End-to-End O-RAN Control-Loop For Radio Resource Allocation in SDR-Based 5G Network
MILCOM 2023 - 2023 IEEE MILITARY COMMUNICATIONS CONFERENCE(2023)
摘要
End-to-end Open Radio Access Network (ORAN) demos, including Software-defined Radios (SDRs), can identify key gaps in the early stages of research and accelerate time-to-market and early adoption for next-generation wireless technologies. This work showcases an innovative end-to-end 5G-based ORAN deployment that leverages open-source tools and an Artificial Intelligence (AI)/Machine Learning (ML) framework. The deployment utilizes Software Radio Systems RAN (srsRAN) Central Unit (CU)-Distributed Unit (DU) connected to the Open5GS core network operating in 5G standalone (SA) mode. It also demonstrates the integration of Non-Real Time RIC (Non-RT RIC) and Near-Real Time RIC (Near-RT RIC) with embedded intelligence to perform radio resource allocation. An AI/ML framework deploys an optimized ML model as an rApp that complements a resource allocation xApp.
更多查看译文
关键词
ORAN,srsRAN,Non-RT RIC,Near-RT RIC,AI/ML framework,5G NR SA
AI 理解论文
溯源树
样例
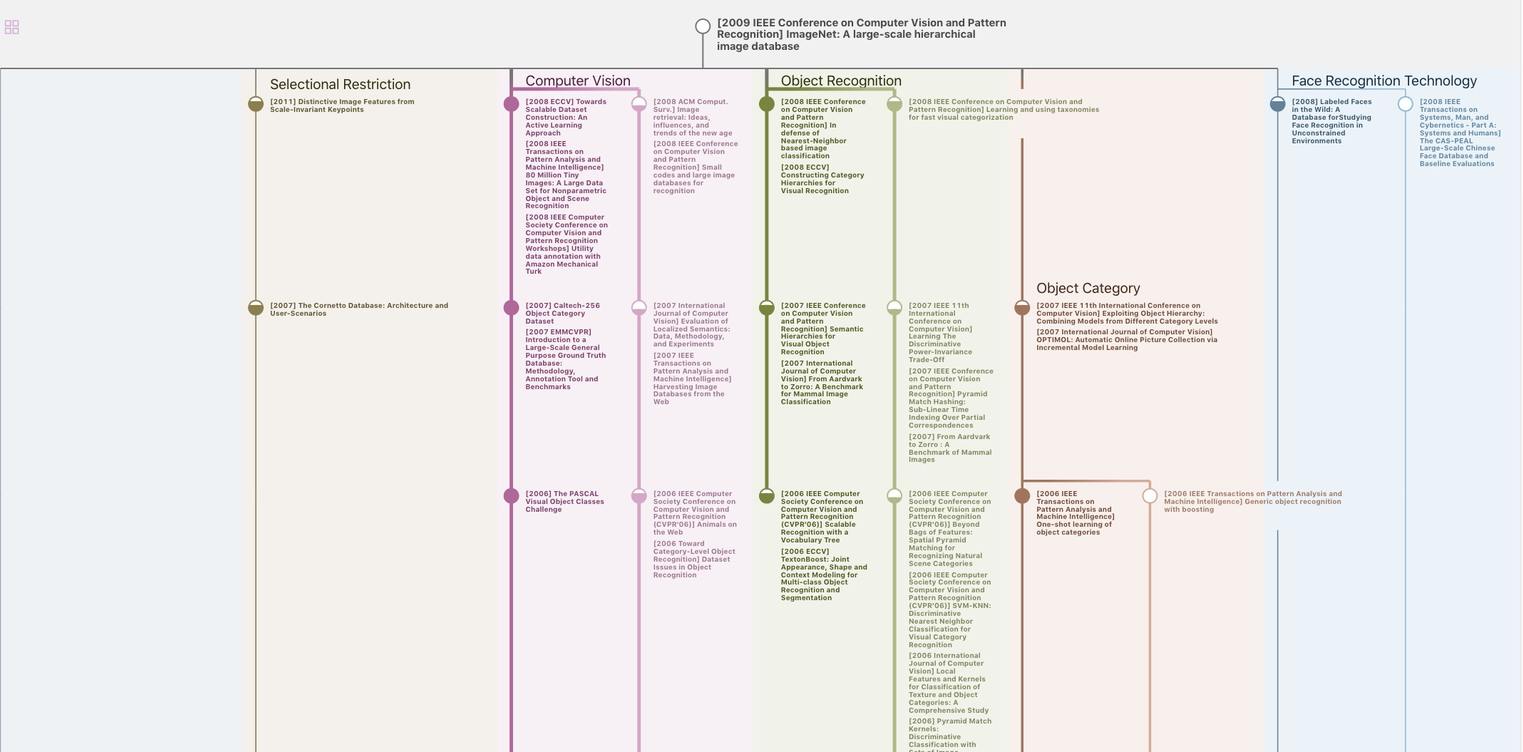
生成溯源树,研究论文发展脉络
Chat Paper
正在生成论文摘要