Best-of-Both-Worlds Algorithms for Linear Contextual Bandits
CoRR(2023)
摘要
We study best-of-both-worlds algorithms for K-armed linear contextual bandits. Our algorithms deliver near-optimal regret bounds in both the adversarial and stochastic regimes, without prior knowledge about the environment. In the stochastic regime, we achieve the polylogarithmic rate (dK)^2polylog(dKT)/Δ_min, where Δ_min is the minimum suboptimality gap over the d-dimensional context space. In the adversarial regime, we obtain either the first-order O(dK√(L^*)) bound, or the second-order O(dK√(Λ^*)) bound, where L^* is the cumulative loss of the best action and Λ^* is a notion of the cumulative second moment for the losses incurred by the algorithm. Moreover, we develop an algorithm based on FTRL with Shannon entropy regularizer that does not require the knowledge of the inverse of the covariance matrix, and achieves a polylogarithmic regret in the stochastic regime while obtaining O(dK√(T)) regret bounds in the adversarial regime.
更多查看译文
AI 理解论文
溯源树
样例
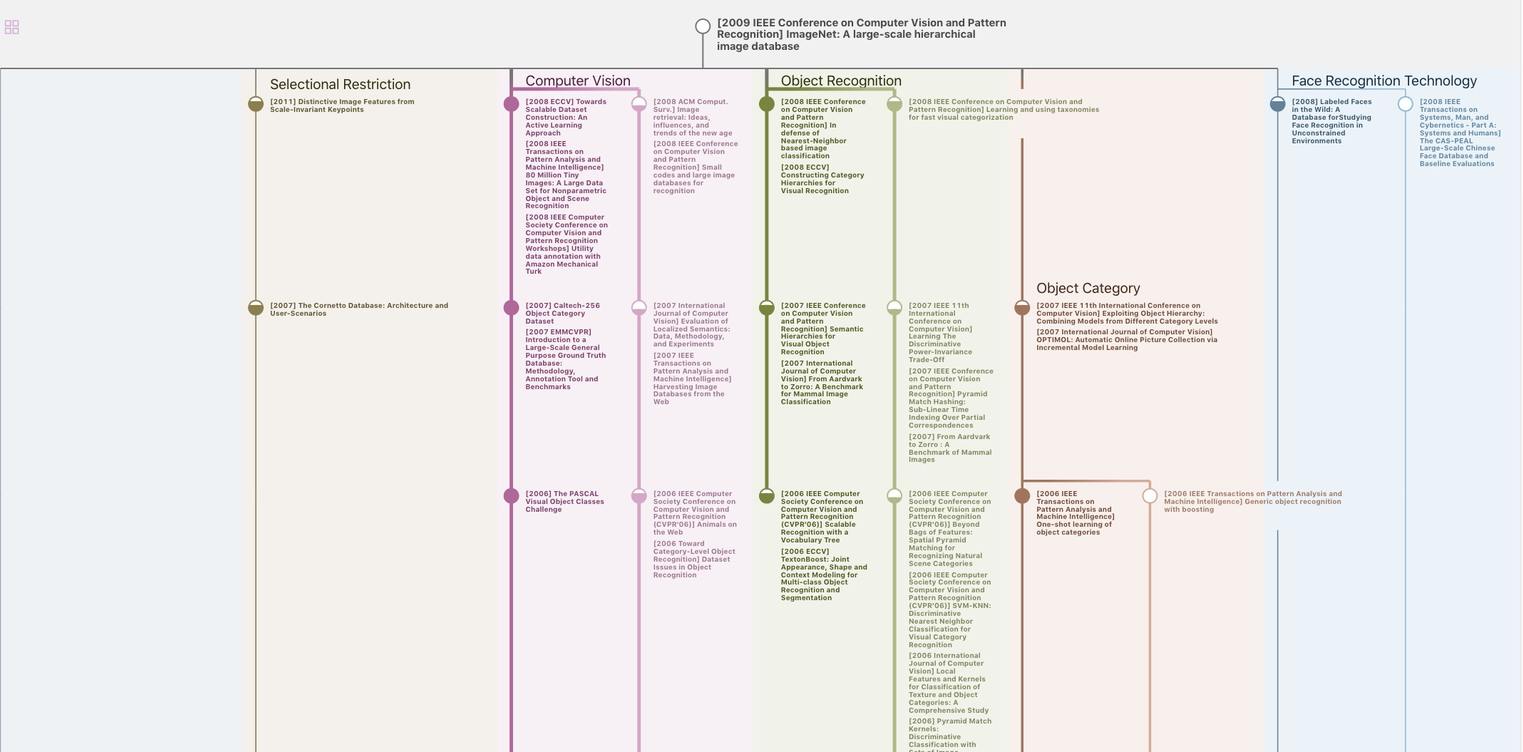
生成溯源树,研究论文发展脉络
Chat Paper
正在生成论文摘要