DPA-2: Towards a universal large atomic model for molecular and material simulation
arxiv(2023)
摘要
The rapid development of artificial intelligence (AI) is driving significant changes in the field of atomic modeling, simulation, and design. AI-based potential energy models have been successfully used to perform large-scale and long-time simulations with the accuracy of ab initio electronic structure methods. However, the model generation process still hinders applications at scale. We envision that the next stage would be a model-centric ecosystem, in which a large atomic model (LAM), pre-trained with as many atomic datasets as possible and can be efficiently fine-tuned and distilled to downstream tasks, would serve the new infrastructure of the field of molecular modeling. We propose DPA-2, a novel architecture for a LAM, and develop a comprehensive pipeline for model fine-tuning, distillation, and application, associated with automatic workflows. We show that DPA-2 can accurately represent a diverse range of chemical systems and materials, enabling high-quality simulations and predictions with significantly reduced efforts compared to traditional methods. Our approach paves the way for a universal large atomic model that can be widely applied in molecular and material simulation research, opening new opportunities for scientific discoveries and industrial applications.
更多查看译文
AI 理解论文
溯源树
样例
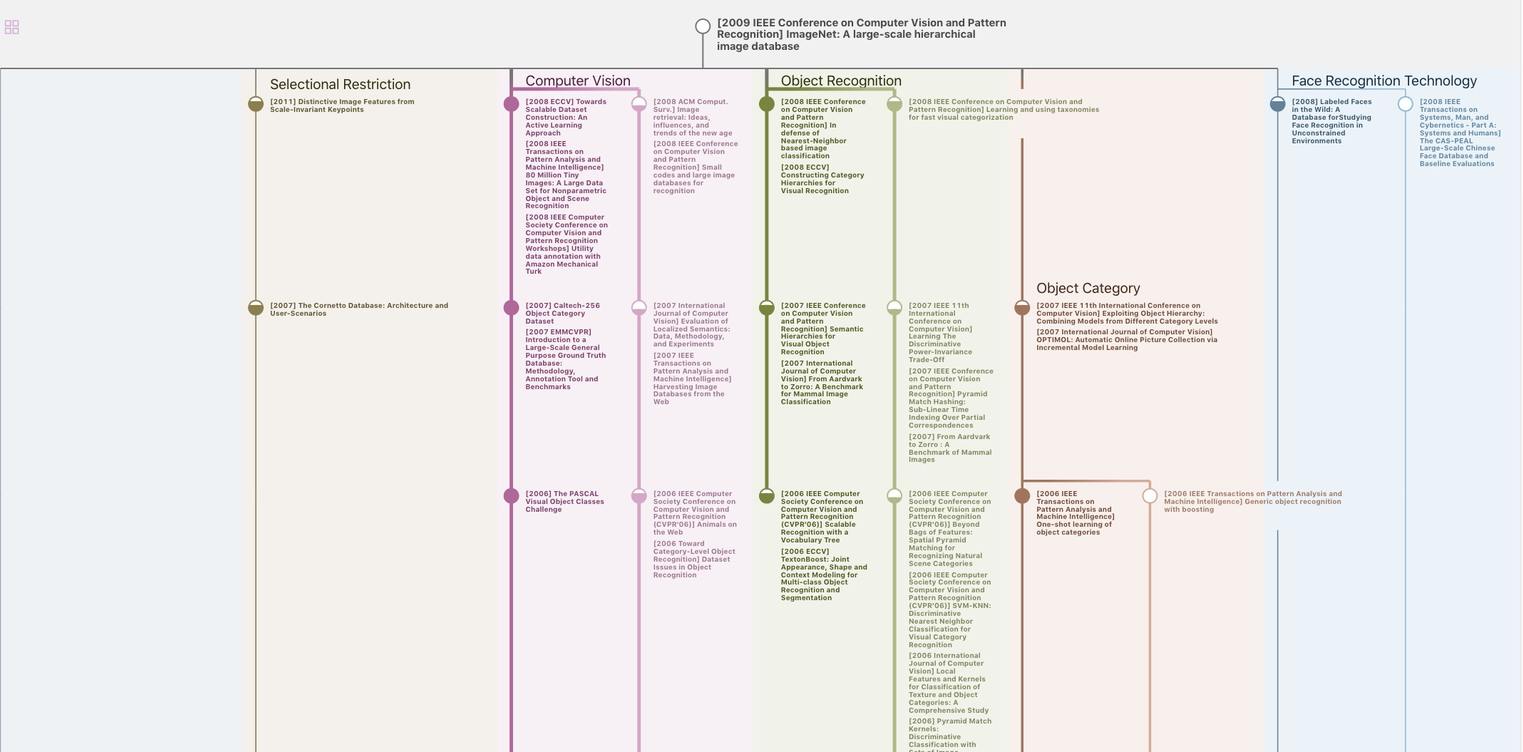
生成溯源树,研究论文发展脉络
Chat Paper
正在生成论文摘要