Enhancing Convolutional Neural Network Architectures with Long Short-Term Memory for Improved Image Classification
2023 8th International Scientific Conference on Applying New Technology in Green Buildings (ATiGB)(2023)
摘要
In recent years, the application of artificial intelligence and deep learning has expanded to address a variety of tasks, including image classification. Researchers have de-veloped more complex network architectures with more pa-rameters to improve the convergence performance of neural networks. However, this has also increased the complexity of problem-solving and hardware requirements. To address this issue, we propose integrating Long Short-Term Memory (LSTM) into known Convolutional Neural Network (CNN) architectures to enhance their performance without significantly increasing complexity. Our experiments with several well-known neural network architectures, including some network architectures such as ResNet, DenseNet, and MobileNet, evaluated on different datasets such as 525 bird species, CIFAR10, and GTSRB for classification, demonstrate that the integration of LSTM into known CNN architectures can improve their performance in image classification tasks.
更多查看译文
关键词
Long Short-Term Memory,Image Classification,Neural Networks,Deep Learning
AI 理解论文
溯源树
样例
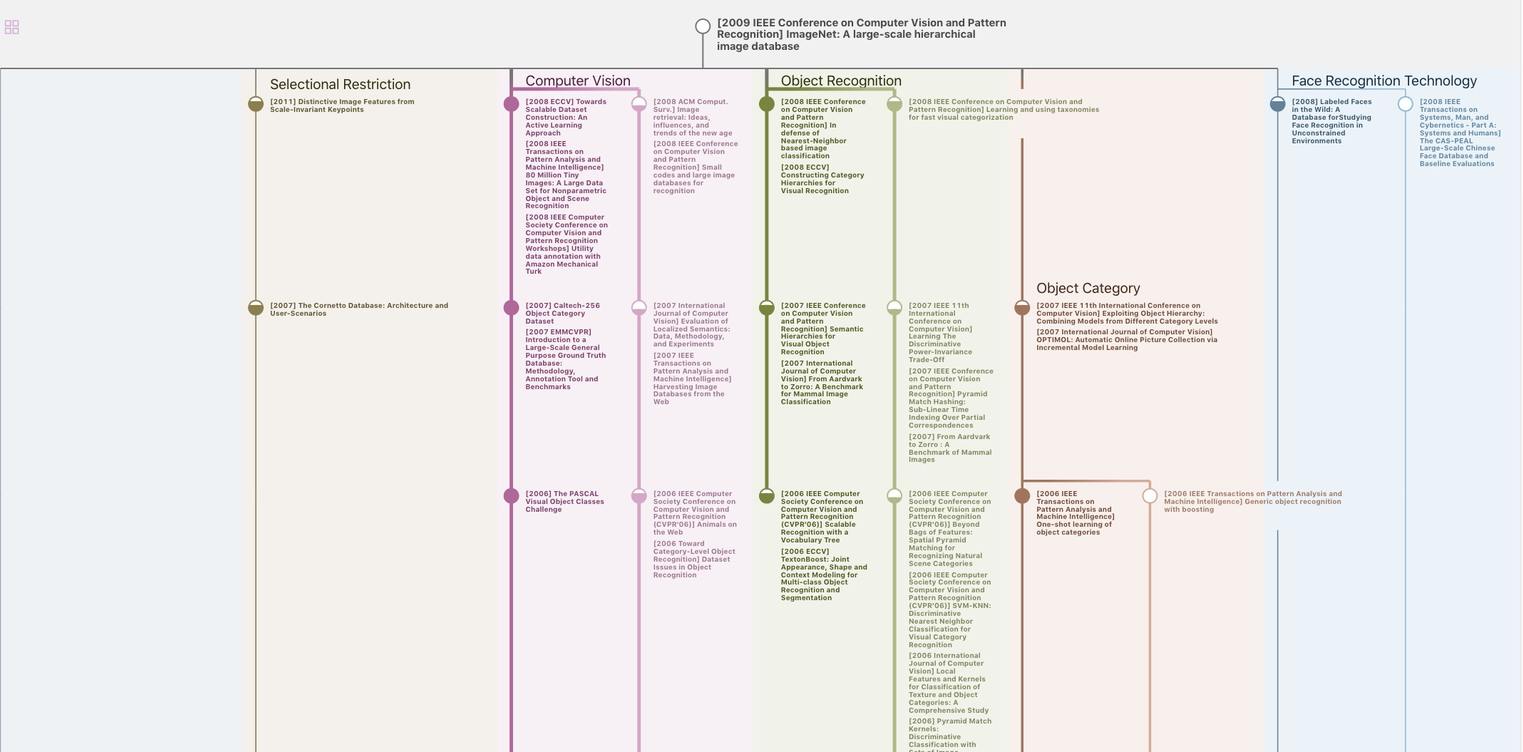
生成溯源树,研究论文发展脉络
Chat Paper
正在生成论文摘要