文摘要