A Variational Autoencoder for Neural Temporal Point Processes with Dynamic Latent Graphs
AAAI 2024(2024)
摘要
Continuously observed event occurrences, often exhibit self and mutually exciting effects, which can be well modeled using temporal point processes. Beyond that, these event dynamics may also change over time, with certain periodic trends. We propose a novel variational autoencoder to capture such a mixture of temporal dynamics. More specifically, the whole time interval of the input sequence is partitioned into a set of sub intervals. The event dynamics are assumed to be stationary within each subinterval, but could be changing across those subintervals. In particular, we use a sequential latent variable model to learn a dependency graph between the observed dimensions, for each subinterval. The model predicts the future event times, by using the learned dependency graph to remove the non contributing influences of past events. By doing so, the proposed model demonstrates its higher accuracy in predicting inter event times and event types for several real world event sequences, compared with existing state of the art neural point processes.
更多查看译文
关键词
ML: Time-Series/Data Streams,DMKM: Data Stream Mining
AI 理解论文
溯源树
样例
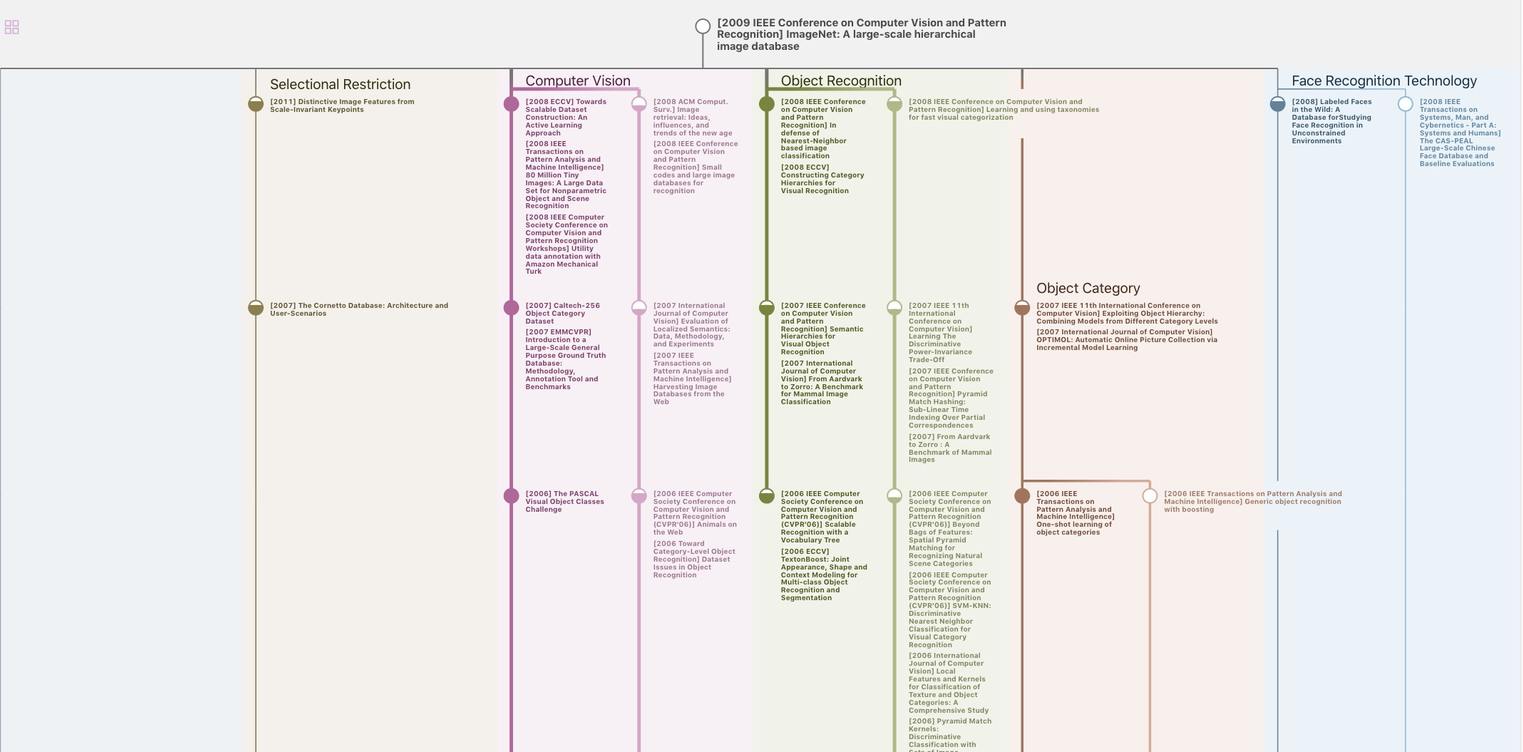
生成溯源树,研究论文发展脉络
Chat Paper
正在生成论文摘要