SED: Searching Enhanced Decoder with switchable skip connection for semantic segmentation
PATTERN RECOGNITION(2024)
摘要
Neural architecture search (NAS) has shown excellent performance. However, existing semantic segmentation models rely heavily on pre-training on Image-Net or COCO and mainly focus on the designing of decoders. Directly training the encoder-decoder architecture search models from scratch to SOTA for semantic segmentation requires even thousands GPU days, which greatly limits the application of NAS. To address this issue, we propose a novel neural architecture Search framework for Enhanced Decoder (SED). Utilizing the pre-trained hand-designing backbone and the searching space composed of light-weight cells, SED searches for a decoder which can perform high-quality segmentation. Furthermore, we attach switchable skip connection operations to search space, expanding the diversity of possible network structure. The parameters of backbone and operations selected in searching phrase are copied to retraining process. As a result, searching, pruning and retraining can be done in just 1 day. The experimental results show that the SED proposed in this paper only needs 1/4 of the parameters and calculation in contrast to hand-designing decoder, and obtains higher segmentation accuracy on Cityscapes. Transferring the same decoder architecture to other datasets, such as: Pascal VOC 2012, Camvid, ADE20K proves the robustness of SED.
更多查看译文
关键词
NAS,Semantic segmentation,Encoder-decoder model
AI 理解论文
溯源树
样例
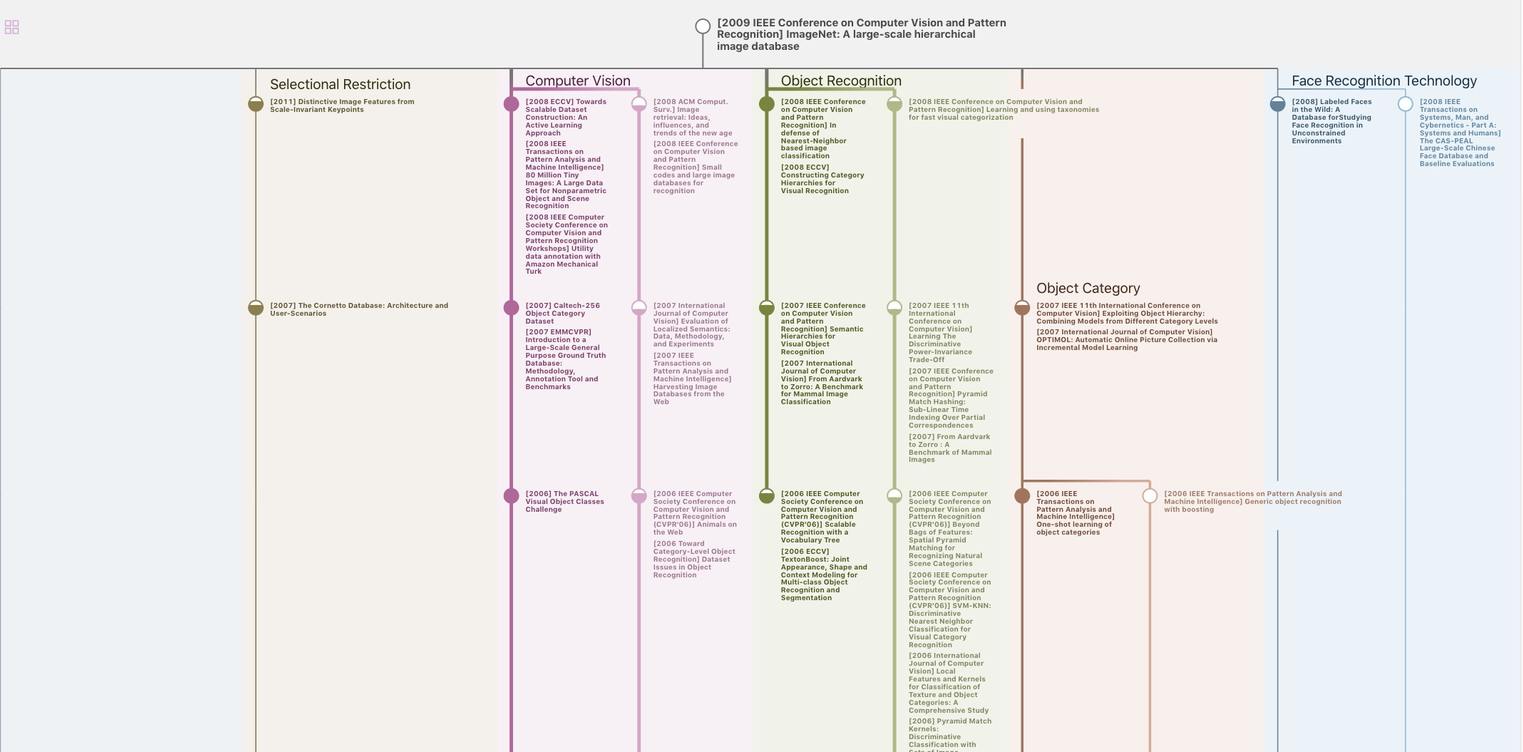
生成溯源树,研究论文发展脉络
Chat Paper
正在生成论文摘要