MCRFF: A Meta-Contrastive Learning-Based RF Fingerprinting Method
MILCOM 2023 - 2023 IEEE MILITARY COMMUNICATIONS CONFERENCE(2023)
摘要
Radio frequency fingerprinting (RFF) is used for wireless device identification based on the hardware impairments of the transmitter. Recent works extensively adopt deep learning models to perform RFF, which train the model with data collected from some devices (seen devices), and the trained model can then perform classification. The performance of these methods needs to be improved. More importantly, new unseen devices may often be added to the network. Unfortunately, the performance of existing methods for detecting and classifying unseen devices is often unsatisfactory. In this paper, we propose a meta-contrastive learning-based RFF method (MCRFF). As a model-agnostic training procedure, MCRFF can be applied to almost any RFF model, which can improve the performance of the model for seen devices while especially improving the performance of the model for unseen devices. Inspired by recent advances in contrastive learning and meta-learning, MCRFF enables models to learn to extract similarity-based discriminative representations and to coordinately improve the model's performance for both seen and unseen devices. We conduct extensive experiments on LoRa and WiFi devices to demonstrate the effectiveness of MCRFF. Moreover, we explore replacing existing models with a lightweight model that has an order of magnitude less computational cost, yet achieves better performance with the help of MCRFF.
更多查看译文
关键词
RF fingerprinting,contrastive learning,metalearning,Internet of things
AI 理解论文
溯源树
样例
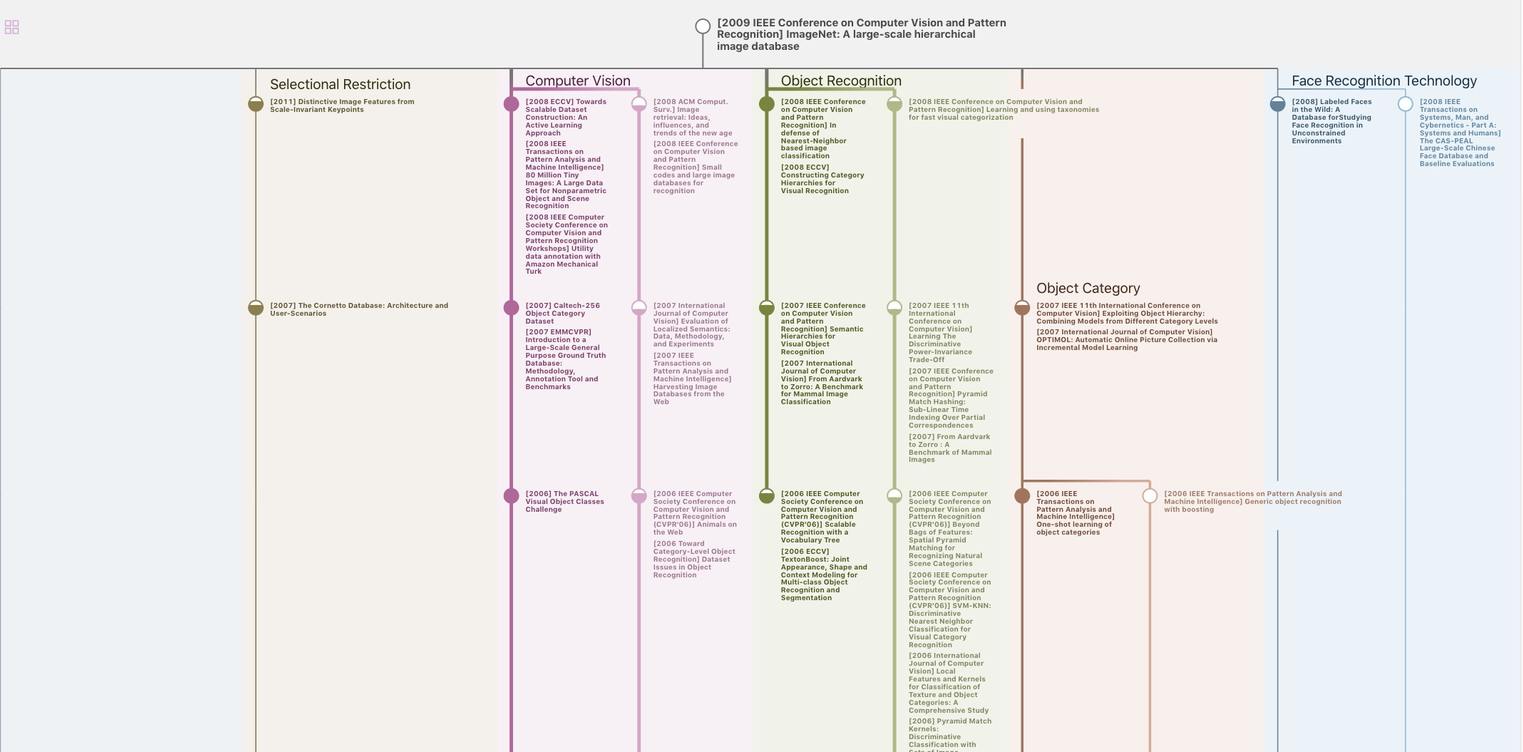
生成溯源树,研究论文发展脉络
Chat Paper
正在生成论文摘要