PDATC-NCPMKL: Predicting drug's Anatomical Therapeutic Chemical (ATC) codes based on network consistency projection and multiple kernel learning
COMPUTERS IN BIOLOGY AND MEDICINE(2024)
摘要
The development and discovery of new drugs is time-consuming and needs lots of human and material resources. Therefore, discovery of novel effects of existing drugs is an important alternative way, which can accelerate the process of designing "new" drugs. The anatomical Therapeutic Chemical (ATC) classification system recommended by World Health Organization (WHO) is a basic research area in this regard. A novel ATC code of an existing drug suggests its novel effects. Some computational models have been proposed, which can predict the drug-ATC code associations. However, their performance is not very high. There still exist spaces for improve-ment. In this study, a new recommendation system (named PDATC-NCPMKL), which incorporated network consistency projection and multi-kernel learning, was designed to identify drug-ATC code associations. For drugs or ATC codes, several kernels were constructed, which were fused by a multiple kernel learning method and an additional kernel integration scheme. To enhance the performance, the drug-ATC code association adjacency matrix was reformulated by a variant of weighted K nearest known neighbors (WKNKN). The reformulated adjacency matrix, drug and ATC code kernels were fed into network consistency projection to generate the as-sociation score matrix. The proposed recommendation system was tested on the ATC codes at the second, third and fourth levels in drug ATC classification system using ten-fold cross-validation. The results indicated that all AUROC and AUPR values were close to or exceeded 0.96. Such performance was higher than some existing computational models. Some additional tests were conducted to prove the utility of adjacency matrix reformulation and to analyze the importance of drug and ATC code kernels.
更多查看译文
关键词
Anatomical therapeutic chemical,Drug repositioning,Network consistency projection,Multiple kernel learning
AI 理解论文
溯源树
样例
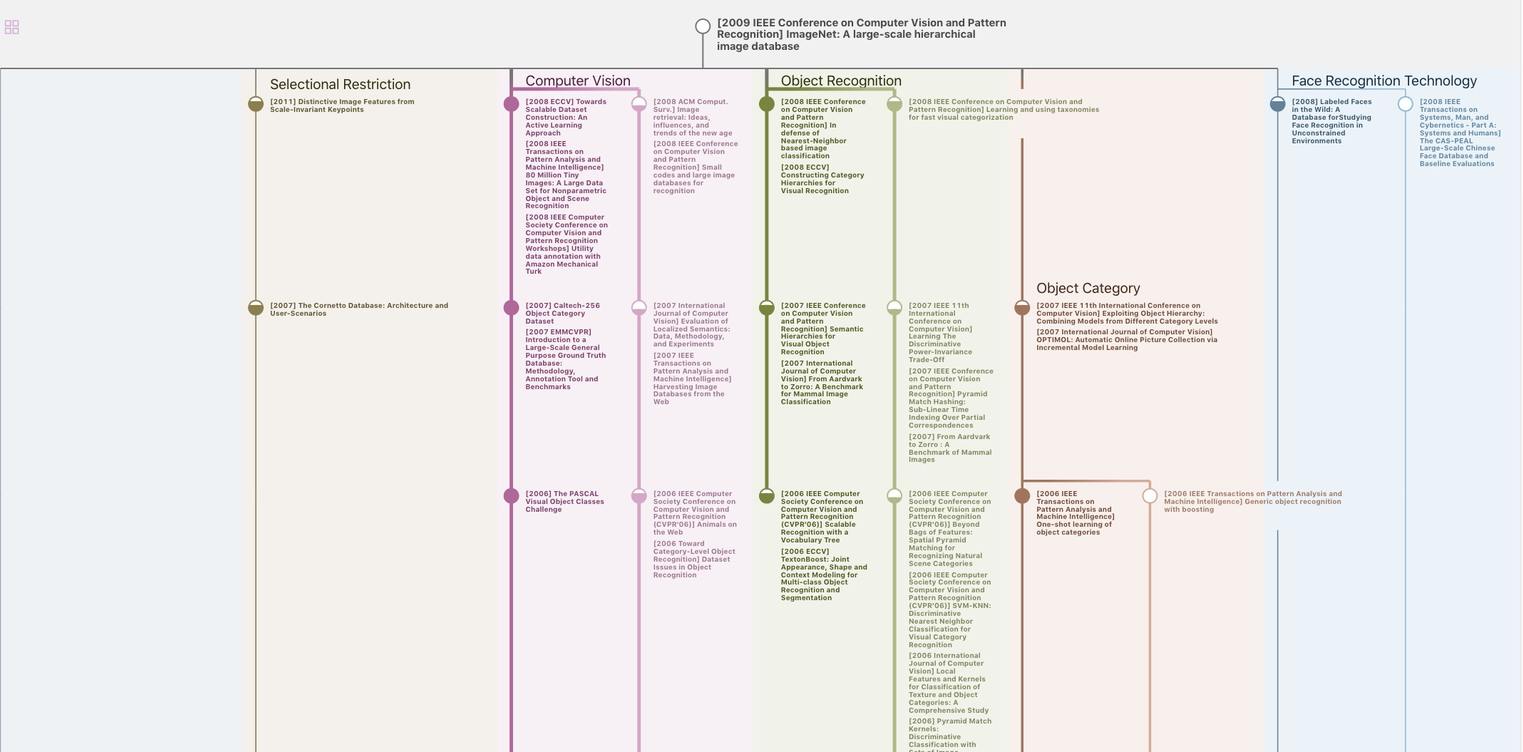
生成溯源树,研究论文发展脉络
Chat Paper
正在生成论文摘要